Local Anomaly Detection by Application of Regression Analysis on PMU Data
2018 IEEE POWER & ENERGY SOCIETY GENERAL MEETING (PESGM)(2018)
Abstract
PMU data has the potential of providing a wealth of information on power system operation, health, faults and anomalies. PMU tend to provide tens of measurements per second, therefore automated anomaly detection is required; especially for use in real or near-time applications by power system operators. This paper demonstrates a method of detecting local anomalies in PMU data utilizing multiple linear regression. A window of near-time data is employed to generate a regression function that predicts the live data that arrives. If the error between the observed and predicted values exceeds a threshold an exception is noted. The threshold is dynamically updated based on the error in the regression function, allowing the method to work equally well on data of varying regularity. This anomaly detection method is not tuned to particular events and should detect novel occurrences. The method is evaluated on two numerical case studies, the real data was collected from PMUs placed on the Irish power system.
MoreTranslated text
Key words
Anomaly detection, PMU, machine learning, linear regression
AI Read Science
Must-Reading Tree
Example
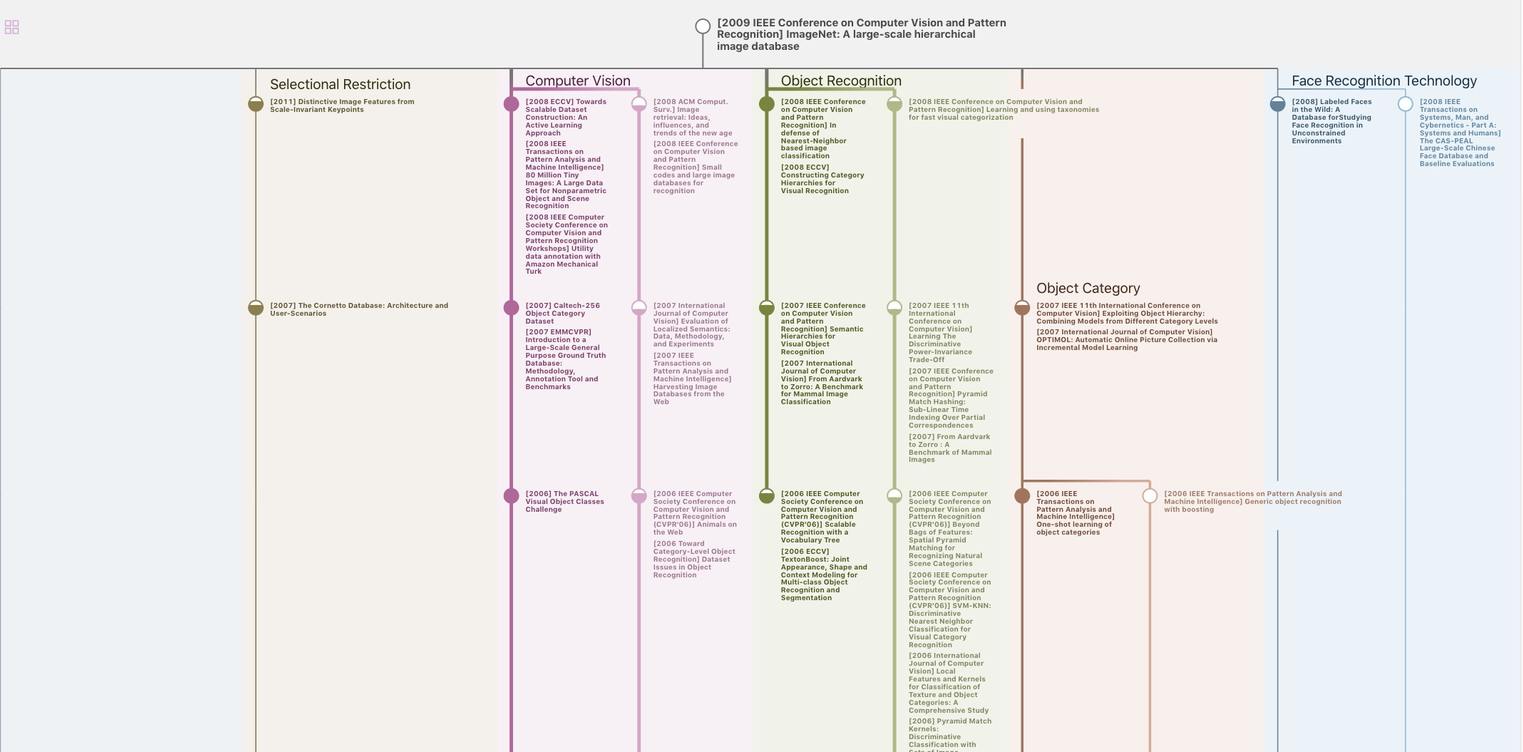
Generate MRT to find the research sequence of this paper
Chat Paper
Summary is being generated by the instructions you defined