Classifying Imbalanced Data Sets by a Novel RE-Sample and Cost-Sensitive Stacked Generalization Method
MATHEMATICAL PROBLEMS IN ENGINEERING(2018)
摘要
Learning with imbalanced data sets is considered as one of the key topics in machine learning community. Stacking ensemble is an efficient algorithm for normal balance data sets. However, stacking ensemble was seldom applied in imbalance data. In this paper, we proposed a novel RE-sample and Cost-Sensitive Stacked Generalization (RECSG) method based on 2-layer learning models. The first step is Level 0 model generalization including data preprocessing and base model training. The second step is Level 1 model generalization involving cost-sensitive classifier and logistic regression algorithm. In the learning phase, preprocessing techniques can be embedded in imbalance data learning methods. In the cost-sensitive algorithm, cost matrix is combined with both data characters and algorithms. In the RECSG method, ensemble algorithm is combined with imbalance data techniques. According to the experiment results obtained with 17 public imbalanced data sets, as indicated by various evaluation metrics (AUC, Geo Mean, and AGeoMean), the proposed method showed the better classification performances than other ensemble and single algorithms. The proposed method is especially more efficient when the performance of base classifier is low. All these demonstrated that the proposed method could be applied in the class imbalance problem.
更多查看译文
关键词
imbalanced data sets,stacked,generalization,re-sample,cost-sensitive
AI 理解论文
溯源树
样例
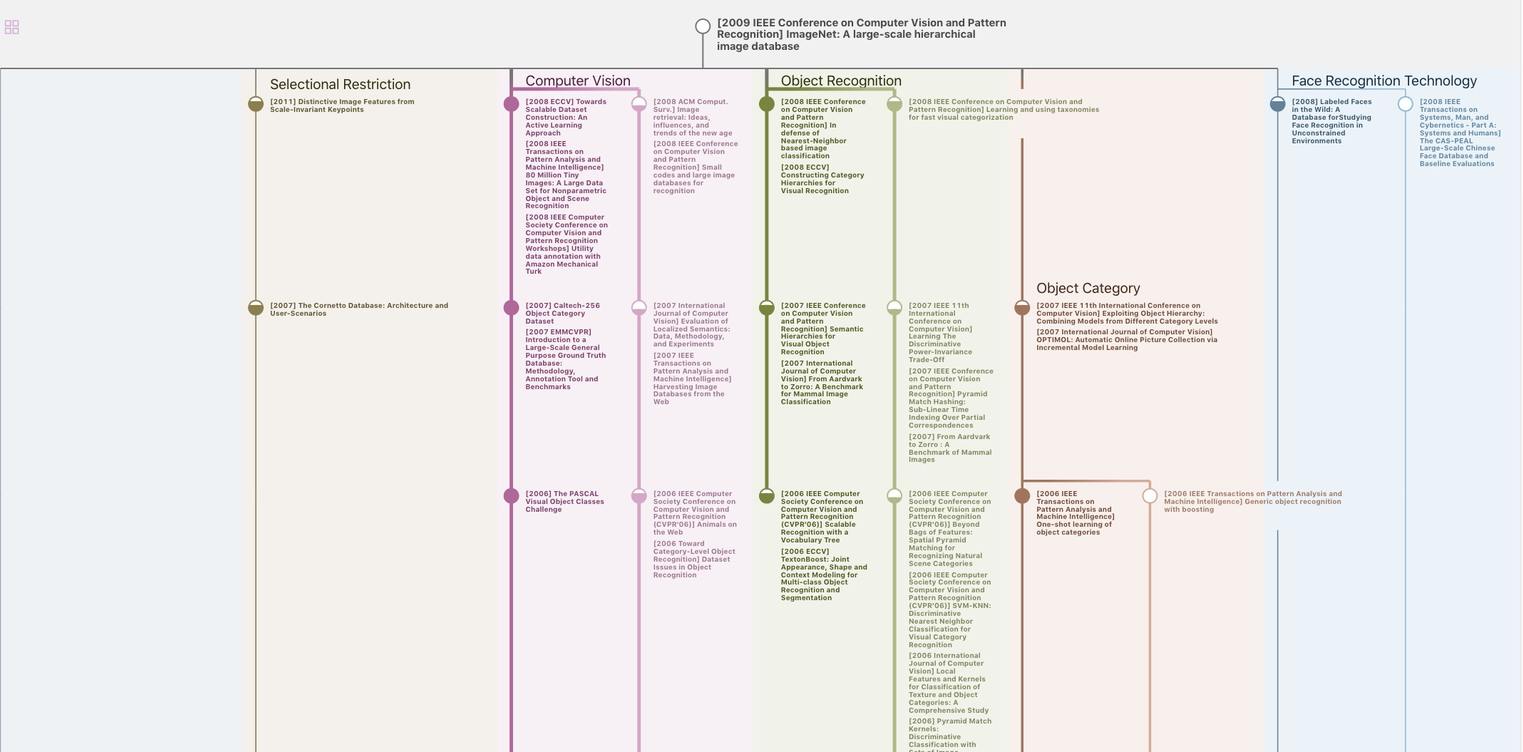
生成溯源树,研究论文发展脉络
Chat Paper
正在生成论文摘要