A Sparse Recovery Algorithm Based on Ratio of Stable Variables with Small Alpha
2016 8th International Conference on Intelligent Human-Machine Systems and Cybernetics (IHMSC)(2016)
摘要
In Compressed Sensing, recovery algorithms become poorer in efficiency when the problem dimension and signal sparsity increase. To reconstruct the large-scale sparse signal efficiently, a novel sparse recovery algorithm is proposed based on extremely heavy-tailed ratio of stable variables with small α. In the algorithm, the measurement matrix samples from a small α-stable distribution, which generates samples extremely close to the magnitude of each coordinate. Utilizing these samples, nonzero coordinates can be estimated exactly by a few iterations. Comparisons with BP algorithm and OMP algorithm demonstrate that the proposed algorithm could be faster in decoding speed, especially as the signal sparsity is not small. In addition, the reported experiments show that the recovery accuracy of our procedure is invariant for additional measurement noise.
更多查看译文
关键词
sparse recovery,stable distribution,ratio of stable variables,heavy-tailed
AI 理解论文
溯源树
样例
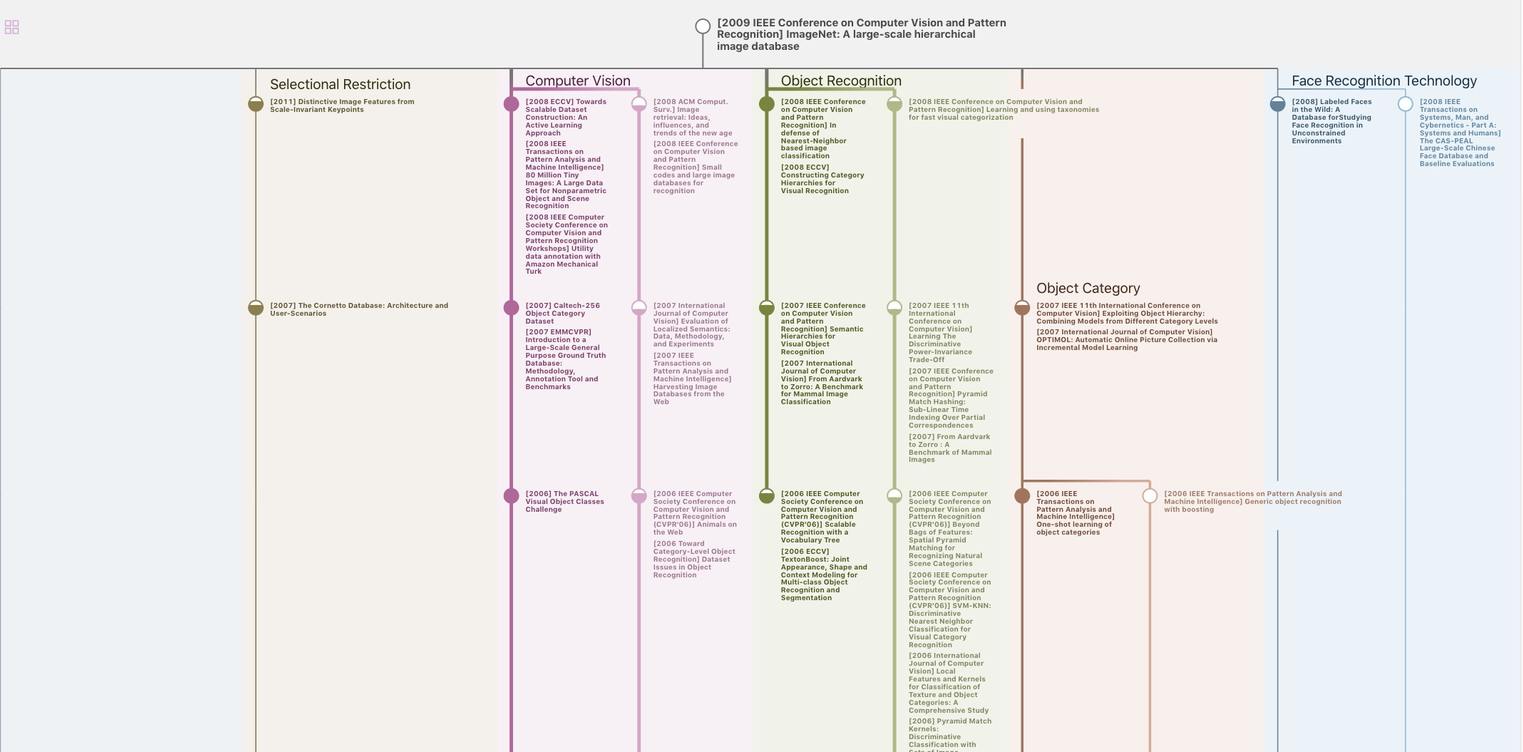
生成溯源树,研究论文发展脉络
Chat Paper
正在生成论文摘要