CONVERGENCE OF EIGENVECTOR EMPIRICAL SPECTRAL DISTRIBUTION OF SAMPLE COVARIANCE MATRICES
ANNALS OF STATISTICS(2020)
摘要
The eigenvector empirical spectral distribution (VESD) is a useful tool in studying the limiting behavior of eigenvalues and eigenvectors of covariance matrices. In this paper, we study the convergence rate of the VESD of sample covariance matrices to the deformed Marcenko-Pastur (MP) distribution. Consider sample covariance matrices of the form Sigma(XX)-X-1/2* Sigma(1/2), where X = (x(ij)) is an M x N random matrix whose entries are independent random variables with mean zero and variance N-1, and Sigma is a deterministic positive-definite matrix. We prove that the Kolmogorov distance between the expected VESD and the deformed MP distribution is bounded by N-1+epsilon for any fixed epsilon > 0, provided that the entries root Nx(ij) have uniformly bounded 6th moments and vertical bar N/M - 1 vertical bar >= tau for some constant tau > 0. This result improves the previous one obtained in (Ann. Statist. 41 (2013) 2572-2607), which gave the convergence rate O(N-1/2) assuming i.i.d. X entries, bounded 10th moment, Sigma = I and M < N. Moreover, we also prove that under the finite 8th moment assumption, the convergence rate of the VESD is O(N-1/2+epsilon) almost surely for any fixed epsilon > 0, which improves the previous bound N-1/4+epsilon in (Ann. Statist. 41 (2013) 2572-2607).
更多查看译文
关键词
Sample covariance matrix,empirical spectral distribution,eigenvector empirical spectral distribution,Marcenko-Pastur distribution
AI 理解论文
溯源树
样例
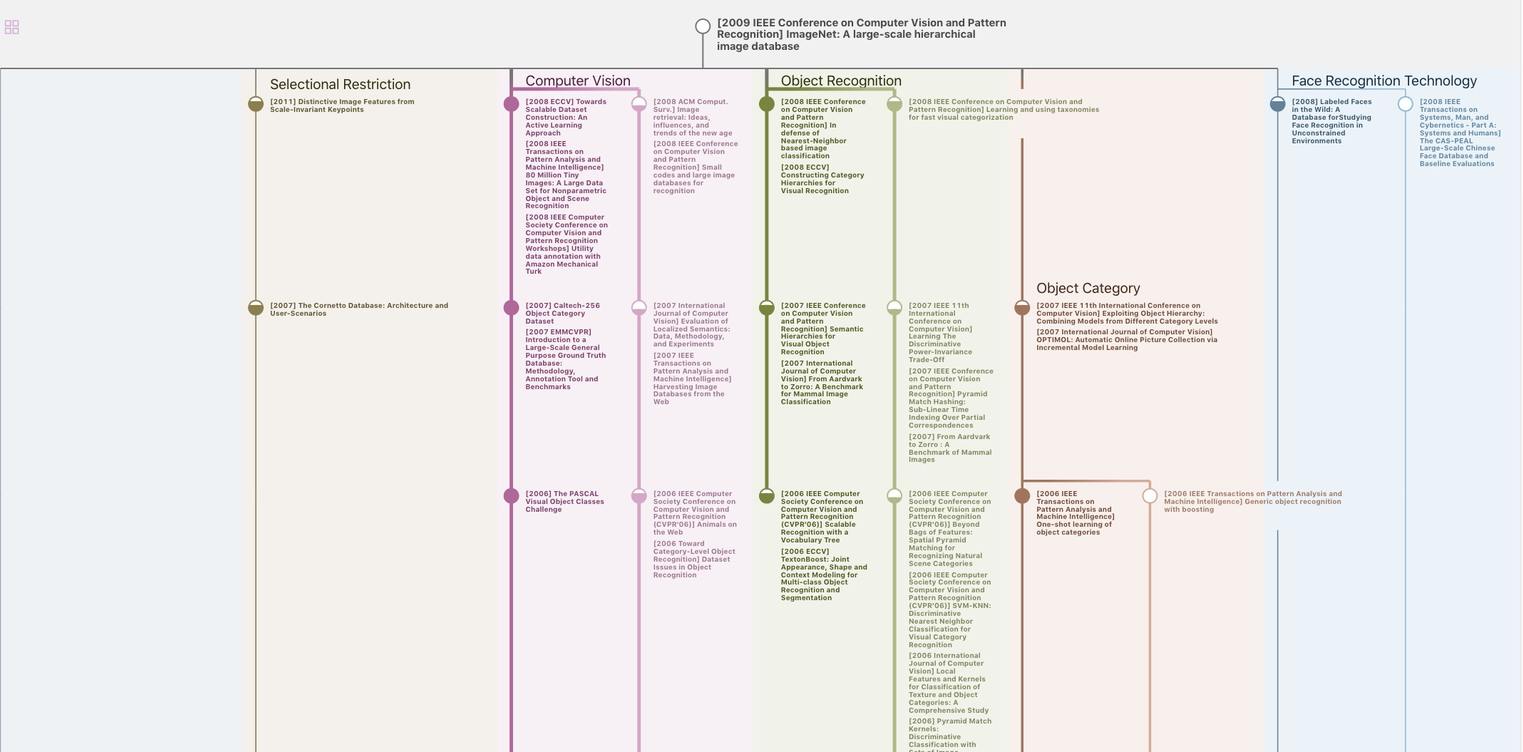
生成溯源树,研究论文发展脉络
Chat Paper
正在生成论文摘要