Robust clustering for functional data based on trimming and constraints
Advanced Data Analysis and Classification(2018)
Abstract
Many clustering algorithms when the data are curves or functions have been recently proposed. However, the presence of contamination in the sample of curves can influence the performance of most of them. In this work we propose a robust, model-based clustering method that relies on an approximation to the “density function” for functional data. The robustness follows from the joint application of data-driven trimming, for reducing the effect of contaminated observations, and constraints on the variances, for avoiding spurious clusters in the solution. The algorithm is designed to perform clustering and outlier detection simultaneously by maximizing a trimmed “pseudo” likelihood. The proposed method has been evaluated and compared with other existing methods through a simulation study. Better performance for the proposed methodology is shown when a fraction of contaminating curves is added to a non-contaminated sample. Finally, an application to a real data set that has been previously considered in the literature is given.
MoreTranslated text
Key words
Functional data analysis, Clustering, Robustness, Trimming, Functional principal components analysis, 62G35, 62H30, 68T10
AI Read Science
Must-Reading Tree
Example
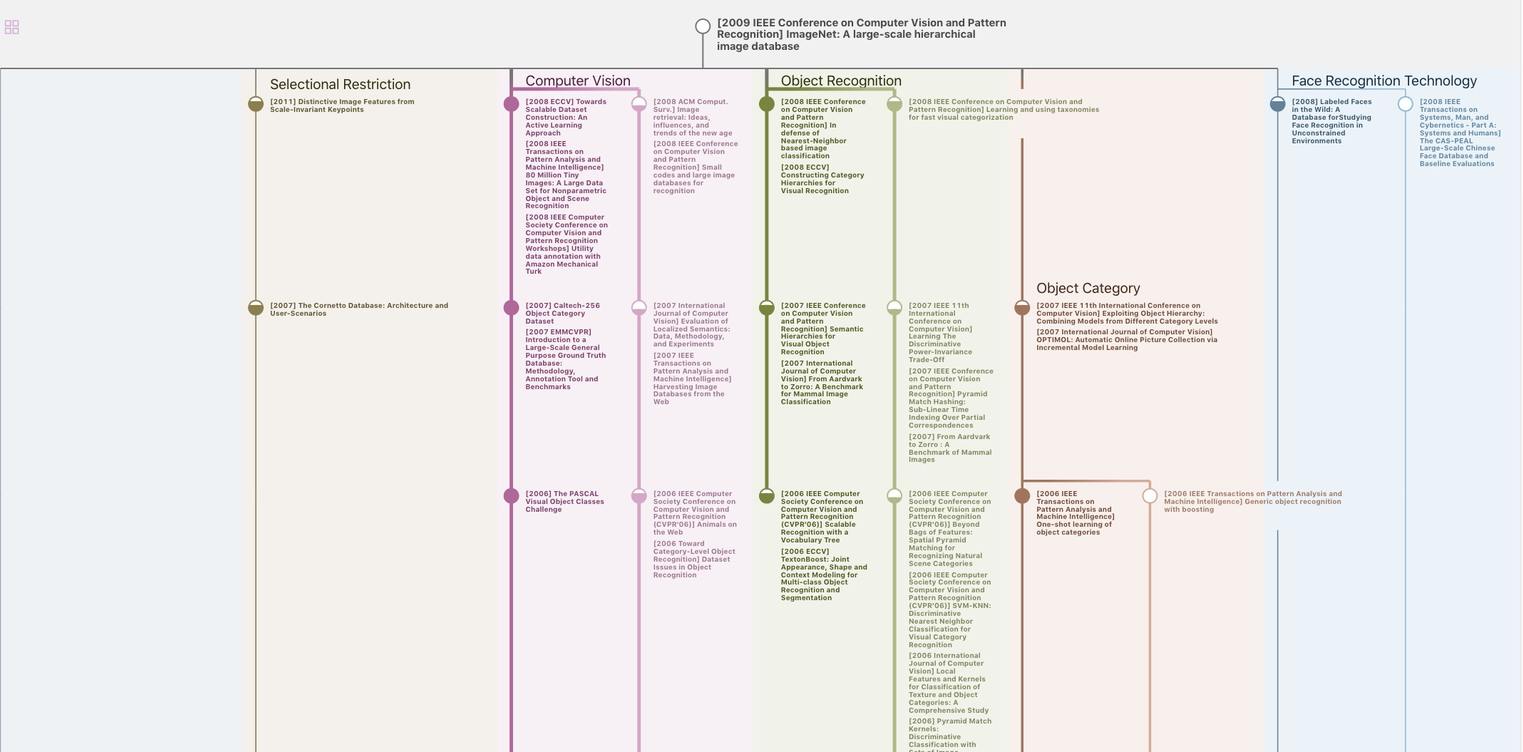
Generate MRT to find the research sequence of this paper
Chat Paper
Summary is being generated by the instructions you defined