Parallel LDA with Over-Decomposition
2017 IEEE 24th International Conference on High Performance Computing Workshops (HiPCW)(2017)
摘要
Latent Dirichlet Allocation (LDA) is a statistical technique for topic modeling. Prior efforts to parallelize LDA have either used expensive atomic operations or weakened the sampling model to enable parallelization without heavy use of atomics. In this paper, we present a parallel LDA implementation that uses an over-decomposed 2D tiling strategy to overcome the limitations of previous parallelization schemes. An alternate implementation that uses some approximation is also presented that fully avoids use of atomic operations. Experimental results with the NIPS and NYTimes datasets demonstrate the effectiveness of the new implementations.
更多查看译文
关键词
Parallel Topic Modeling,Parallel Latent Dirichlet Allocation,Parallel Machine Learning
AI 理解论文
溯源树
样例
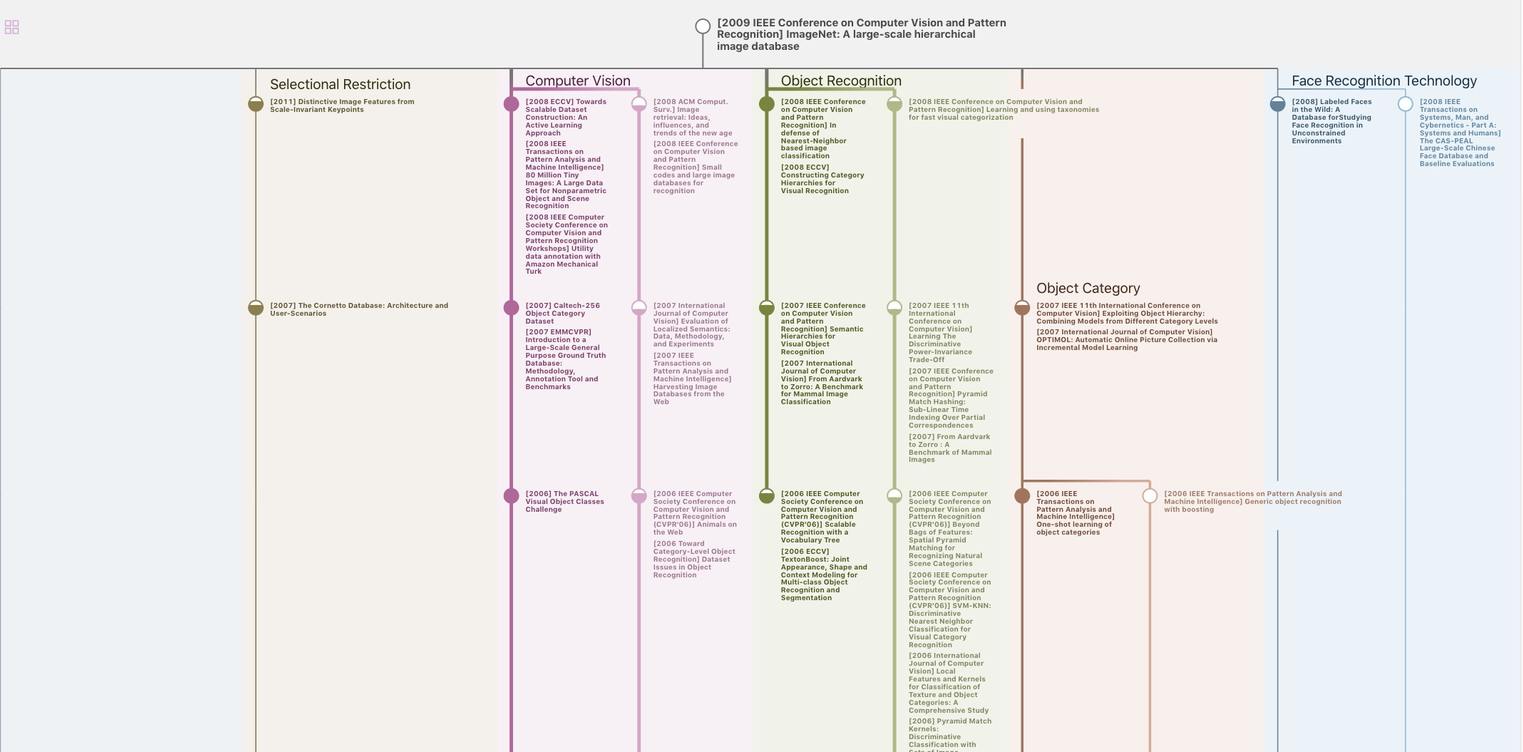
生成溯源树,研究论文发展脉络
Chat Paper
正在生成论文摘要