Exploring the major factors affecting fly-ash concrete carbonation using artificial neural network
Neural Computing and Applications(2017)
Abstract
Concrete carbonation is one of the main causes of corrosion of the reinforcement and consequently causing damage to the reinforced concrete structures. The progress of the carbonation front depends on many factors including mixture proportions and exposure conditions. Several carbonation prediction models including mathematical and analytical predictions are available. Most of these models, however, are based on simple regression equations and cannot predict or accurately reflect the various factors involved in concrete carbonation. The current published results in this issue are in conflict. In view of this, our research aims to apply an artificial neural network (ANN) approach for predicting the carbonation of fly-ash concrete taking into account the most influential parameters, including mixture proportions and exposure conditions. Six parameters were considered as inputs to the ANN model, covering, binder and fly-ash content, water-to-binder ratio, CO 2 concentration, relative humidity, and time of exposure; one output is carbonation depth. The ANN model was prepared, trained, and tested with 300 datasets from experiments as well as past research. The performance of training, validation, and test sets shows a high correlation between the experimental and the ANN predicted values of the carbonation depth. In addition, the proposed prediction model was in good agreement with the experimental data in comparison with other model. This study concludes that the use of this model for numerical investigations on the parameters affecting the carbonation depth in fly-ash concrete is successful and provides scientific guidance for durability design.
MoreTranslated text
Key words
Carbonation depth, Fly-ash, Concrete, Artificial neural network
AI Read Science
Must-Reading Tree
Example
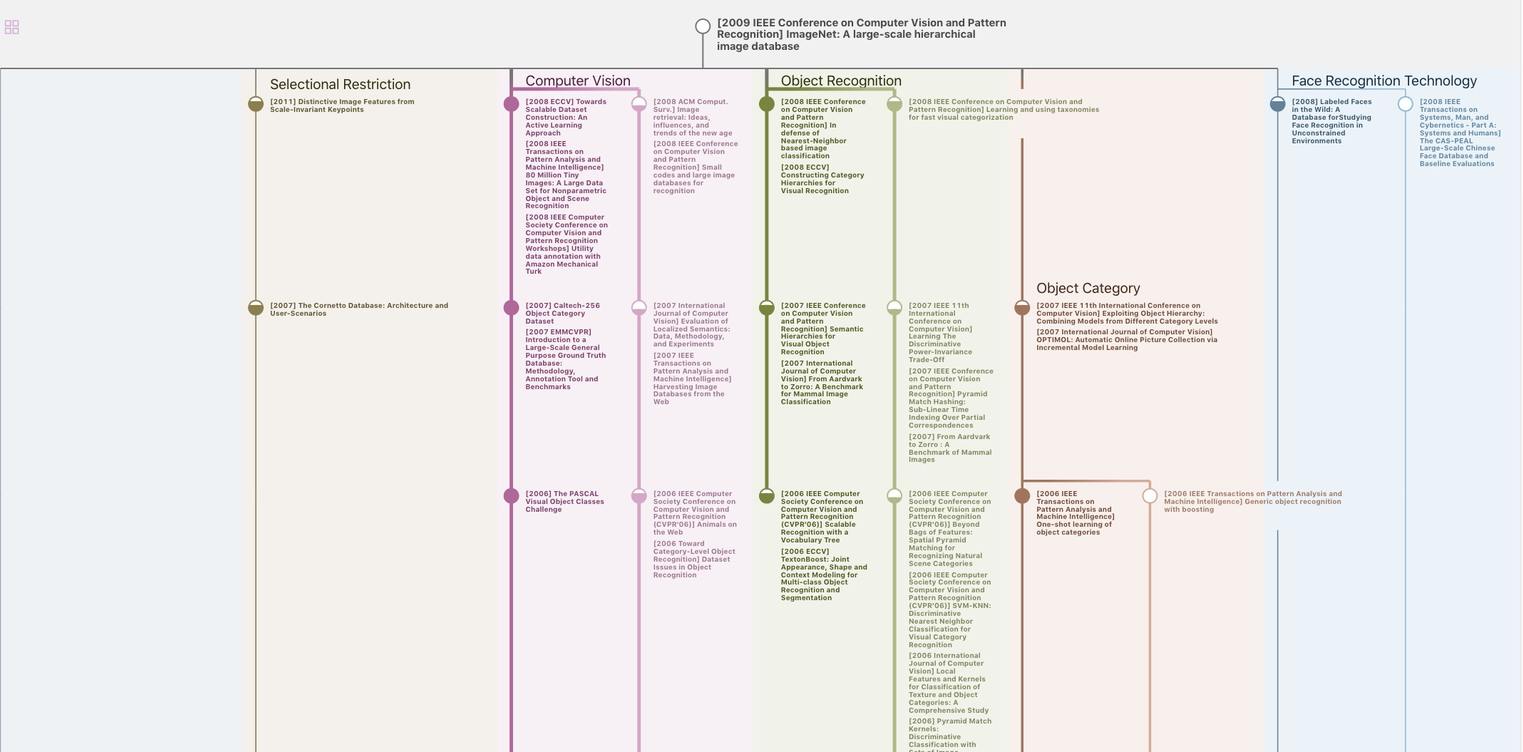
Generate MRT to find the research sequence of this paper
Chat Paper
Summary is being generated by the instructions you defined