Application of Feature-Learning Methods Toward Product Usage Context Identification and Comfort Prediction
JOURNAL OF COMPUTING AND INFORMATION SCIENCE IN ENGINEERING(2018)
摘要
Usage context is considered a critical driving factor for customers' product choices. In addition, physical use of a product (i.e., user-product interaction) dictates a number of customer perceptions (e.g., level of comfort). In the emerging internet of things (IoT), this work hypothesizes that it is possible to understand product usage and level of comfort while it is "in-use" by capturing the user-product interaction data. Mining this data to understand both the usage context and the comfort of the user adds new capabilities to product design. There has been tremendous progress in the field of data analytics, but the application in product design is still nascent. In this work, application of feature-learning methods for the identification of product usage context and level of comfort is demonstrated, where usage context is limited to the activity of the user. A novel generic architecture using foundations in convolutional neural network (CNN) is developed and applied to a walking activity classification using smartphone accelerometer data. Results are compared with feature-based machine learning algorithms (neural network and support vector machines (SVM)) and demonstrate the benefits of using the feature-learning methods over the feature-based machine-learning algorithms. To demonstrate the generic nature of the architecture, an application toward comfort level prediction is presented using force sensor data from a sensor-integrated shoe.
更多查看译文
AI 理解论文
溯源树
样例
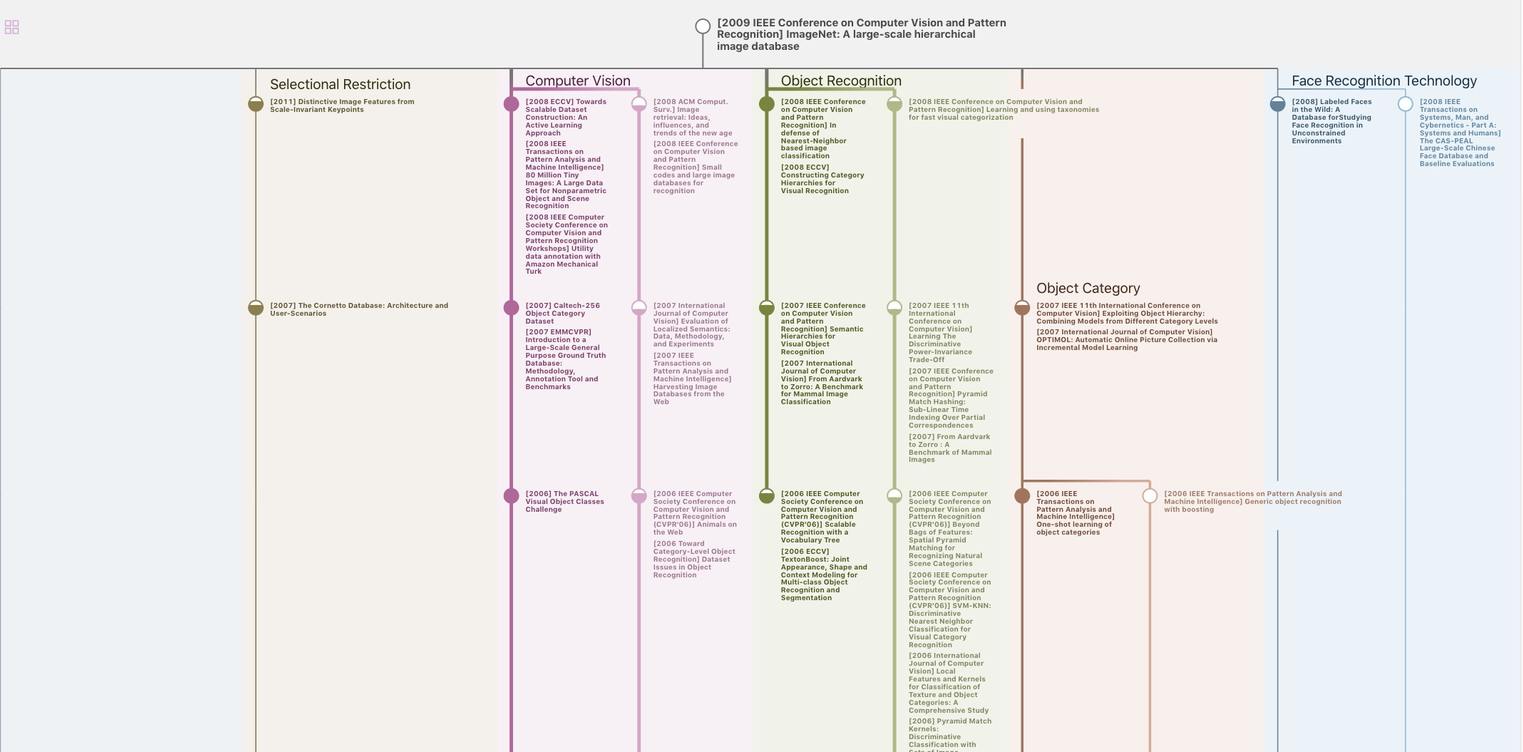
生成溯源树,研究论文发展脉络
Chat Paper
正在生成论文摘要