AUTOMATED APPROACH TO PREDICTING MALIGNANT TRANSFORMATIONS IN RECURRENT LOW-GRADE GLIOMAS USING EX VIVO SPECTROSCOPIC DATA
Neuro-oncology(2016)
Abstract
To automatically classify recurrent low-grade gliomas (LGGs) that remain grade II versus those that have transformation to a higher grade (grade III or IV) by applying machine learning algorithms on ex vivo nuclear magnetic resonance (NMR) data, acquired from biopsy samples of LGG patients. Multivariate statistical models were used to identify regions in the spectra that were characteristic for each group and to obtain the metabolic signatures of both types of LGGs. Due to the high-dimensionality of spectroscopic data, we utilized orthogonal partial least squares discriminant analysis (OPLS-DA) to build the predictive model. This is a supervised learning algorithm well suited for the analysis of data where the number of observations is much smaller than the number of variables. The data comprised high-resolution magic angle spectroscopy (HRMAS) spectra of 112 image-guided tissue samples from 56 patients with recurrent LGG. Part of each sample was examined by a board-certified pathologist to evaluate histological grade and the remaining portion was analyzed using HRMAS. 43 samples (22 patients) originated from gliomas that remained grade II, 52 samples from 26 patients transforming to grade III and 19 samples from 8 patients transforming to grade IV. An OPLS-DA model (R2x=88%, R2y=94%, Q2=61% and root mean squared error of estimation RMSEE = 0.1) was trained and validated using leave-one-out cross-validation, resulting in mean specificity of 0.84 and sensitivity of 0.88. The peak regions containing the highest amount of discriminatory information for distinguishing between LGGs that transformed to a higher grade and those remaining grade II corresponded to glucose, myo-inositol, hypotaurine, choline, glycerophosphocholine, glutathione, 2-hydroxyglutarate, alanine, lactate and lipid. Metabolite signatures of tissue samples from lesions that remained grade II versus those that transformed to a higher grade included several features that can be measured non-invasively using in vivo H1 magnetic resonance spectroscopy.
MoreTranslated text
Key words
Medical Image Analysis,MRI Segmentation,Image Segmentation,Deep Learning
AI Read Science
Must-Reading Tree
Example
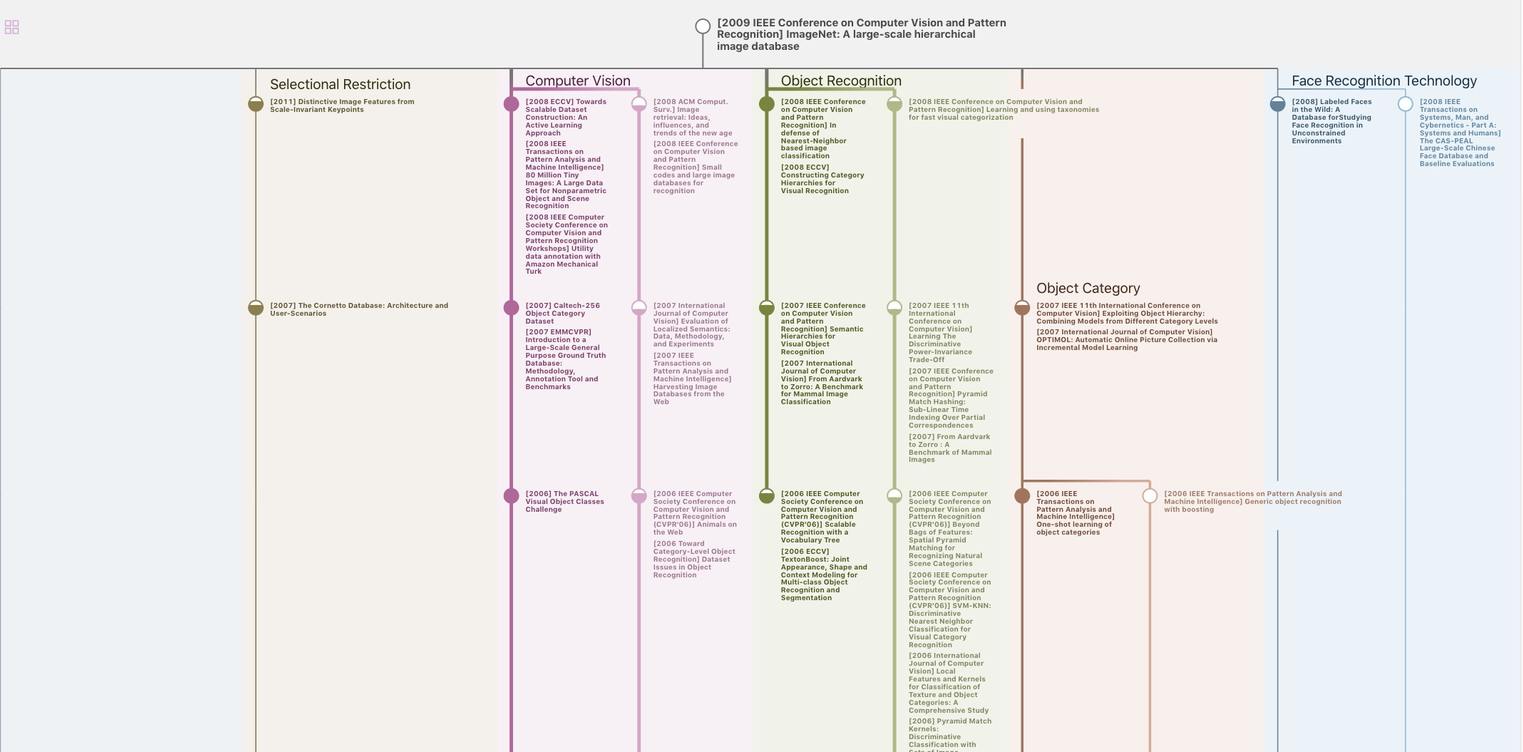
Generate MRT to find the research sequence of this paper
Chat Paper
Summary is being generated by the instructions you defined