Load-Capacity Rating of Bridge Populations through Machine Learning: Application of Decision Trees and Random Forests
JOURNAL OF BRIDGE ENGINEERING(2017)
摘要
The functionality of the U.S. transportation infrastructure system is dependent upon the health of an aging network of over 600,000 bridges, and agencies responsible for maintaining these bridges rely on the process of load rating to assess the adequacy of individual structures. This paper presents a new approach for safety screening and load-capacity evaluation of large bridge populations that seeks to uncover heretofore unseen patterns within the National Bridge Inventory database and establish relationships between select bridge attributes and their load-capacity status. Decision-tree and random-forest classification models were trained on the national concrete slab bridge data set of over 40,000 structures. The resulting models were validated on an independent data set and then compared with a number of existing judgment-based schemes found in an extensive survey of the current state of practice in the United States. The proposed approach offers a method that provides guidance for improved allocation of resources by informing maintenance decisions through rapid identification of candidate bridges that require further scrutiny for either possible load restriction or restriction removal. (C) 2017 American Society of Civil Engineers.
更多查看译文
关键词
National Bridge Inventory (NBI),Data-driven,Load rating,Load posting,Decision trees,Random forests
AI 理解论文
溯源树
样例
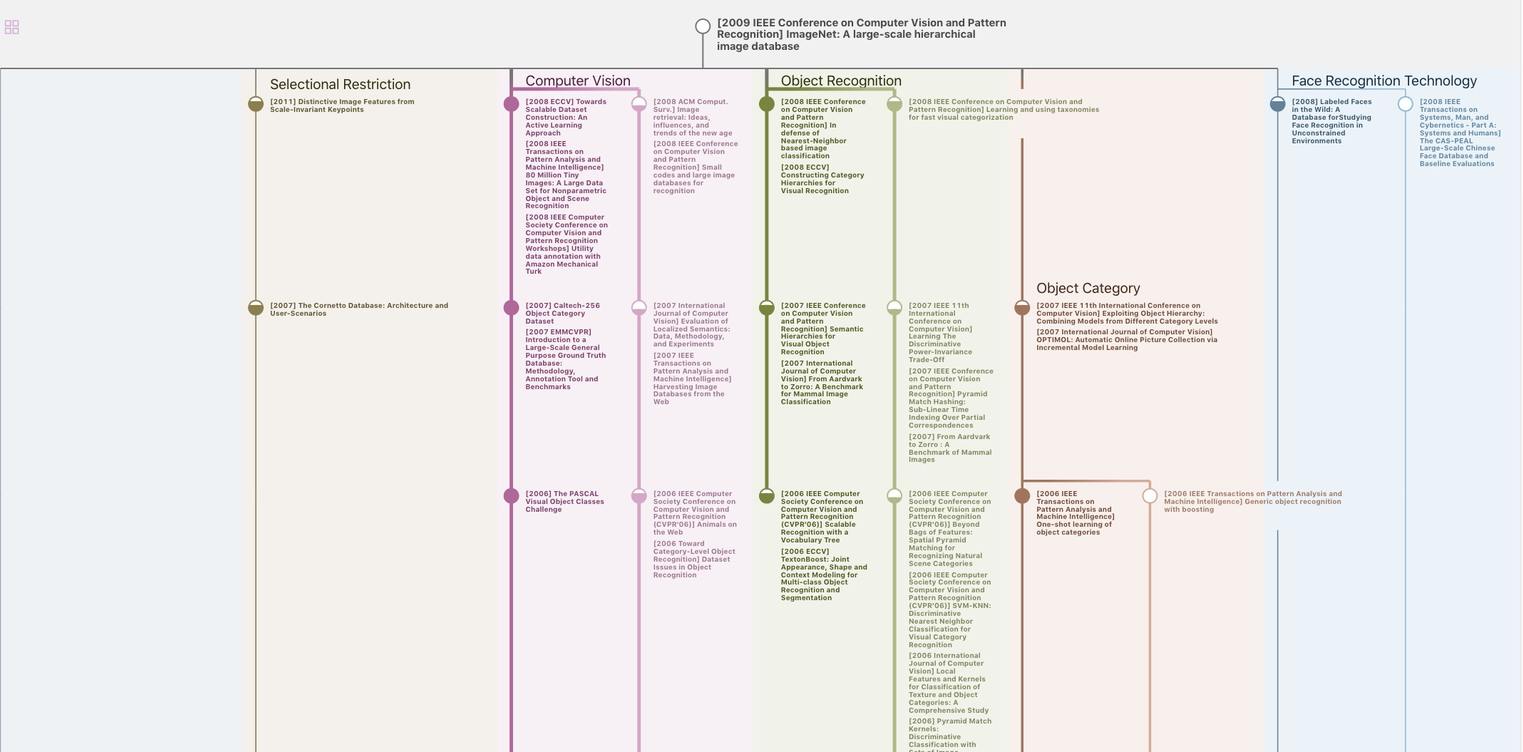
生成溯源树,研究论文发展脉络
Chat Paper
正在生成论文摘要