Simultaneous selection of multiple important single nucleotide polymorphisms in familial genome wide association studies data
arXiv: Applications(2023)
摘要
We propose a resampling-based fast variable selection technique for detecting relevant single nucleotide polymorphisms (SNP) in a multi-marker mixed effect model. Due to computational complexity, current practice primarily involves testing the effect of one SNP at a time, commonly termed as ‘single SNP association analysis’. Joint modeling of genetic variants within a gene or pathway may have better power to detect associated genetic variants, especially the ones with weak effects. In this paper, we propose a computationally efficient model selection approach—based on the e-values framework—for single SNP detection in families while utilizing information on multiple SNPs simultaneously. To overcome computational bottleneck of traditional model selection methods, our method trains one single model, and utilizes a fast and scalable bootstrap procedure. We illustrate through numerical studies that our proposed method is more effective in detecting SNPs associated with a trait than either single-marker analysis using family data or model selection methods that ignore the familial dependency structure. Further, we perform gene-level analysis in Minnesota Center for Twin and Family Research (MCTFR) dataset using our method to detect several SNPs using this that have been implicated to be associated with alcohol consumption.
更多查看译文
关键词
genome,association,simultaneous selection
AI 理解论文
溯源树
样例
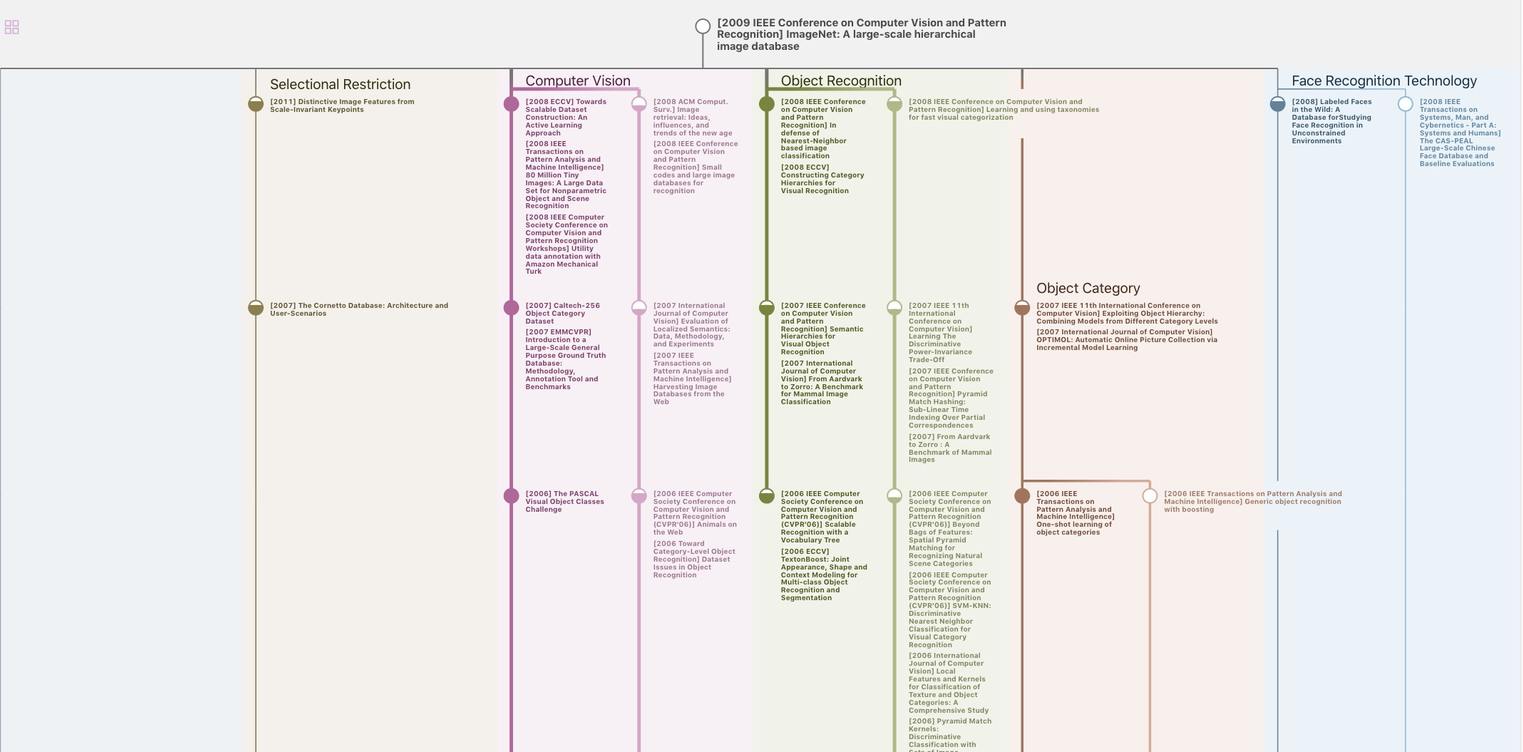
生成溯源树,研究论文发展脉络
Chat Paper
正在生成论文摘要