Improved Data Collection from Online Sources Using Query Expansion and Active Learning
Social Science Research Network(2017)
摘要
Datasets derived from searching online textual sources, such as social media sites and news article repositories are increasingly used in political science research. Common approaches for retrieving such data are mostly based on keyword queries, and lack systematic evaluation of the quality of the retrieved sample. Based on the framework proposed in Li et al. (2014) I propose a methodology that combines approaches from machine learning and natural language processing to improve the identification of relevant data in large text corpora, while minimizing the required amount of human supervision. It consists of two steps. First, a larger set of data is retrieved from the total population using keywords. In the second step, a machine learning approach is taken to separate the initial set into relevant and irrelevant tweets. Information from the labeled data is then used to suggest additional keywords to expand the initial query. I evaluate the approach in a case study, retrieving Tweets about the German refugee crisis from a large dataset of German language Tweets. The proposed approach provides increased precision and recall as well as substantive representativeness, compared to commonly applied data retrieval strategies. I additionally provide software that implements the algorithm specifically for Twitter and makes it accessible for applied researchers.
更多查看译文
AI 理解论文
溯源树
样例
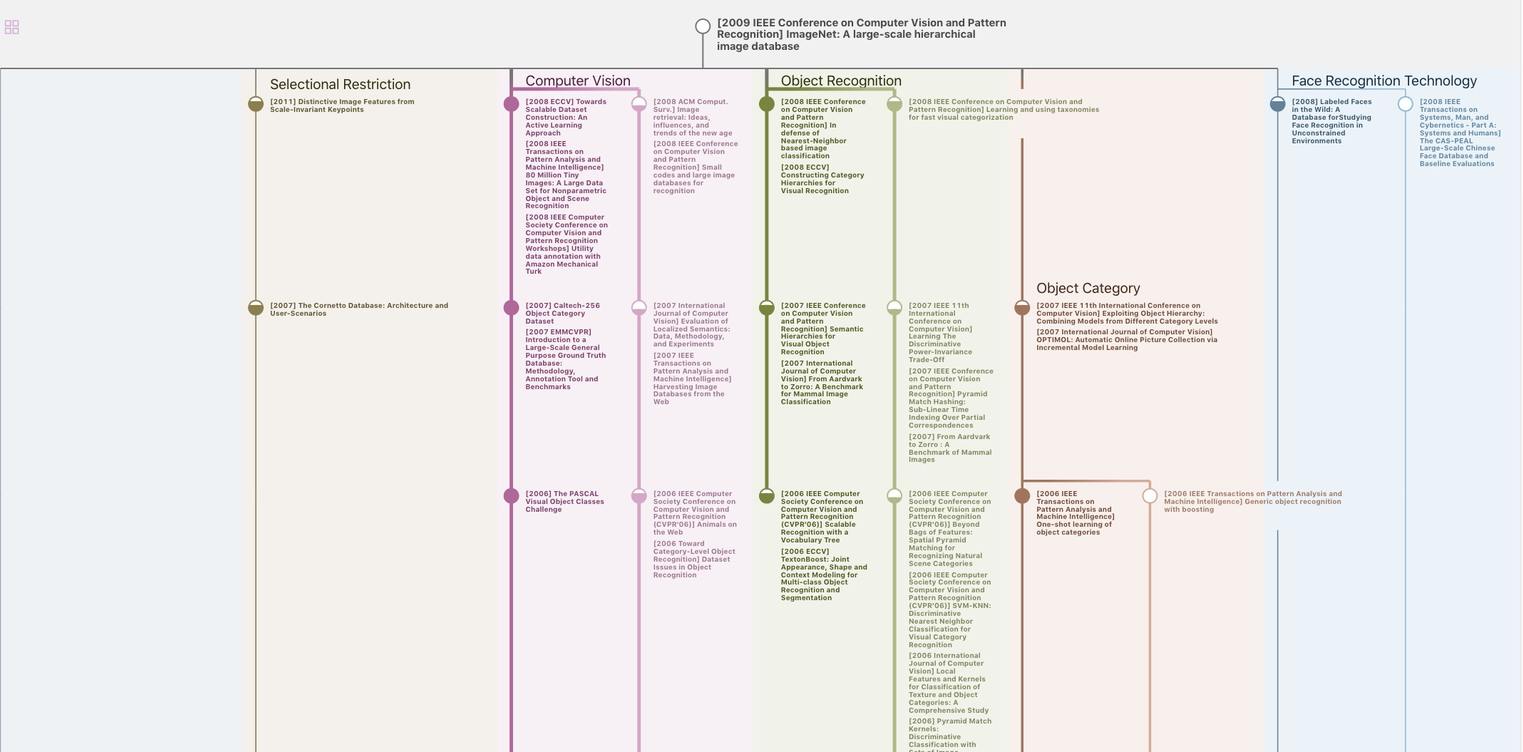
生成溯源树,研究论文发展脉络
Chat Paper
正在生成论文摘要