MKPLS: Multiple kernel partial least squares for transcription factor binding site identification
2017 CHINESE AUTOMATION CONGRESS (CAC)(2017)
摘要
The core of computational identification of transcription factor binding sites (TFBSs) is to deal with high dimensional and small sample size data and to handle the complex nonlinear relationships between features. Partial least squares (PLS) performs well in reducing dimensionality as well as explaining relations between multiple variables. Besides, kernel methods are widely applied to non-linear relationship process. Therefore, we reasonably introduce kernel partial least squares(KPLS) as a feature selection method for TFBS identification. Moreover, to lower the instability caused by the choice of kernel functions in conventional kernel-based methods, we combine multiple kernel methods with KPLS to develop a new method named multiple kernel PLS (MKPLS) to perform feature selection. PSO is utilized to estimate the parameters of linear combination of multiple kernels, furthermore, SVM is applied here to build an identification framework. 52 Escherichia coli k-12 TFBS datasets are used to test MKPLS as well as KPLS and Mutual Information(MI). Results demonstrate that MKPLS acquires a better ability to pick out the key features and can obtain a noticeable identification accuracy.
更多查看译文
关键词
transcription factor binding site, high dimensional and small sample size data, multiple kernel PIS, feature selection
AI 理解论文
溯源树
样例
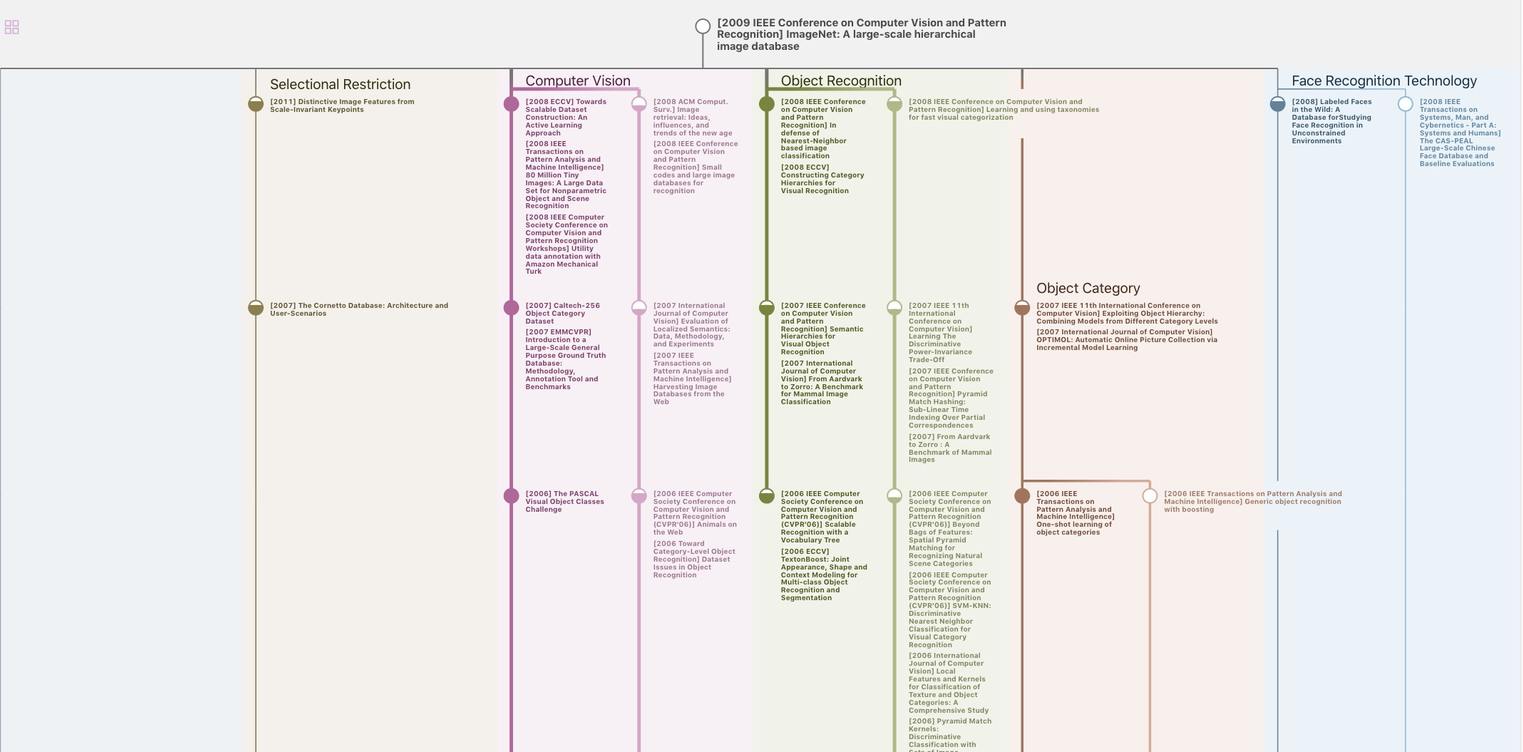
生成溯源树,研究论文发展脉络
Chat Paper
正在生成论文摘要