Proceedings of the 7th Annual Workshop on Audio/Visual Emotion Challenge
acm multimedia(2017)
摘要
It is our great pleasure to welcome you to the 7th Audio-Visual Emotion Challenge -- AVEC'17, held in conjunction with the ACM Multimedia 2017 in Mountain View, CA, USA.
This year's challenge and associated workshop continues to push the boundaries of audio-visual emotion and depression recognition towards real-life applications of behavioural computing. Looking back in the history of AVEC, the first challenge posed the problem of detecting discrete emotion classes on a large set of natural behaviour data. The second AVEC extended this problem to the prediction of continuous valued dimensional affect. This problem was enlarged further for the third edition to include the prediction of self-reported severity of depression. The fourth edition was a refined run with enriched annotations. The fifth AVEC introduced physiological signals, along with audio-visual data, for the prediction of dimensional affect. In the sixth edition, we introduced human-agent interactions for depression analysis, in addition to affect recognition. Finally, this year we've focused the study of affect from human behaviours captured 'in-the-wild', along with depression analysis from human-agent interactions.
The mission of AVEC challenge and workshop series is to provide a common benchmark test set for individual multimodal information processing and to bring together the audio, video and audio-visual emotion recognition communities, to compare the relative merits of the approaches to emotion recognition under well-defined and strictly comparable conditions and establish to what extent fusion of the approaches is possible and beneficial. The main underlying motivation is the need to advance emotion recognition and depression estimation for multimedia retrieval to a level where behaviours can be reliably sensed in real-life conditions, as this is exactly the type of data that applications would have to face in the real world.
更多查看译文
AI 理解论文
溯源树
样例
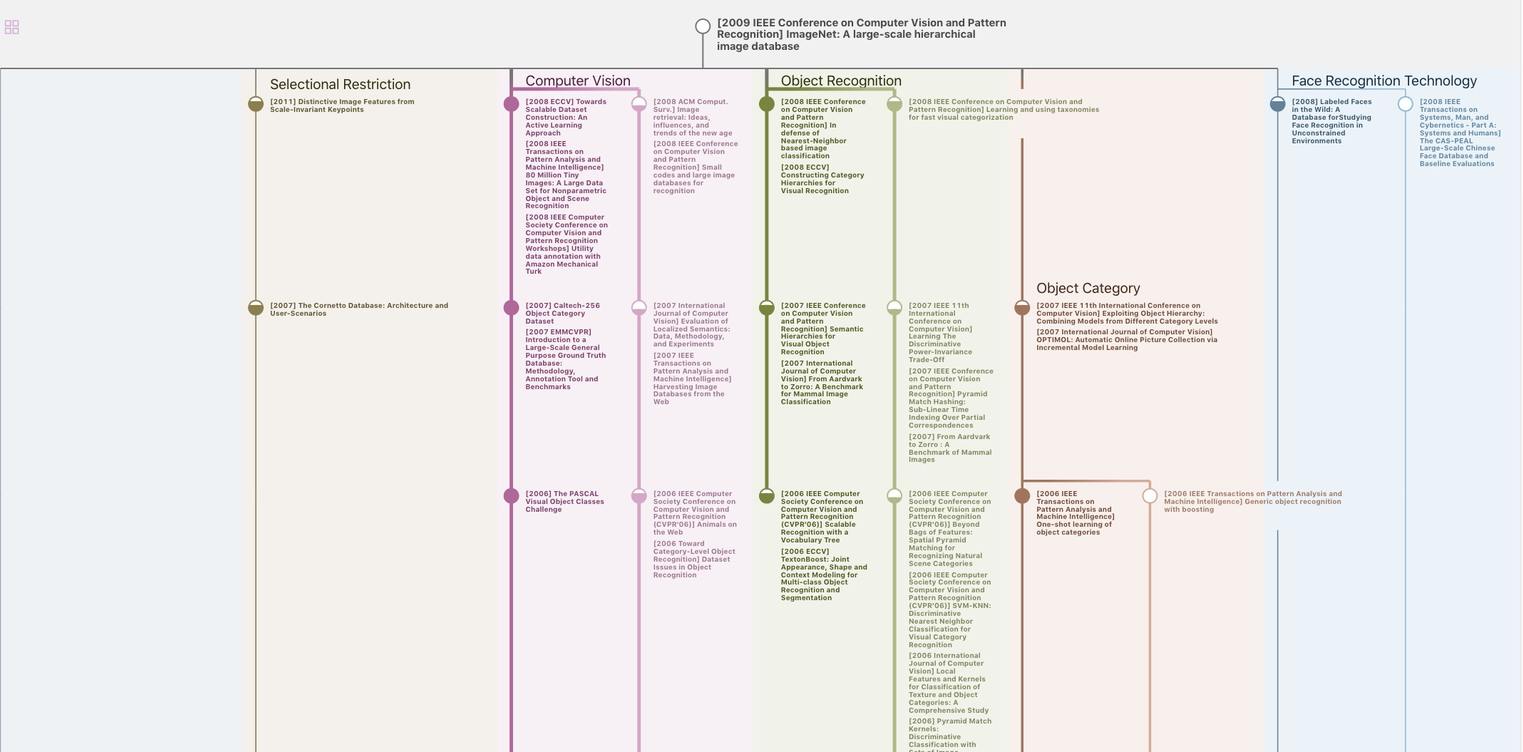
生成溯源树,研究论文发展脉络
Chat Paper
正在生成论文摘要