Finite sample performance of a long run variance estimator based on exactly (almost) unbiased autocovariance estimators
Economics Letters(2018)
Abstract
This paper proposes a bias reduced long run variance (LRV) estimator of a univariate time series with unknown mean that addresses well known finite sample bias problems. The LRV estimator is based on the (almost) exactly unbiased autocovariance estimator proposed by Vogelsang and Yang (2016). Whereas using fixed-b critical values is known to correct downward bias in LRV estimates generated by demeaning the data, the approach we take also corrects the classic Parzen bias that is not captured by the fixed-b approach. When applied to the tests of the null hypothesis of the mean in a simple location model, a simulation study shows that the proposed LRV estimator leads to tests with less over-rejections while maintaining power at least as high and often higher as the standard robust t test based on fixed-b critical values. These simulations suggest further theoretical analysis of the bias reduction approach is warranted.
MoreTranslated text
Key words
C12,C13,C14,C22
AI Read Science
Must-Reading Tree
Example
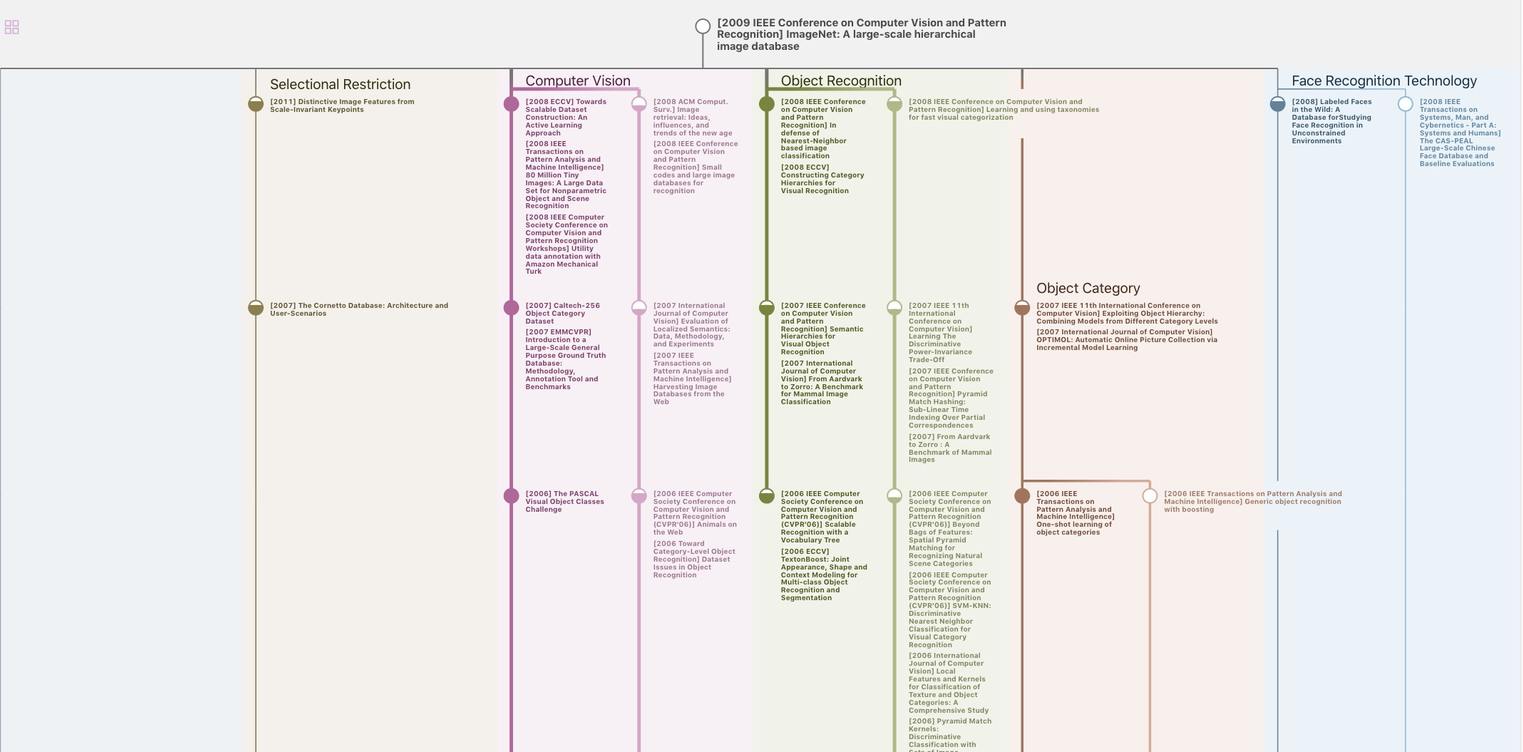
Generate MRT to find the research sequence of this paper
Chat Paper
Summary is being generated by the instructions you defined