Pseudo-kernel method in U-statistic variance estimation with large kernel size
STATISTICA SINICA(2018)
摘要
This paper addresses the problem of variance estimation of a general U statistic with large kernel size (degree) k. U-statistics form a class of unbiased estimators. It was first proposed in Hoeffding (1948) and has since been widely used in many statistical applications. Wang and Lindsay (2014) propose an unbiased variance estimator for a general U-statistic; it is applicable provided that the kernel size k is at most half of the sample size n. This condition restricts its application to common K-fold cross-validation problems. We devise a pseudo-kernel variance estimator that can be realized in the same fashion as the unbiased variance estimator, but is defined based on a pseudo-kernel function of degree two. We demonstrate how to construct a pseudo-kernel function and show that the resulting variance estimator is second-order unbiased. Moreover, we develop an efficient realization of the proposal in the context of K-fold cross-validation. The proposed variance estimator shows comparable performance with significantly improved computational efficiency compared to its bootstrap and jackknife counterparts in simulation and data analysis in the context of model selection using the "one-standard-error" rule.
更多查看译文
关键词
K-fold cross-validation,Kullback-Leibler distance,pseudo kernel,second-order unbiased,U-statistic,variance estimation
AI 理解论文
溯源树
样例
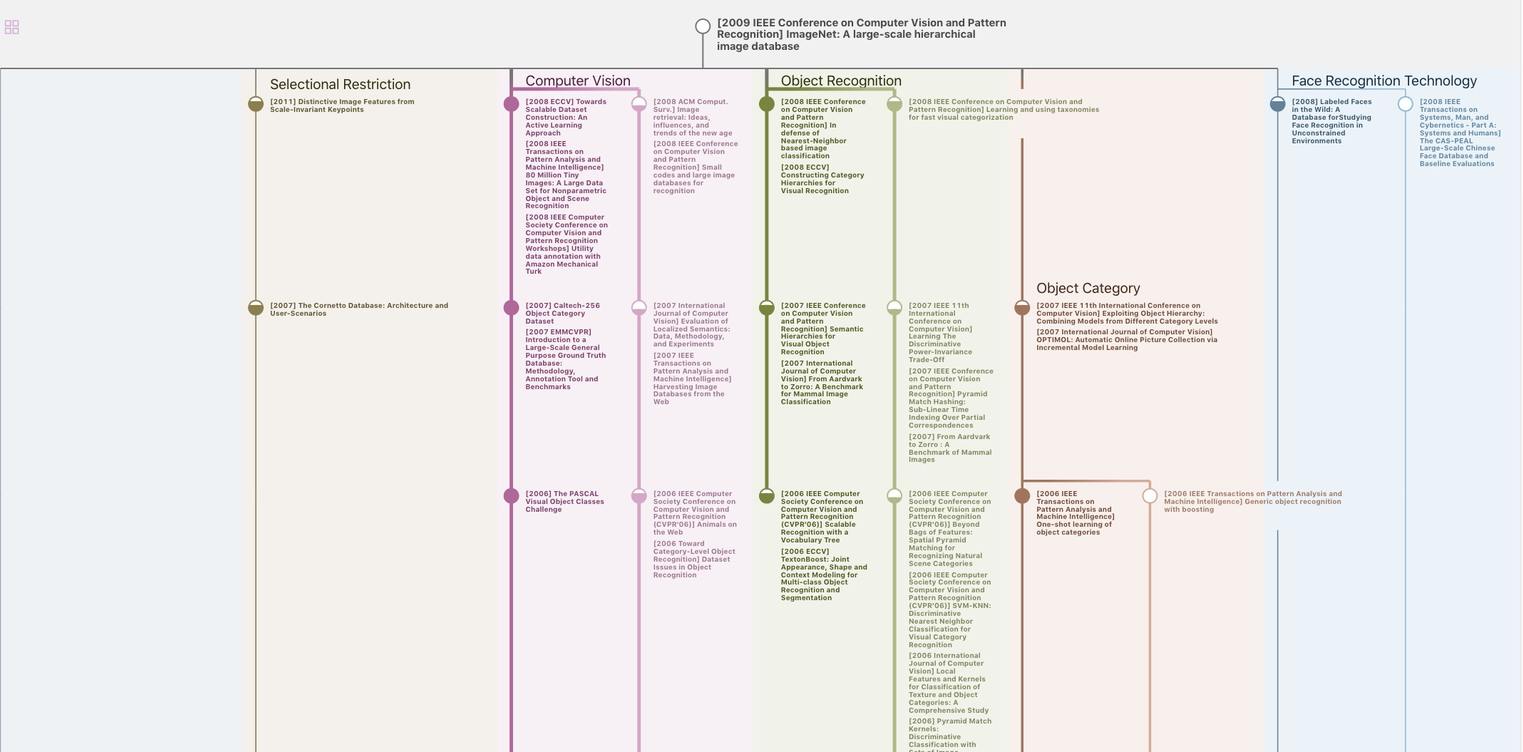
生成溯源树,研究论文发展脉络
Chat Paper
正在生成论文摘要