Online object tracking via bag-of-local-patches
Proceedings of SPIE(2017)
摘要
As one of the most important tasks in computer vision, online object tracking plays a critical role in numerous lines of research, which has drawn a lot of researchers' attention and be of many realistic applications. This paper develops a novel tracking algorithm based on the bag-of-local-patches representation with the discriminative learning scheme. In the first frame, a codebook is learned by applying the Kmeans algorithm to a set of densely sampled local patches of the tracked object, and then used to represent the template and candidate samples. During the tracking process, the similarities between the coding coefficients of the candidates and template are chosen as the likelihood values of these candidates. In addition, we propose effective model updating and discriminative learning schemes to capture the appearance change of the tracked object and incorporate the discriminative information to achieve a robust matching. Both qualitative and quantitative evaluations on some challenging image sequences demonstrate that the proposed tracker performs better than other state-of-the-art tracking methods.
更多查看译文
关键词
Object tracking,local patches,template matching,discriminative weight
AI 理解论文
溯源树
样例
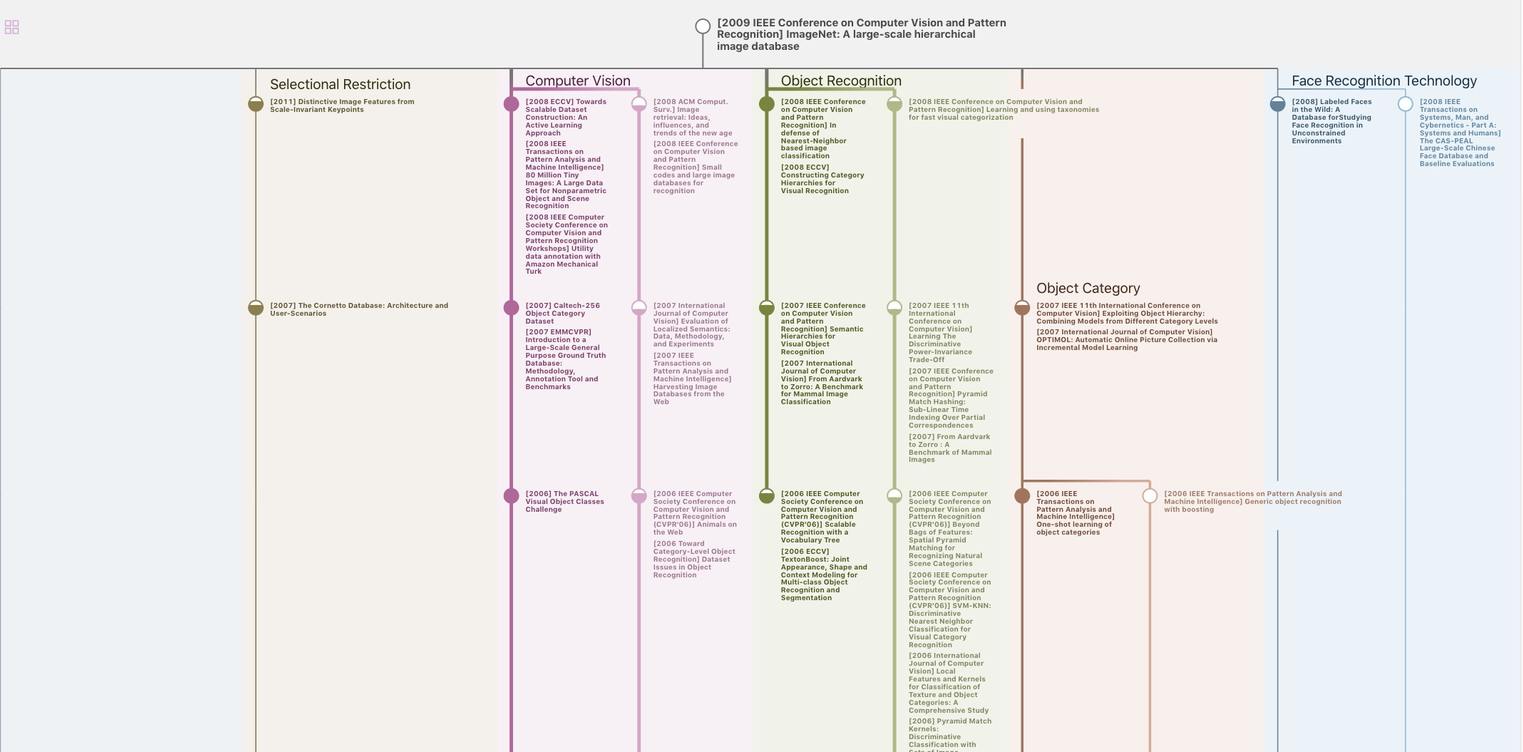
生成溯源树,研究论文发展脉络
Chat Paper
正在生成论文摘要