Fisher information under Gaussian quadrature models
STATISTICA NEERLANDICA(2018)
Abstract
This paper develops formulae to compute the Fisher information matrix for the regression parameters of generalized linear models with Gaussian random effects. The Fisher information matrix relies on the estimation of the response variance under the model assumptions. We propose two approaches to estimate the response variance: the first is based on an analytic formula (or a Taylor expansion for cases where we cannot obtain the closed form), and the second is an empirical approximation using the model estimates via the expectation-maximization process. Further, simulations under several response distributions and a real data application involving a factorial experiment are presented and discussed. In terms of standard errors and coverage probabilities for model parameters, the proposed methods turn out to behave more reliably than does the disparity rule' or direct extraction of results from the generalized linear model fitted in the last expectation-maximization iteration.
MoreTranslated text
Key words
Gaussian random effects,generalized linear models,overdispersion,standard errors
AI Read Science
Must-Reading Tree
Example
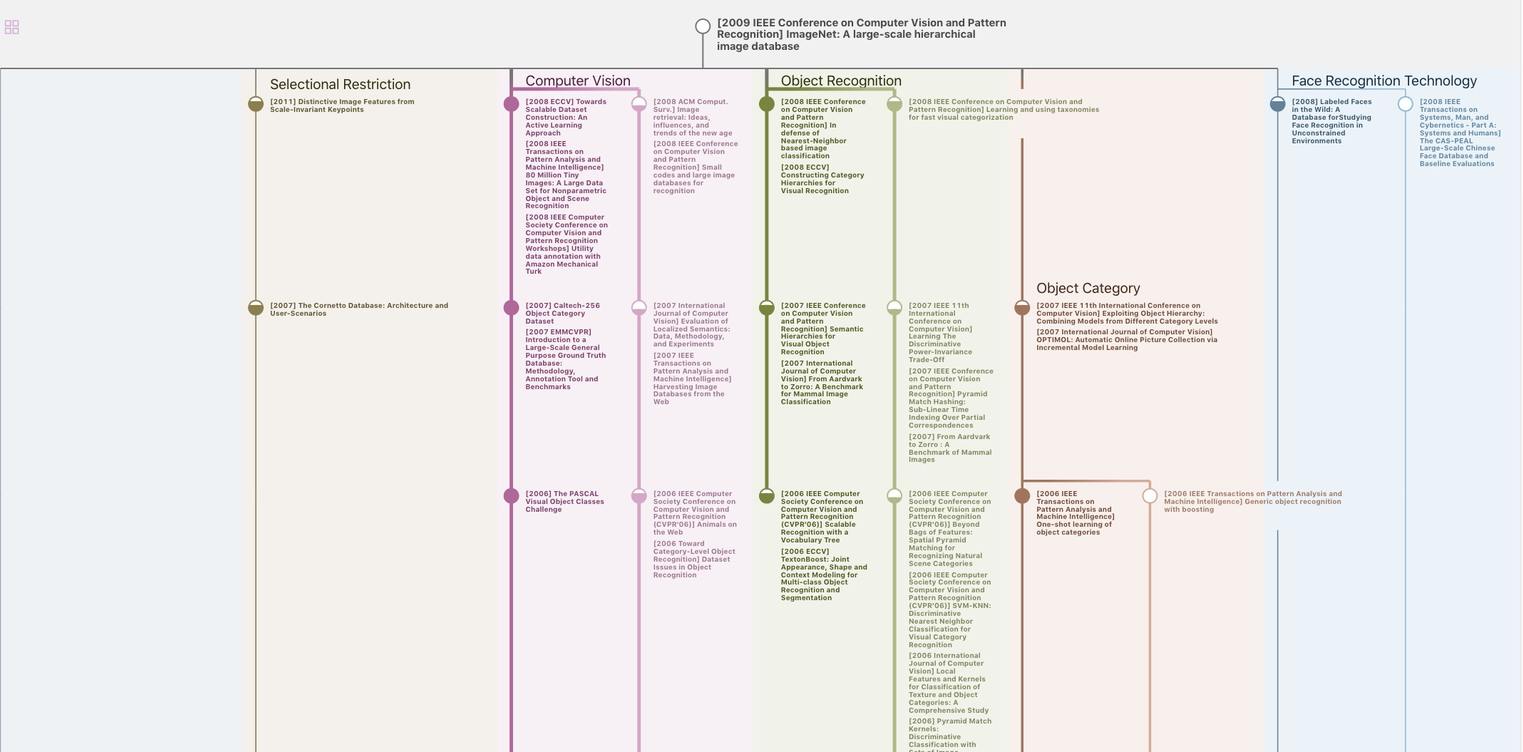
Generate MRT to find the research sequence of this paper
Chat Paper
Summary is being generated by the instructions you defined