Simulation and uncertainty analysis for groundwater levels using radial basis function neural network and support vector machine models
JOURNAL OF WATER SUPPLY RESEARCH AND TECHNOLOGY-AQUA(2017)
摘要
Accurate measurement of groundwater levels is often difficult and involves great uncertainty. Therefore, simulating and predicting the fluctuating behavior of groundwater levels is necessary for water resource planning and management. In this study, radial basis function (RBF) neural networks and support vector machines (SVM) were employed to simulate groundwater level fluctuations. The time series data of precipitation, evaporation, and temperature were used as model inputs. Groundwater level data from the first 10 years, from 2003 to 2014, were used as the training dataset, while data from the last 2 years were used as the test dataset. Uncertainties caused by errors in the measurements of the variables or in outputs were estimated at 95% confidence intervals. The results showed that the SVM model had a superior simulation and prediction capability according to four statistical standards. The comparisons of the outputs and the confidence intervals of the two models showed that the SVM model was more accurate and had less uncertainty. The conclusions suggest that SVM is an effective method for simulating groundwater levels and analyzing model uncertainties using confidence intervals and can be used to facilitate sustainable groundwater management strategies.
更多查看译文
关键词
groundwater level,radial basis function neural networks,support vector machines,uncertainty analysis
AI 理解论文
溯源树
样例
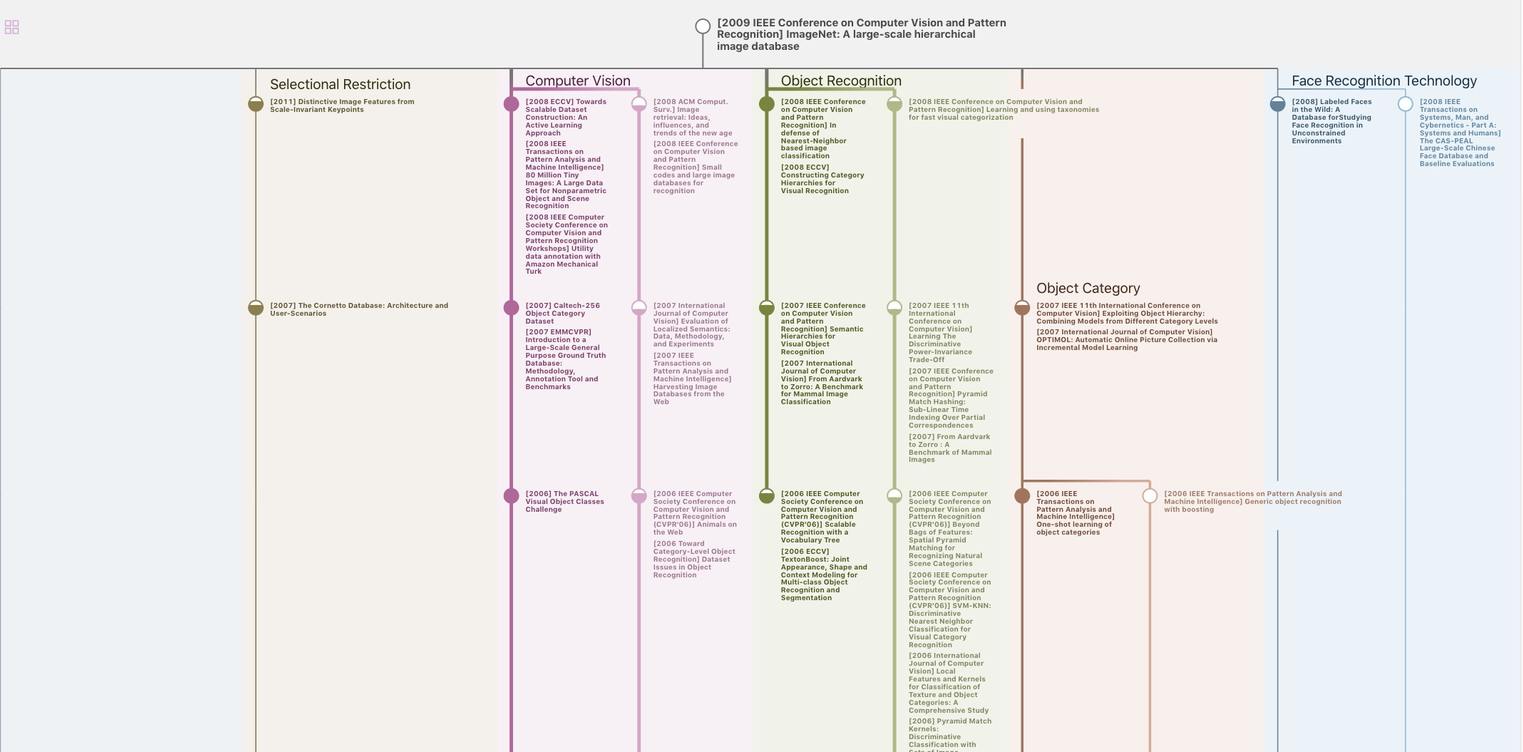
生成溯源树,研究论文发展脉络
Chat Paper
正在生成论文摘要