A general algorithm for computing simultaneous prediction intervals for the (log)-location-scale family of distributions
JOURNAL OF STATISTICAL COMPUTATION AND SIMULATION(2017)
摘要
Making predictions of future realized values of random variables based on currently available data is a frequent task in statistical applications. In some applications, the interest is to obtain a two-sided simultaneous prediction interval (SPI) to contain at least k out ofmfuture observations with a certain confidence level based on n previous observations from the same distribution. A closely related problem is to obtain a one-sided upper (or lower) simultaneous prediction bound (SPB) to exceed (or be exceeded) by at least k out ofmfuture observations. In this paper, we provide a general approach for computing SPIs and SPBs based on data from a particular member of the (log)-location-scale family of distributions with complete or right censored data. The proposed simulation-based procedure can provide exact coverage probability for complete and Type II censored data. For Type I censored data, our simulation results show that our procedure provides satisfactory results in small samples. We use three applications to illustrate the proposed simultaneous prediction intervals and bounds.
更多查看译文
关键词
Censored data,coverage probability,k out of m,lognormal,simulation,Weibull
AI 理解论文
溯源树
样例
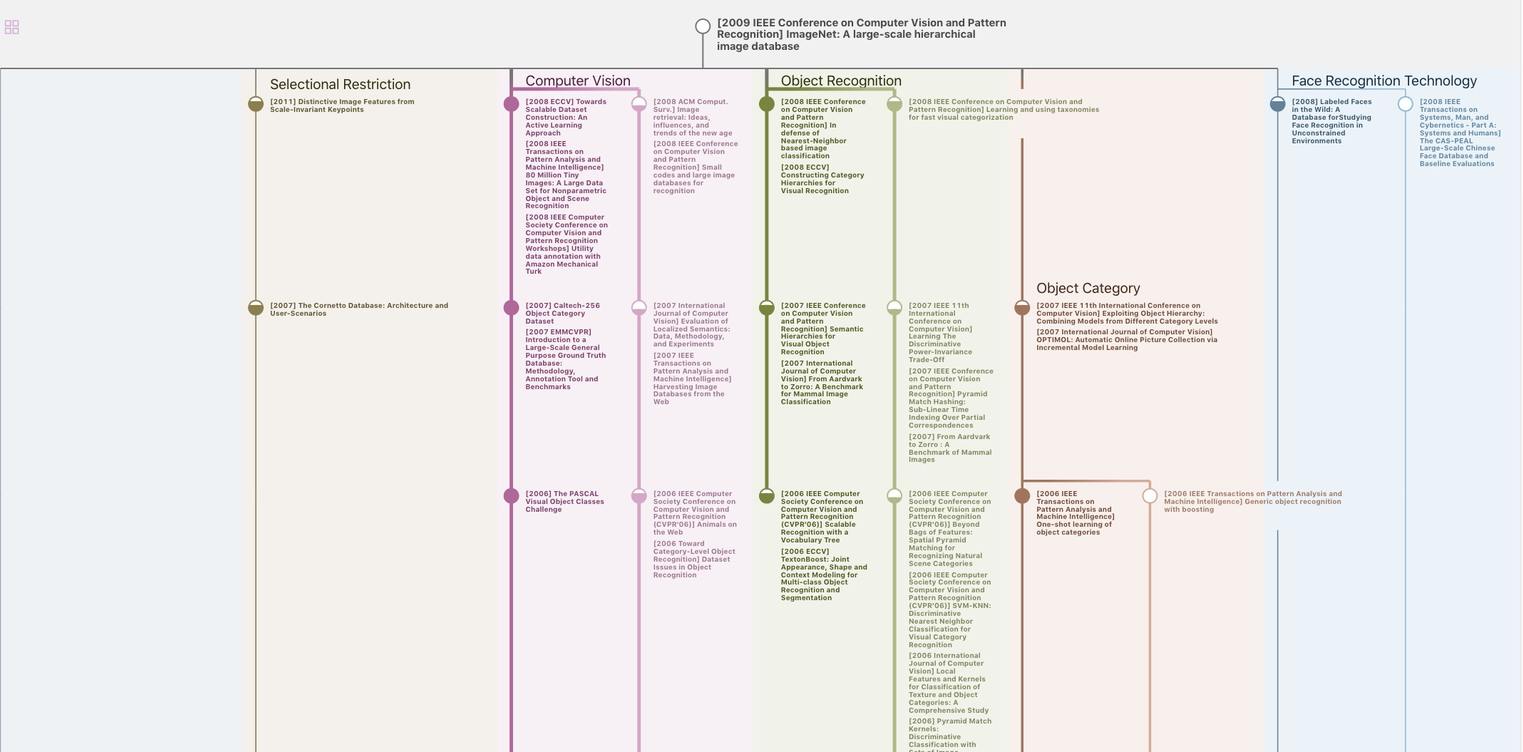
生成溯源树,研究论文发展脉络
Chat Paper
正在生成论文摘要