Not All Experimental Questions Are Created Equal: Accelerating Biological Data to Knowledge Transformation (BD2K) via Science Informatics, Active Learning and Artificial Intelligence
bioRxiv(2017)
摘要
The fathers of Artificial Intelligence (AI) dreamt about the application of AI to Scientific Discovery. We propose a new twist on these early dreams and describe a novel approach aimed at remodeling of the biomedical research infrastructure and catalyze gene function determination. We aim to start a bold discussion of new ideas aimed towards increasing the efficiency of the allocation of research capacities, reproducibility, provenance tracking, removing redundancy and catalyzing knowledge gain with each experiment. In particular, we describe a tractable computational framework and infrastructure that can help researchers assess the potential information gain of millions of experiments before conducting them. The utility of experiments in this paper is modeled as the predictive knowledge (formalized as information) to be gained as a result of performing an experiment. The experimentalist would then be empowered to select experiments that maximize information gain if they wished, recognizing that there are frequently other considerations, such as a specific technological or medical utility, that might override the priority of maximizing information gain. The conceptual approach we develop is general, and here we apply it to the study of gene function.
更多查看译文
AI 理解论文
溯源树
样例
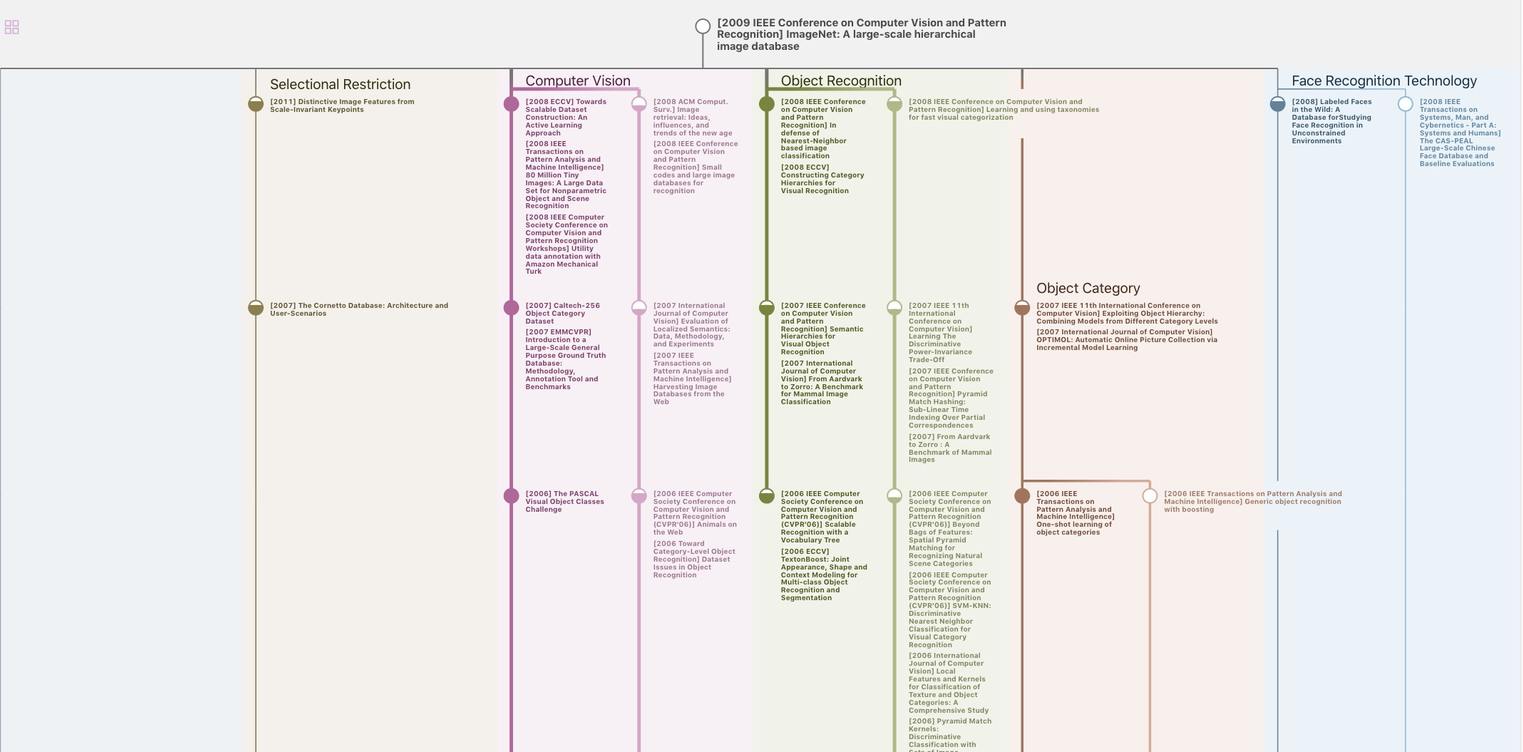
生成溯源树,研究论文发展脉络
Chat Paper
正在生成论文摘要