Optimal designs for minimising covariances among parameter estimators in a linear model
AUSTRALIAN & NEW ZEALAND JOURNAL OF STATISTICS(2017)
Abstract
We construct approximate optimal designs for minimising absolute covariances between least-squares estimators of the parameters (or linear functions of the parameters) of a linear model, thereby rendering relevant parameter estimators approximately uncorrelated with each other. In particular, we consider first the case of the covariance between two linear combinations. We also consider the case of two such covariances. For this we first set up a compound optimisation problem which we transform to one of maximising two functions of the design weights simultaneously. The approaches are formulated for a general regression model and are explored through some examples including one practical problem arising in chemistry. This paper establishes optimality conditions for a non-standard criterion function (neither convex nor concave). We construct optimal designs such that certain parameter estimators in a regression model are uncorrelated or as uncorrelated as possible.
MoreTranslated text
Key words
Lagrangian optimality conditions,multiplicative algorithms,near uncorrelated parameter estimators,optimal design theory,vertex directional derivatives
AI Read Science
Must-Reading Tree
Example
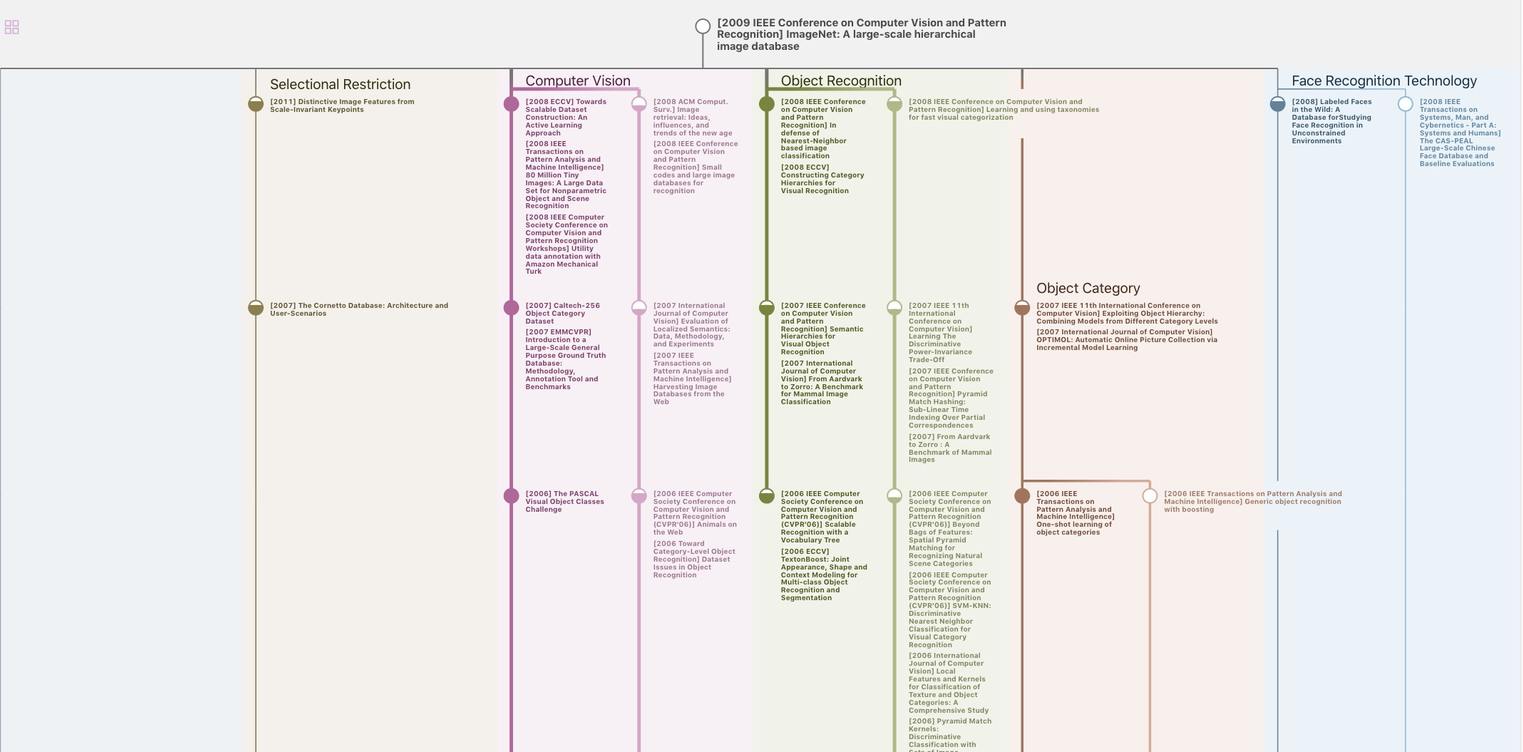
Generate MRT to find the research sequence of this paper
Chat Paper
Summary is being generated by the instructions you defined