Experimental Demonstration of Feature Extraction and Dimensionality Reduction Using Memristor Networks
NANO LETTERS(2017)
摘要
Memristors have been considered as a leading candidate for a number of critical applications ranging from nonvolatile memory to non-Von Neumann computing systems. Feature extraction, which aims to transform input data from a high-dimensional space to a space with fewer dimensions, is an important technique widely used in machine learning and pattern recognition applications. Here, we experimentally demonstrate that memristor arrays can be used to perform principal component analysis, one of the most commonly used feature extraction techniques, through online, unsupervised learning. Using Sangers rule, that is, the generalized Hebbian algorithm, the principal components were obtained as the memristor conductances in the network after training. The network was then used to analyze sensory data from a standard breast cancer screening database with high classification success rate (97.1%).
更多查看译文
关键词
Unsupervised learning,principal component analysis,clustering,neuromorphic computing,artificial neural network,RRAM
AI 理解论文
溯源树
样例
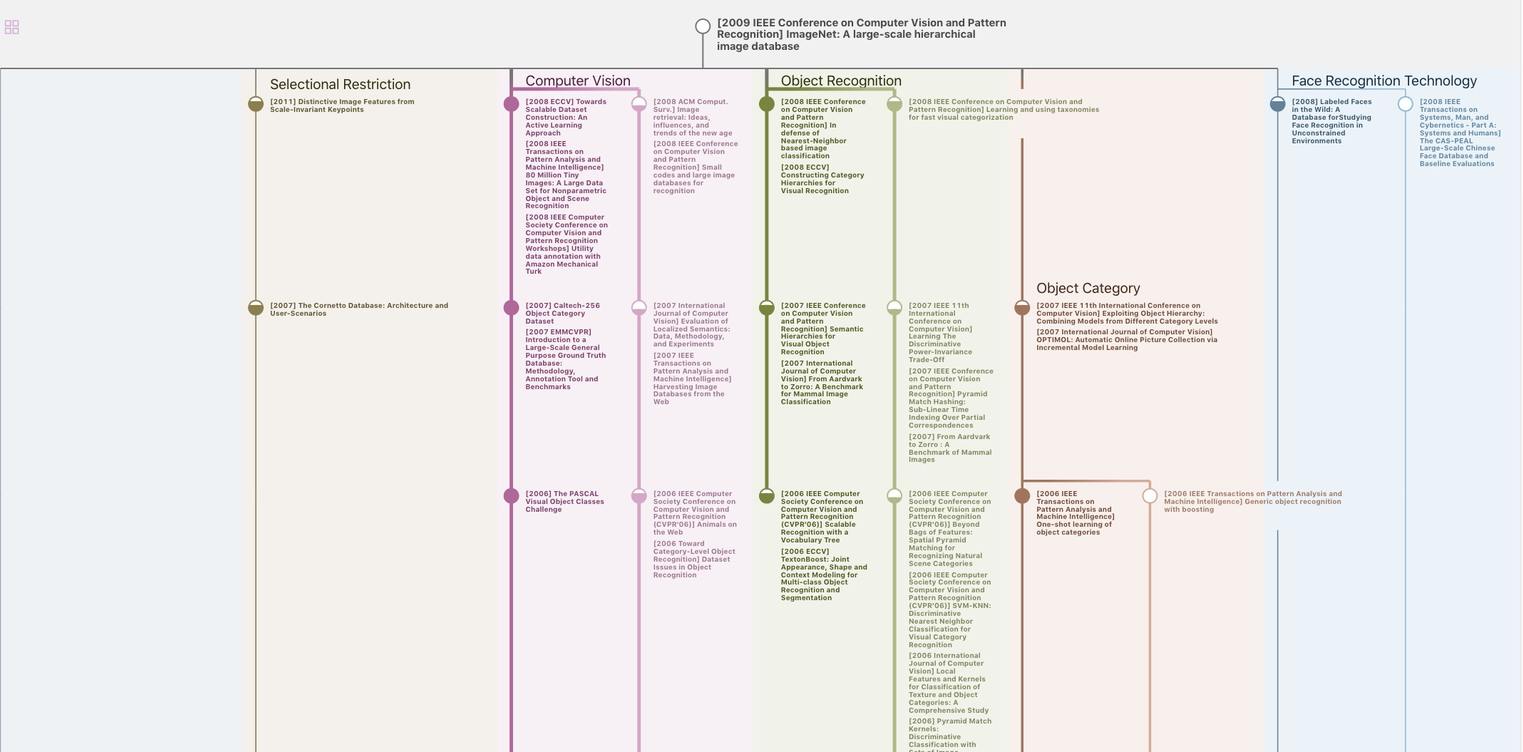
生成溯源树,研究论文发展脉络
Chat Paper
正在生成论文摘要