Partial volume effect and noise compensation for improved Dose Volume Histogram in 90Y-SIRT PET based dosimetry
JOURNAL OF NUCLEAR MEDICINE(2016)
Abstract
1013 Objectives Dose Volume Histograms (DVH) for 90Y-SIRT PET based dosimetry are impacted by partial volume effect (PVE) and noise. In this work we compare several PVE compensations (PVC) and the effect of noise removal on the DVH. Methods A 1-hour acquisition of the NEMA94 phantom with a Biograph mCT (Siemens) PET system was simulated using GATE V7.1 and reconstructed using Siemens reconstruction software (VG50, 3 iterations, 24 subsets, PSF). The phantom consisted of an hot cylinder (45mm ∅, 18cm long) of water with 3256MBq of 90Y within a cylinder (20 cm long, 20 cm ∅) of water devoid of activity. The absorbed-dose distribution was computed using dose-kernel convolution. The spatial resolution was determined from the measured activity recovery coefficient of the cylinder on the reconstructed images. Two PVC algorithms were implemented on the reconstructed images: • Geometric transfer matrix (GTM), assuming that the activity distribution is piecewise constant (Rousset et al, JNM 1998) • Region based voxel-wise (RBV) correction at the voxel level (Thomas et al, EJNMMI 2011). Additionally, we investigated a local deposition approach (LED), where the energy spread from electron transport is assumed to be similar to the image degradation due to spatial resolution. To reduce the effect of noise, a Gaussian pre-filtering of the reconstructed images prior to LED was also tested. Finally, a MLEM deconvolution of the probability density function (PDF) of noise was applied to the DVHs obtained from RBV and LED. The PDF was determined from the histogram of the inner part of the hot cylinder (inner 17mm), where the dose is assumed to be uniform. The DVH resulting from each approach was compared to the true DVH in terms of deviations of the D2%, D50% and D80% dosimetric indexes. Results The spatial resolution FWHM was 5 mm. The noise PDF was a lognormal distribution. The deviations ΔD2%, ΔD50% and ΔD80% were 43.9%, -13.8% and -18.6% when no correction was applied; -0.7%, 0.2% and 4.5% for GTM; 51.9%, -9.5% and -12.1% for RBV; 179%, -26.6% and -63.4% for LED and 13.3%, -9.3% and -11.1% for LED with Gaussian prefiltering. When noise deconvolution was applied, the deviations ΔD2%, ΔD50% and ΔD80% were 24.0%, -8.3% and 7.1% for LED and -12.8%, -5.0% and 2.4% for RBV. GTM provided the best agreement, as expected since the test phantom obeys the assumptions of GTM, and it is quite insensitive to noise because it only estimates the mean activity in each region. LED does not make any prior assumptions about the activity distribution but showed results worse than performing no PVE correction at all, due to the effect of noise. Adding a Gaussian prefiltering prior to LED reduces noise at the expense of spatial resolution. RBV, working at the voxel level, should not be as sensitive as GTM to the quality of the segmentation but it is affected by noise. In all cases, knowing the noise PDF allows for partial noise deconvolution of the DVH, improving results. The best results among the voxel based methods were found for the combination RBV + noise deconvolution. Conclusions PVC improves DVHs. Noise has a smoothing effect on DVHs because it introduces a spread of PVC improves DVHs. Noise has a smoothing effect on DVHs because it introduces a spread of absorbed dose values, impacting LED and in to lesser degree RBV. In both cases, PDF deconvolution improved the results.
MoreTranslated text
Key words
dose volume histogram,dosimetry,partial volume effect,noise compensation,y-sirt
AI Read Science
Must-Reading Tree
Example
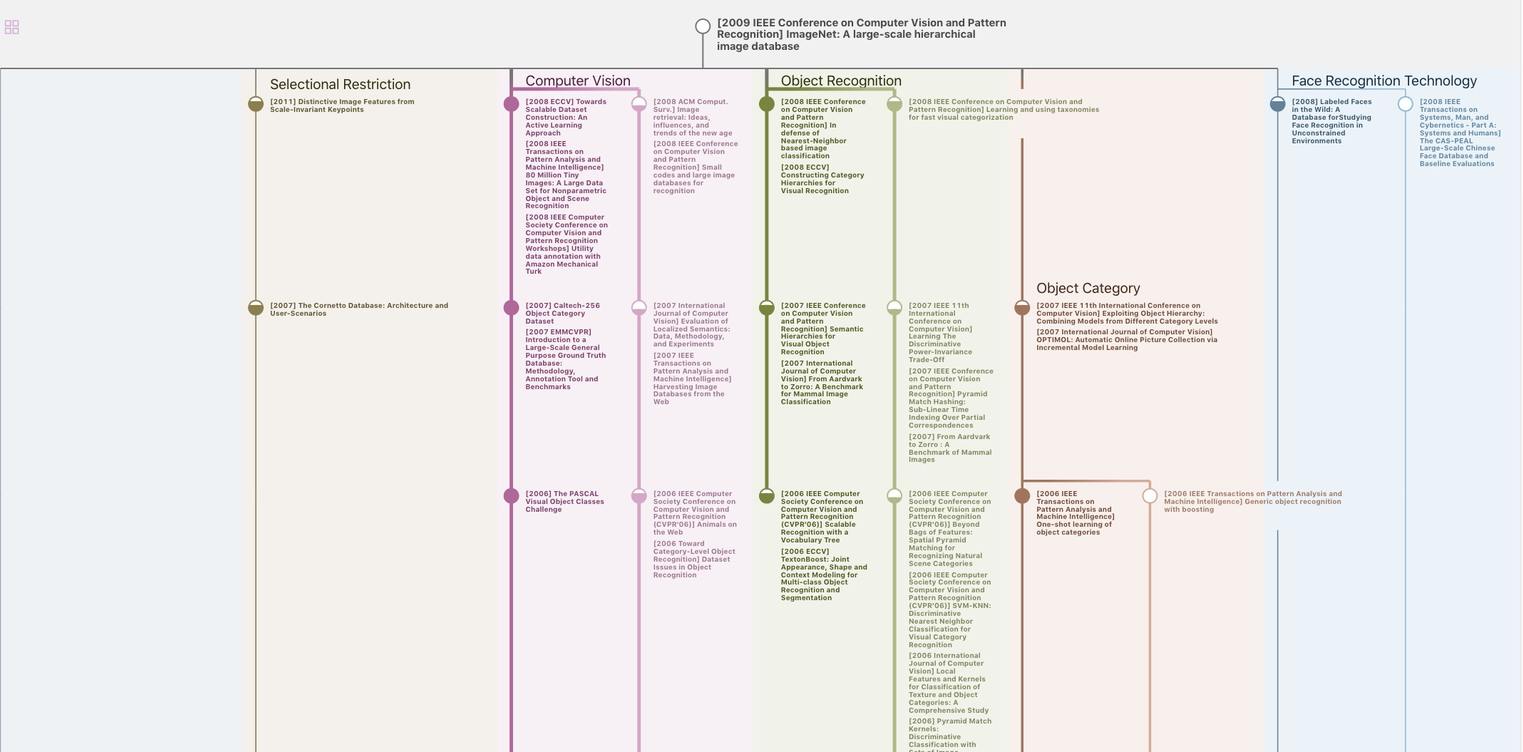
Generate MRT to find the research sequence of this paper
Chat Paper
Summary is being generated by the instructions you defined