0110 AUTOMATED SLEEP STAGING USING BIG DATA FROM A CLINICAL SLEEP LABORATORY
Sleep(2017)
Abstract
Automated sleep staging has been previously limited by a combination of clinical heterogeneity and relatively limited sample sizes. We investigate the extent to which machine learning methods can utilize electroencephalogram features to approximate the performance of human experts when supplied with sufficient numbers of training cases, and to investigate how staging performance depends on the number of training nights, contextual information, model complexity, and imbalance between sleep stage proportions. We analyzed full-night recordings from 2000 diagnostic studies separated into training (n=1000) and testing (n=1000) sets. Extreme learning machine (ELM) algorithm was based on features extracted from six EEG leads. A hidden Markov model was then applied to take into account contextual information. Cohen’s Kappa statistic was used to evaluate agreement between algorithm output and human scoring. Staging performance improved with increasing numbers of training nights until saturation occurs (exceeding ~n=300) cases, beyond which point testing performance largely saturated. Performance was improved by accounting for contextual information, increasing model complexity, and adjusting the model training procedure to account for stage imbalance of stage percentage. The final testing performance was 0.68 as measured by Cohen’s kappa. Automated staging was less accurate for epochs at stage transition points. Training with a large dataset enables automated sleep staging that compares favorably with manual scoring by human-experts. Staging near transitions was less accurate, suggesting that automated algorithm performance may vary depending on the degree of pathophysiology present. Because testing was performed on a large and heterogeneous dataset, it is likely that these results will generalize broadly. This study was funded by Nanyang Technological University Research Scholarship.
MoreTranslated text
Key words
automated sleep staging,sleep laboratory,big data
AI Read Science
Must-Reading Tree
Example
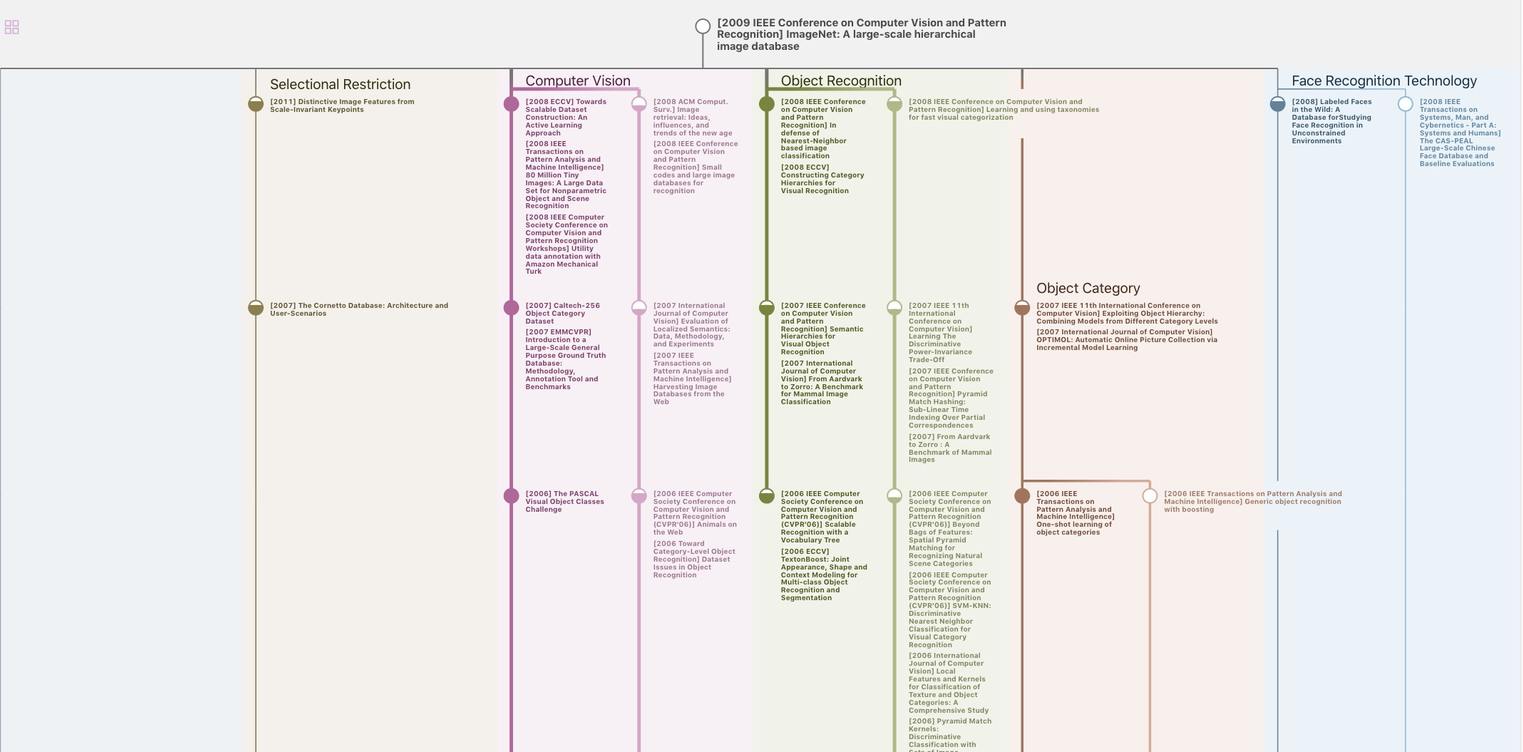
Generate MRT to find the research sequence of this paper
Chat Paper
Summary is being generated by the instructions you defined