Partial discharge patterns recognition with deep Convolutional Neural Networks
2016 International Conference on Condition Monitoring and Diagnosis (CMD)(2016)
摘要
Traditional methods of partial discharge (PD) patterns recognition often rely on much prior knowledge about PD mechanism and signal processing techniques to construct appropriate features. Therefore the performance is not stable. Recent progress in deep neural networks, which contain more than one hidden layer, has shown state-of-art performance in speech recognition, image classification and natural language processing. Besides, the deep neural networks have the ability to handle large dataset, which is the technique for the future as more and more condition monitoring data accumulate. In this paper, a Convolutional Neural Network (CNN) with deep architecture is established to extrapolate new features automatically to realize ultra-high frequency (UHF) signals recognition in GIS. Firstly, a two-dimension spectral frames representation of the UHF signals is obtained by the time-frequency analysis. Then the spectral frames are used to train a deep CNN. It is shown that the proposed method can identify different sources of PD successfully. A comparison with other PD pattern recognition techniques is also discussed.
更多查看译文
关键词
partial discharge,Convolutional Neural Network,ultra-high frequency,pattern recognition
AI 理解论文
溯源树
样例
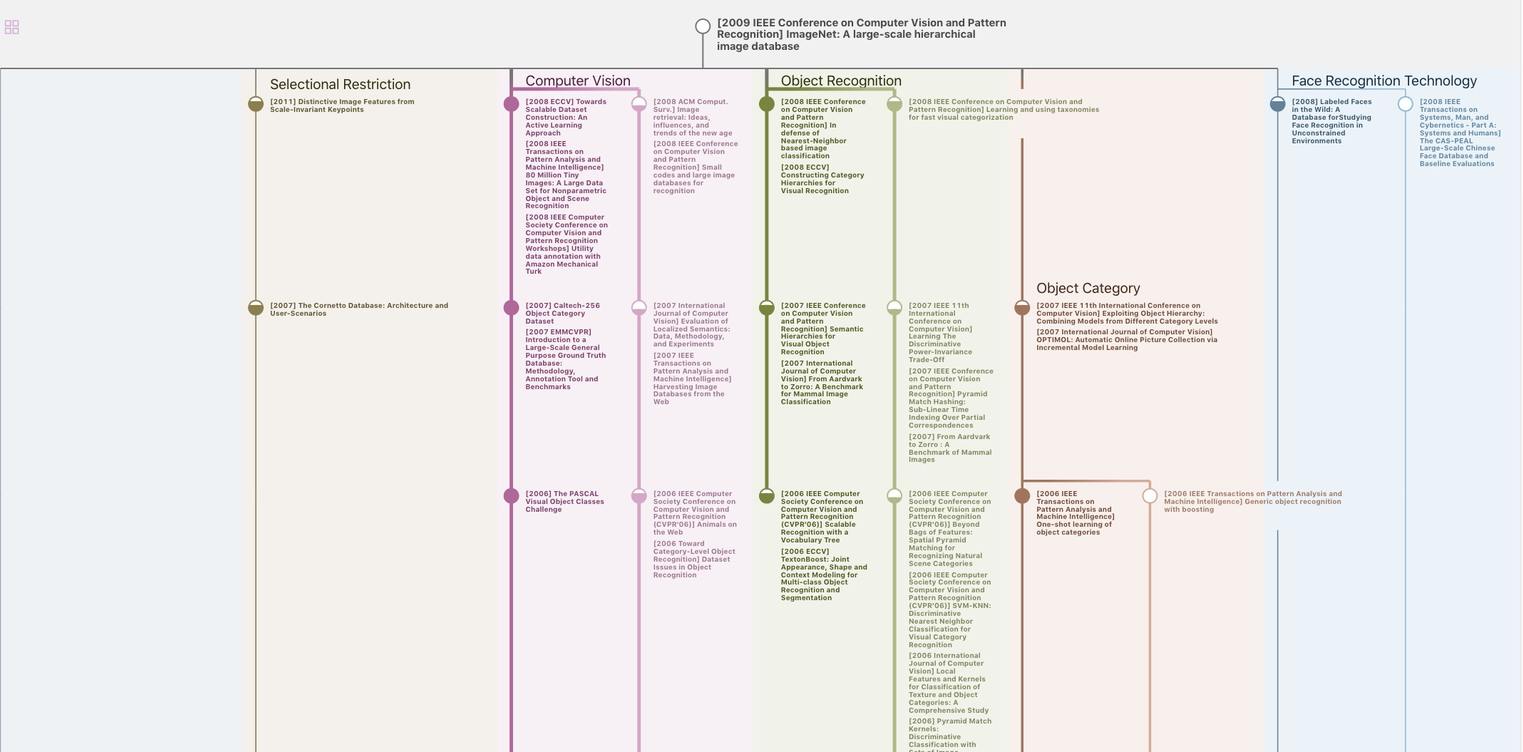
生成溯源树,研究论文发展脉络
Chat Paper
正在生成论文摘要