Confidence Regions for Spatial Excursion Sets From Repeated Random Field Observations, With an Application to Climate
JOURNAL OF THE AMERICAN STATISTICAL ASSOCIATION(2018)
Abstract
The goal of this article is to give confidence regions for the excursion set of a spatial function above a given threshold from repeated noisy observations on a fine grid of fixed locations. Given an asymptotically Gaussian estimator of the target function, a pair of data-dependent nested excursion sets are constructed that are sub- and super-sets of the true excursion set, respectively, with a desired confidence. Asymptotic coverage probabilities are determined via a multiplier bootstrap method, not requiring Gaussianity of the original data nor stationarity or smoothness of the limiting Gaussian field. The method is used to determine regions in North America where the mean summer and winter temperatures are expected to increase by mid-21st century by more than 2 degrees Celsius.
MoreTranslated text
Key words
Functional data,Gaussian kinematic formula,General linear model,Level sets,Multiplier bootstrap,Spatial statistics
AI Read Science
Must-Reading Tree
Example
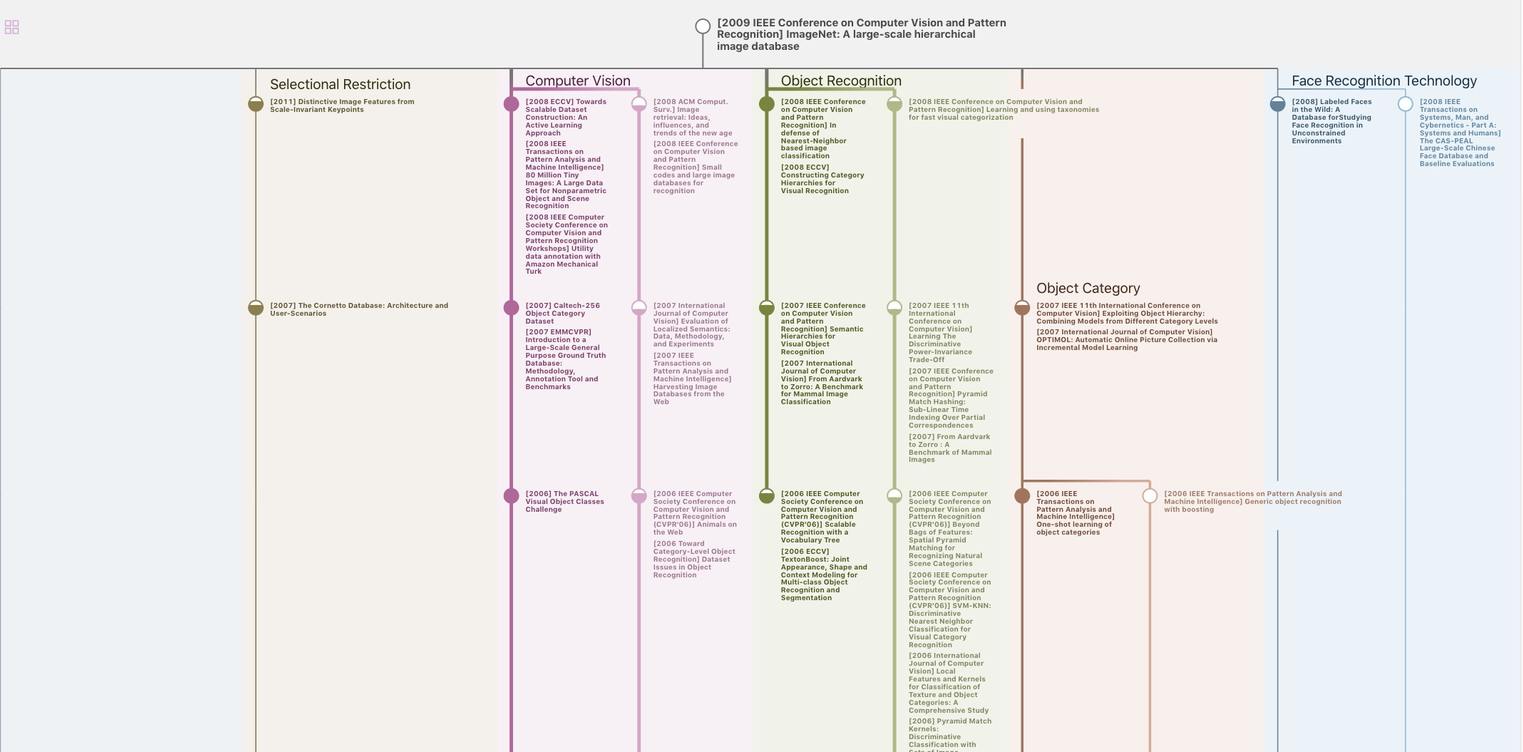
Generate MRT to find the research sequence of this paper
Chat Paper
Summary is being generated by the instructions you defined