Incremental basis estimation adopting global k-means algorithm for NMF-based noise reduction
Applied Acoustics(2018)
Abstract
Nonnegative matrix factorization (NMF) is a data decomposition technique enabling to discover meaningful latent nonnegative components. Since, however, the objective function of NMF is non-convex, the performance of the source separation can degrade when the iterative update of the basis matrix in the training procedure is stuck to a poor local minimum. In most of the previous studies, the whole basis matrix for a specific source is iteratively updated to minimize a certain objective function with random initialization although a few approaches have been proposed for the systematic initialization of the basis matrix such as the singular value decomposition and k-means clustering. In this paper, we propose an approach to robust bases estimation in which an incremental strategy is adopted. Based on an analogy between clustering and NMF analysis, we estimate the NMF bases in a similar way to the global k-means algorithm popular in the data clustering area. Experiments on audio separation from noise showed that the proposed methods outperformed the conventional NMF technique using random initialization by about 2.04dB and 2.34dB in signal-to-distortion ratio when the target source was speech and violin, respectively.
MoreTranslated text
Key words
Nonnegative matrix factorization,Basis training,Incremental approach,Global k-means,Source separation
AI Read Science
Must-Reading Tree
Example
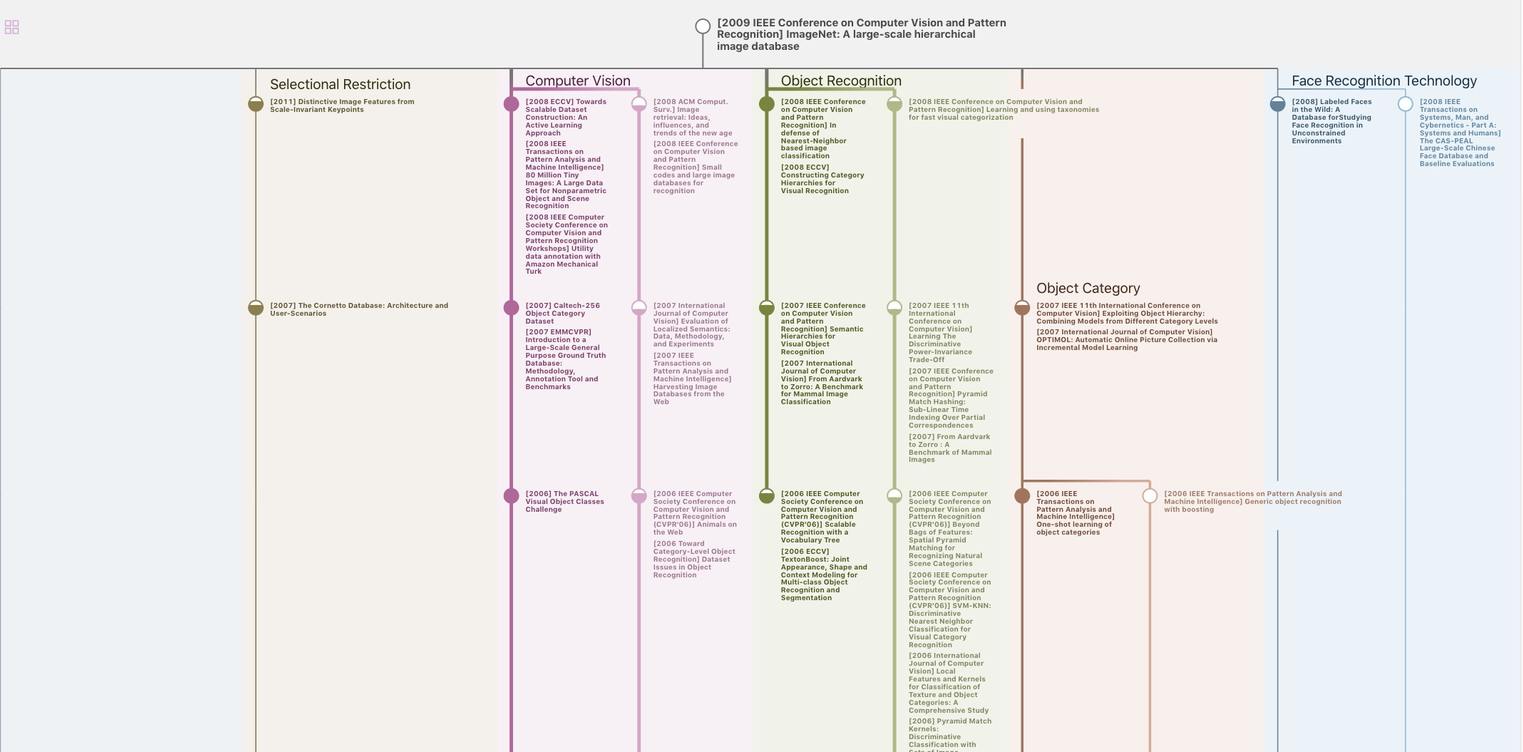
Generate MRT to find the research sequence of this paper
Chat Paper
Summary is being generated by the instructions you defined