Fault detection based on weighted difference principal component analysis
JOURNAL OF CHEMOMETRICS(2017)
Abstract
Recently, multivariate statistical methods, such as principal component analysis (PCA), have drawn increasing attention for fault detection applications in industrial processes. However, industrial processes typically have complex multimodal and nonlinear characteristics. In these situations, the traditional PCA method performs poorly due to its assumption that the process data are linear and unimodal. To improve fault detection performance in nonlinear and multimode industrial processes, this paper proposes a new fault detection method based on weighted difference principal component analysis (WDPCA). Weighted difference principal component analysis first eliminates the multimodal and nonlinear characteristics of the original data by using the weighted difference method. Then, PCA is applied to the preprocessed data, neglecting the influences of multimodality and nonlinearity. Two numerical examples and an industrial application in a semiconductor manufacturing process are used to verify the effectiveness of WDPCA. The simulation results demonstrate that WDPCA shows better fault detection performance than the PCA, kernel principal component analysis (KPCA), independent component analysis (ICA), k-nearest neighbor rule (kNN), and local outlier factor (LOF) methods. Multivariate statistical methods have drawn increasing attention for fault detection applications in industrial processes. However, aiming at the problem that industrial processes have complex multimodal and nonlinear characteristics, PCA method performs poorly. To improve it's fault detection performance in these processes, this paper proposes a new fault-detection method based on weighted difference principal component analysis (WDPCA). WDPCA first eliminates the multimodal and nonlinear characteristics of original data using the weighted difference method. Then, PCA is applied to the preprocessed data, neglecting the influences of multimodality and nonlinearity. Three examples are used to verify the effectiveness of WDPCA compared with other methods.
MoreTranslated text
Key words
fault detection,multimodal data,nonlinear data,principal component analysis,weighted difference principal component analysis
AI Read Science
Must-Reading Tree
Example
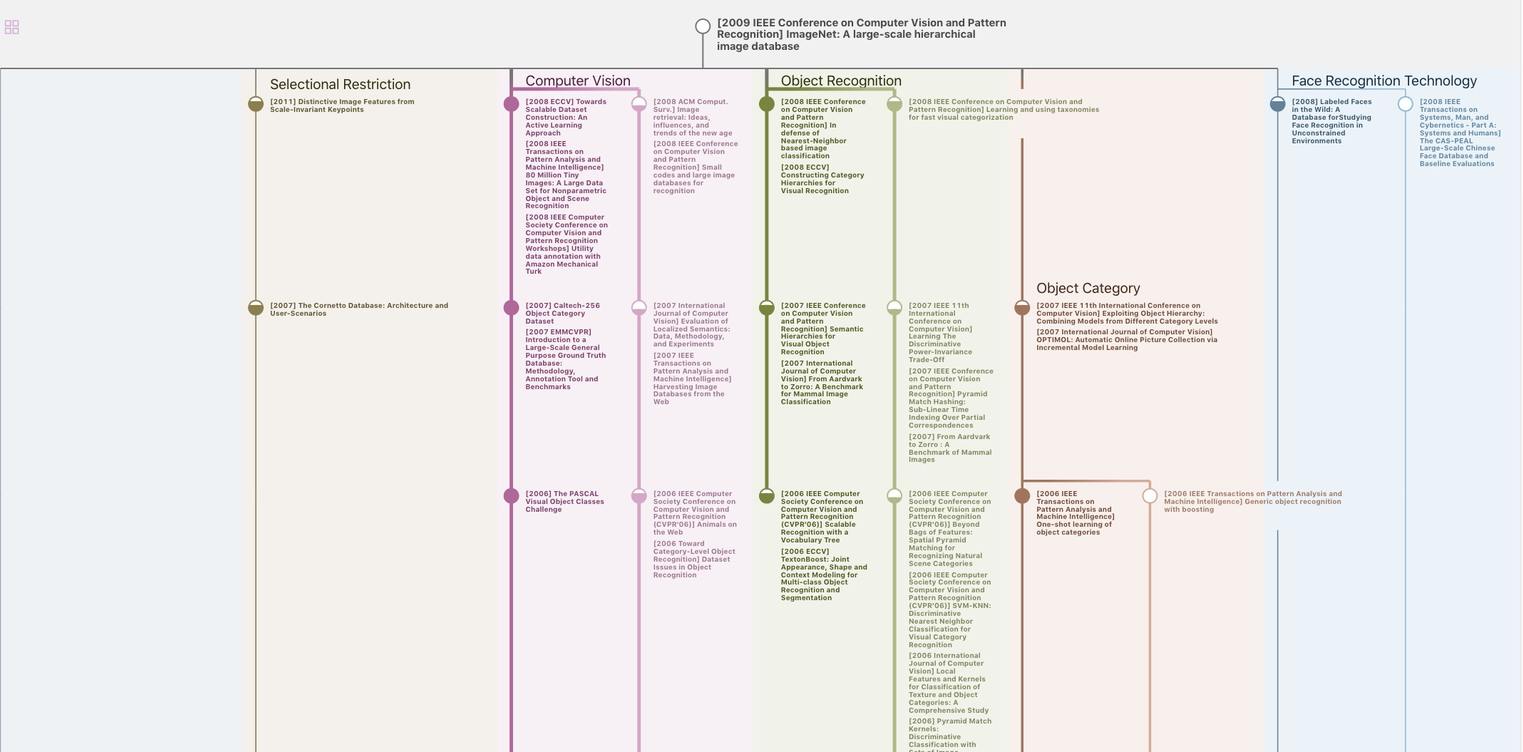
Generate MRT to find the research sequence of this paper
Chat Paper
Summary is being generated by the instructions you defined