Unilaterally Aggregated Contrastive Learning with Hierarchical Augmentation for Anomaly Detection
2023 IEEE/CVF International Conference on Computer Vision (ICCV)(2023)
摘要
Anomaly detection (AD), aiming to find samples that deviate from the training distribution, is essential in safety-critical applications. Though recent self-supervised learning based attempts achieve promising results by creating virtual outliers, their training objectives are less faithful to AD which requires a concentrated inlier distribution as well as a dispersive outlier distribution. In this paper, we propose Unilaterally Aggregated Contrastive Learning with Hierarchical Augmentation (UniCon-HA), taking into account both the requirements above. Specifically, we explicitly encourage the concentration of inliers and the dispersion of virtual outliers via supervised and unsupervised contrastive losses, respectively. Considering that standard contrastive data augmentation for generating positive views may induce outliers, we additionally introduce a soft mechanism to re-weight each augmented inlier according to its deviation from the inlier distribution, to ensure a purified concentration. Moreover, to prompt a higher concentration, inspired by curriculum learning, we adopt an easy-to-hard hierarchical augmentation strategy and perform contrastive aggregation at different depths of the network based on the strengths of data augmentation. Our method is evaluated under three AD settings including unlabeled one-class, unlabeled multi-class, and labeled multi-class, demonstrating its consistent superiority over other competitors.
更多查看译文
关键词
aggregated contrastive learning,anomaly detection,augmentation
AI 理解论文
溯源树
样例
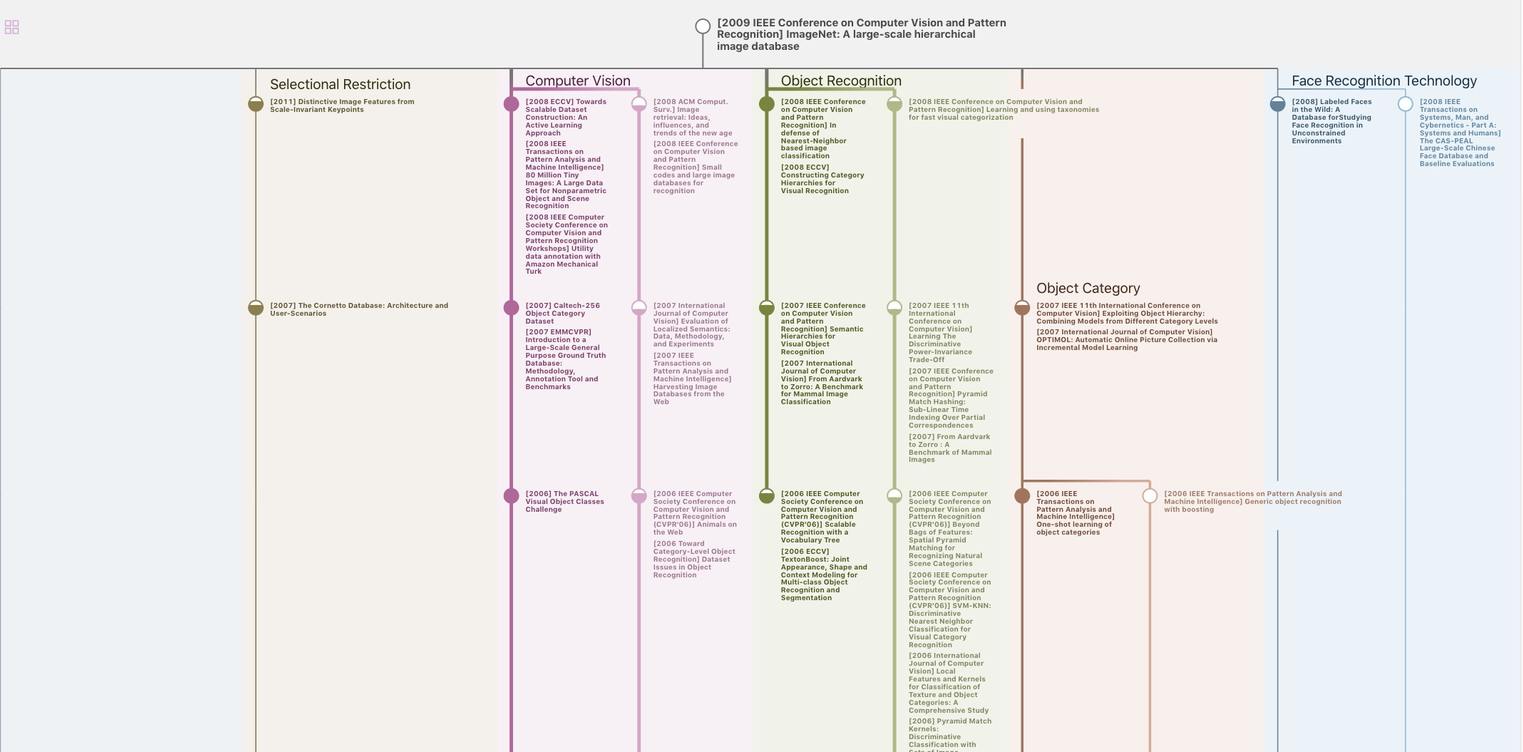
生成溯源树,研究论文发展脉络
Chat Paper
正在生成论文摘要