A nonparametric test for stationarity in functional time series
arXiv: Methodology(2021)
摘要
We propose a new measure for stationarity of a functional time series, which is based on an explicit representation of $L^2$-distance between spectral density operator of a non-stationary process and its best ($L^2$-)approximation by a spectral density operator corresponding to a stationary process. This distance can easily be estimated by sums of Hilbert-Schmidt inner products of periodogram operators (evaluated at different frequencies), and asymptotic normality of an appropriately standardised version of estimator can be established for corresponding estimate under null hypothesis and alternative. As a result we obtain confidence intervals for discrepancy of underlying process from a functional stationary process and a simple asymptotic frequency domain level $alpha$ test (using quantiles of normal distribution) for hypothesis of stationarity of functional time series. Moreover, new methodology allows also to test precise hypotheses of form the functional time series is approximately stationarity, which means that new measure of stationarity is smaller than a given threshold. Thus in contrast to methods proposed in literature our approach also allows to test for relevant deviations from stationarity. We demonstrate in a small simulation study that new method has very good finite sample properties and compare it with currently available alternative procedures. Moreover, we apply our test on annual temperature curves.
更多查看译文
AI 理解论文
溯源树
样例
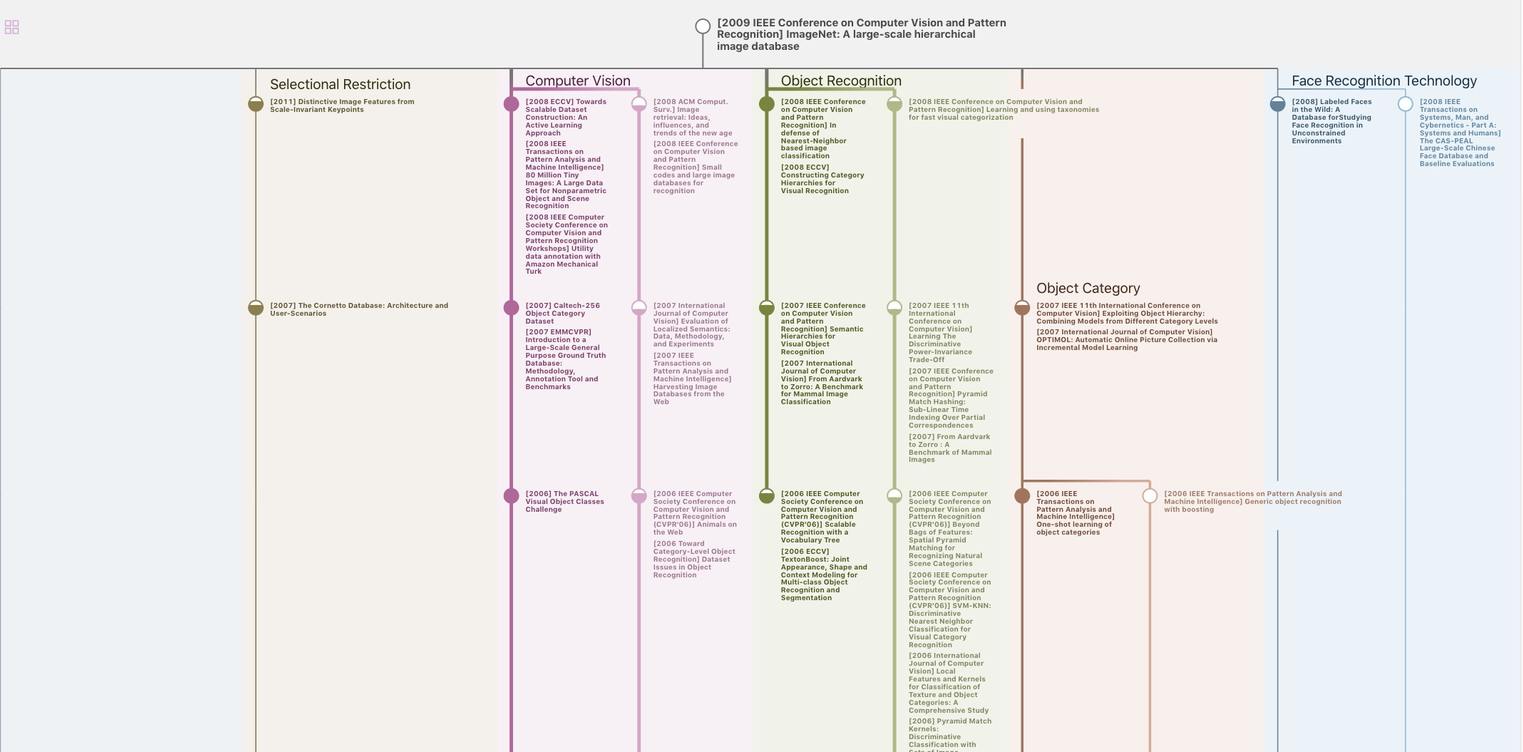
生成溯源树,研究论文发展脉络
Chat Paper
正在生成论文摘要