Voids identification in rubberized mortar digital images using K-Means and Watershed algorithms
Journal of Cleaner Production(2017)
摘要
In the construction industry, the use of sustainable raw materials has become a challenge. Rubber scrap tires have been investigated by several researchers as an alternative aggregate to conventional because it has become a major environmental problem in all parts of the world. Scanning Electron Microscopy (SEM) images are contributing to the understanding of the influence of this type of aggregate in the main physical and mechanical properties of cementitious composites. Voids derived from the use of rubber as aggregate in mortar can be found by SEM technique; however, that depends on a visual analysis, having a low accuracy of the recognition. To do so, image processing techniques can be used to fill the gap by increasing the reliability of the microstructural analysis. In this work, tire rubber waste is used in 0%, 5%, 10%, 15% and 30%, replacing, in mass, the conventional aggregate. Density, voids index, water absorption and mechanical resistance of the rubberized mortars were evaluated. Microscopic images were collected by scanning electron microscopy and subsequently applied to image processing technique for quantitative identification of voids in each mixture with rubber. Rubberized mortars showed lower mechanical strength compared to conventional sample. However, that is due to the voids increase in matrix mortars rubber, a property that could be calculated by SEM image analysis with the aid of image processing technique, K-Means algorithm and Watershed, which showed an accuracy of 88% identification in relation to the visual analysis. The best characterization of rubberized mortars pathologies is an important tool in the fight against mechanical strength, contributing to the increase of the use of rubber as an aggregate, which reduces the extraction of natural materials and the inadequate disposition of waste tires.
更多查看译文
关键词
Rubberized mortar voids,Alternative materials,Scanning electron microscopy,Image processing,K-means,Watershed
AI 理解论文
溯源树
样例
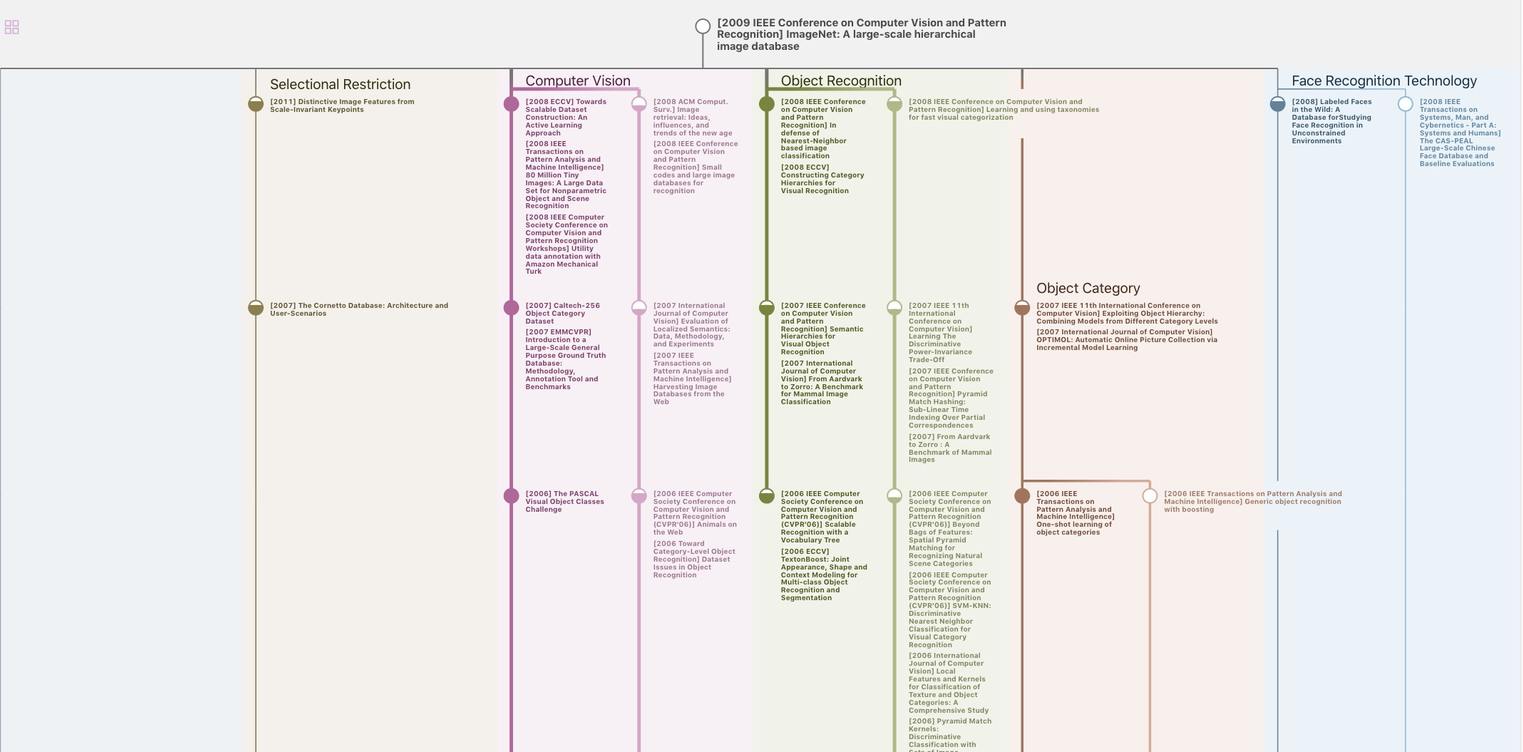
生成溯源树,研究论文发展脉络
Chat Paper
正在生成论文摘要