Occupancy estimation with environmental sensing via non-iterative LRF feature learning in time and frequency domains
Energy and Buildings(2017)
摘要
Occupancy information is an important parameter to energy-efficient Air Conditioning and Mechanical Ventilation (ACMV) systems for the design of suitable control strategies. To predict the occupancy in an air-conditioned space, the harnessing of environmental parameters such as carbon dioxide, relative humidity, temperature and air pressure can provide an implicit and non-intrusive representation of occupancy in the room. Most of the prior works utilized the statistics of time domain features with feature selection, but the way to obtain suitable features based on raw sensor data still remains an issue. The niche of this paper is that a better representation of pattern of occupancy with respect to the environment can be learned automatically to enhance the estimation performance, rather than depending too much on human empirical knowledge in feature extraction. The prediction accuracy could be improved by using a non-iterative feature learning method, namely the Local Receptive Fields (LRF) with random weights. We find that raw data from the frequency domain together with time domain data which carries rich information for feature learning can enhance the performance of the estimation system. Experiments are evaluated in a research laboratory environment which consists of 24 cubicles with more than 20 occupants. Our experimental results demonstrated that feature learning with additional frequency domain data can significantly improve the occupancy estimation system.
更多查看译文
关键词
Occupancy prediction,Environmental sensing,Feature learning
AI 理解论文
溯源树
样例
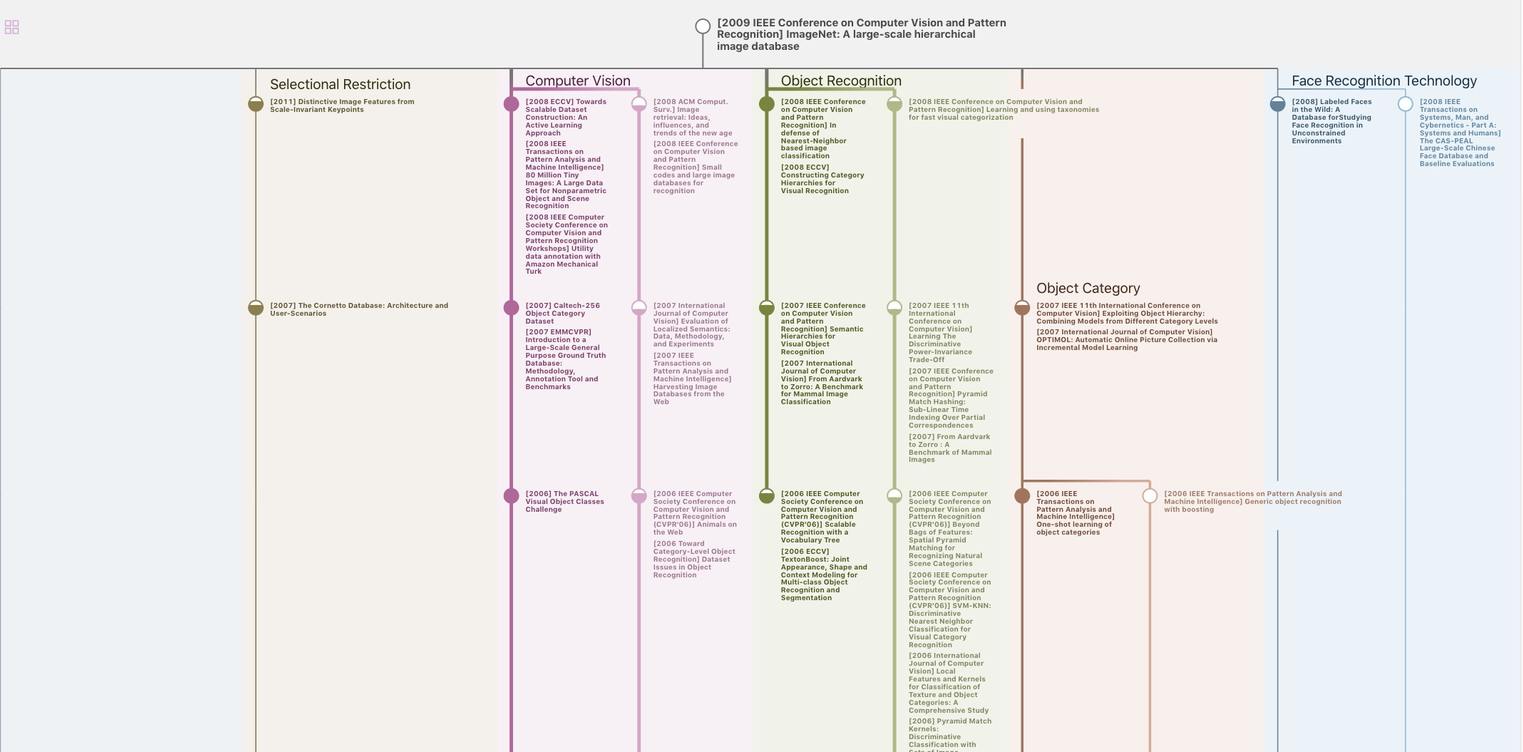
生成溯源树,研究论文发展脉络
Chat Paper
正在生成论文摘要