Robust state estimation for stochastic complex-valued neural networks with sampled-data
Neural Computing and Applications(2017)
摘要
In this paper, the robust state estimation problem is investigated for the complex-valued neural networks involving parameter uncertainties, mixed time delays, as well as stochastic disturbances by resorting to the sampled-data information from the available output measurements. The parameter uncertainties are assumed to be norm-bounded and the stochastic disturbances are assumed to be Brownian motions, which could reflect much more realistic dynamical behaviors of the complex-valued network under a noisy environment. The purpose of the addressed problem is to design an estimator for the complex-valued network such that, for all admissible parameter uncertainties and sampled output measurements, the dynamics of the state estimation error system is assured to be globally asymptotically stable in the mean square. Matrix inequality approach, robust analysis tool, as well as stochastic analysis techniques are utilized together to derive several delay-dependent sufficient criteria guaranteeing the existence of the desired state estimator. Finally, simulation examples are illustrated to demonstrate the feasibility of the proposed estimation design schemes.
更多查看译文
关键词
Complex-valued neural networks,State estimation,Parameter uncertainties,Sampled-data,Stochastic disturbance
AI 理解论文
溯源树
样例
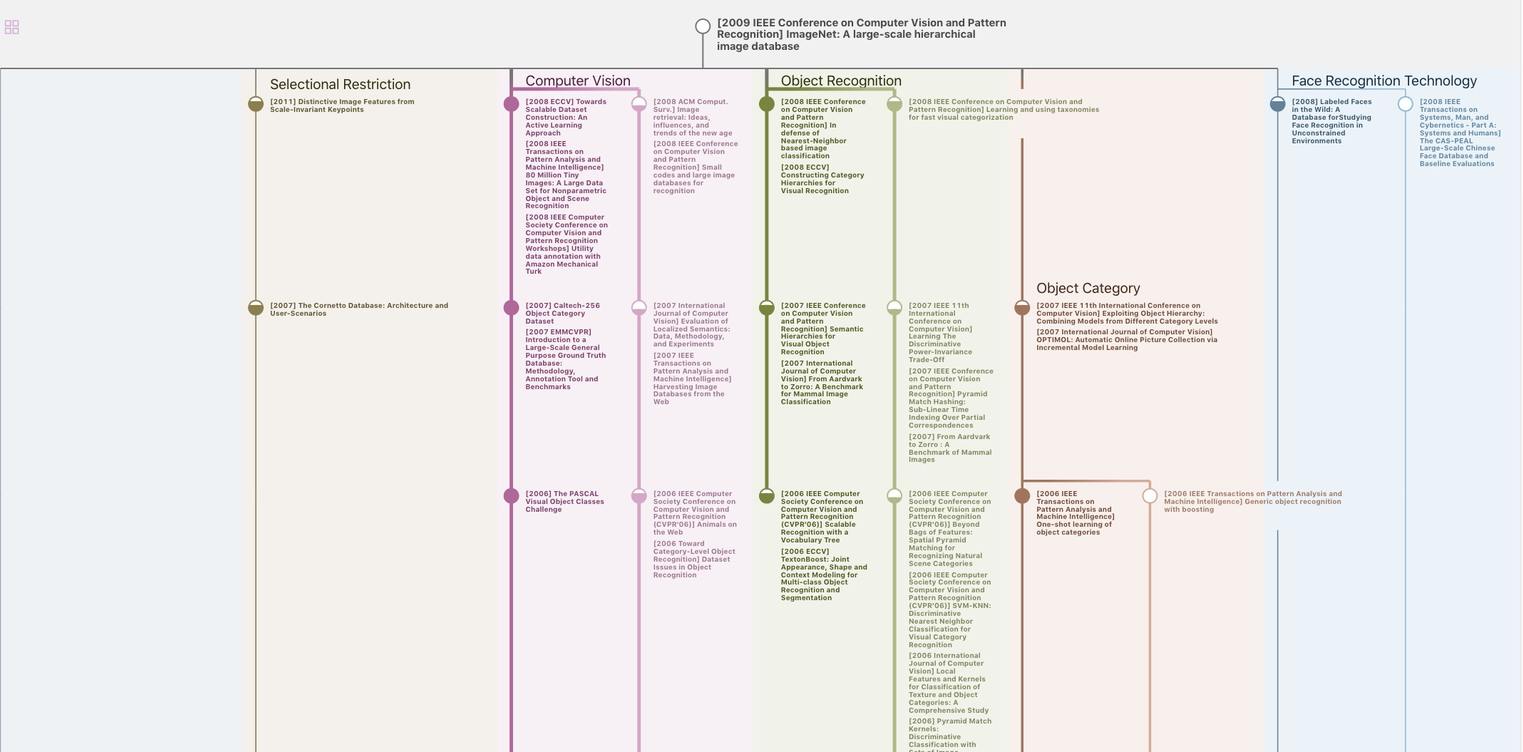
生成溯源树,研究论文发展脉络
Chat Paper
正在生成论文摘要