Fast Two-Stage Segmentation Based On Local Correntropy-Based K-Means Clustering
2017 IEEE 9TH INTERNATIONAL CONFERENCE ON COMMUNICATION SOFTWARE AND NETWORKS (ICCSN)(2017)
Abstract
A novel two-stage segmentation model is proposed for fast segmentation of intensity inhomogeneity images. In the first stage, the image is down-sampled and then segmented by local correntropy-based K-means clustering (LCK) method to get a coarse segmentation contour fast. In the second stage, the image is further segmented using an improved LCK method, which takes the up-sampled coarse segmentation contour of the first stage as the initialization. Due to the suitable initialization from the first stage, the second stage works well in accurately segmenting the image. To update the level set function quickly, we reform the two segmentation problems into the convex optimization framework, which efficiently provides a global minimizer to the LCK method. Experiments on synthetic and medical images shows that this two stage segmentation method has effective improvements on convergence rate, noise sensitivity and segmentation accuracy, compared with the state-of-the-art methods.
MoreTranslated text
Key words
image segmentation, active contours, level set methods, coarse segmentation, split Bregman method, accurate segmentation
AI Read Science
Must-Reading Tree
Example
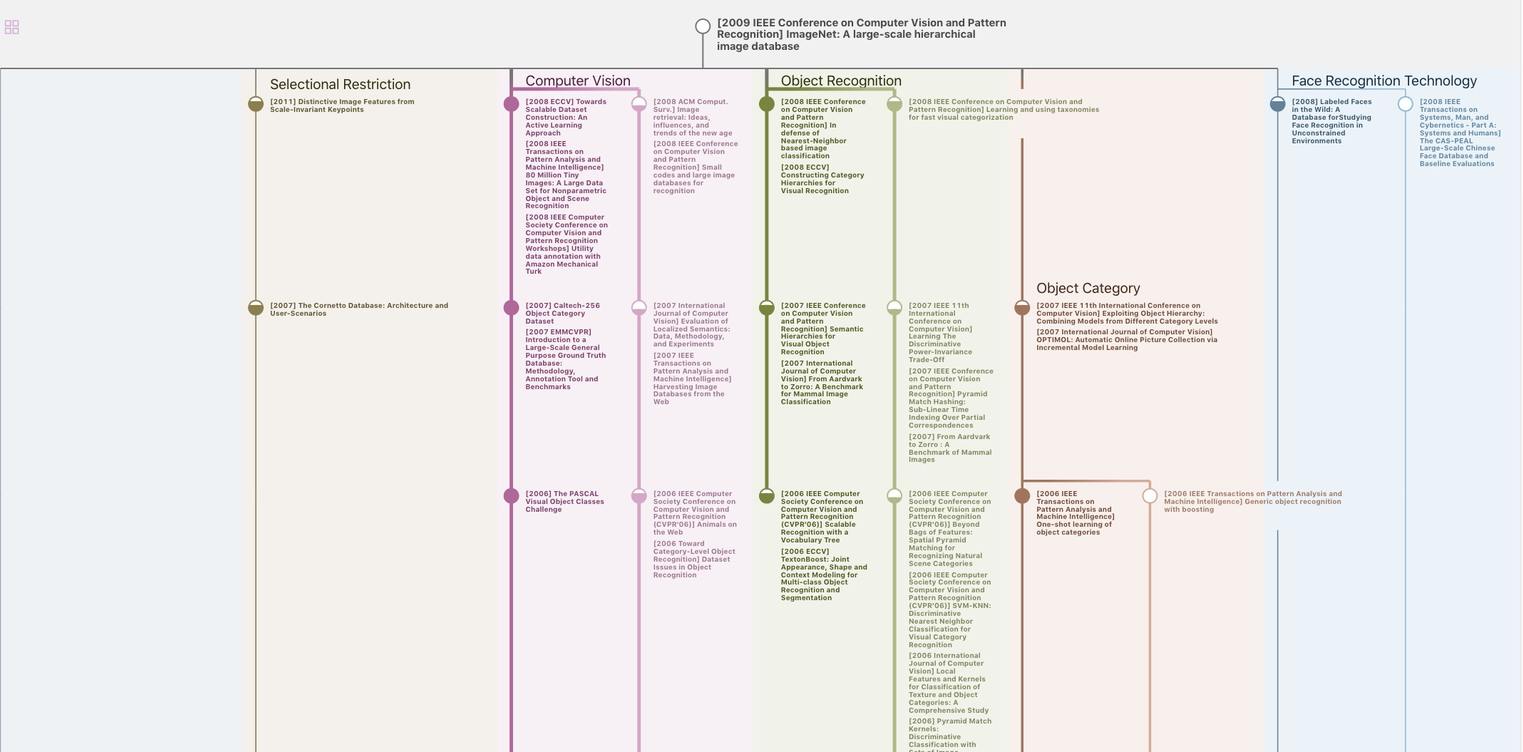
Generate MRT to find the research sequence of this paper
Chat Paper
Summary is being generated by the instructions you defined