Named Entity Recognition For Amharic Using Deep Learning
2017 IST-AFRICA WEEK CONFERENCE (IST-AFRICA)(2017)
摘要
The paper describes a named entity recognition system for Amharic, an under-resourced language, using a recurrent neural network, a bi-directional long short term memory model to identify and classify tokens into six predefined classes: Person, Location, Organization, Time, Title, and Other (non-named entity tokens). Word vectors based on semantic information are built for all tokens using an unsupervised learning algorithm, word2vec. The word vectors were merged with a set of specifically developed language independent features and together fed to the neural network model to predict the classes of the words. When evaluated by 10-fold cross-validation, the created Amharic named entity recogniser achieved good average precision (77.2%), but did worse on recall (63.4%), for a 69.7% F-1-score.
更多查看译文
关键词
Named Entity Recognition,Amharic,Under-resourced languages,Recurrent neural network,Long short term memory
AI 理解论文
溯源树
样例
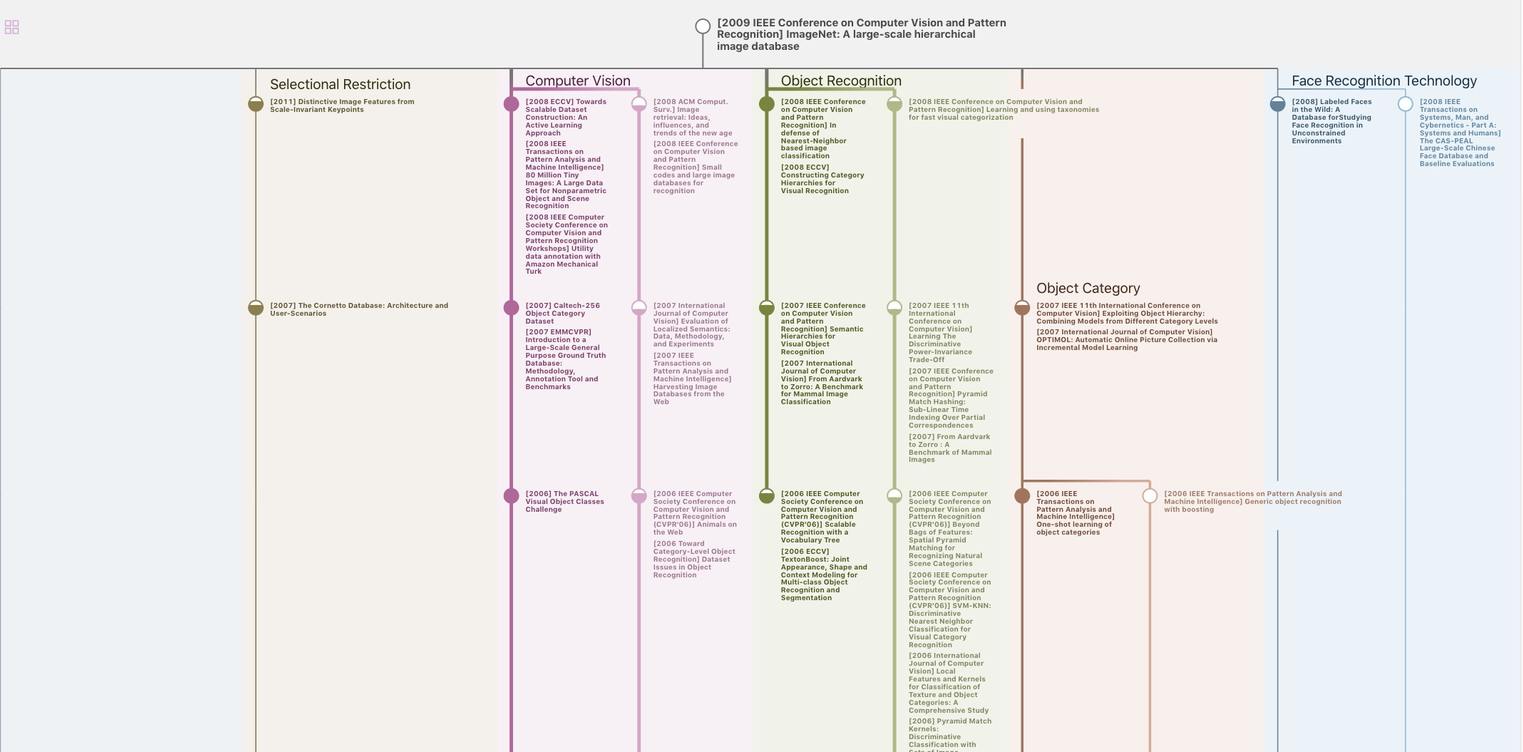
生成溯源树,研究论文发展脉络
Chat Paper
正在生成论文摘要