Quantification of Dockage in Canola Using a Flatbed Scanner
TRANSACTIONS OF THE ASABE(2013)
摘要
Various machine vision techniques have been applied to grade, size, and classify, different grain types such as wheat, rice, lentils, pulses, and soybeans. Little work has been done to grade canola using machine vision. The presence of dockage and conspicuous admixture affects the quality of canola. Therefore, classification of canola samples on the basis of total dockage (machine-separated dockage + conspicuous admixture) using flatbed scanners was outlined as the main objective for this study. Samples were prepared with varying amounts of total dockage: 0.1%, 0.3%, 1%, 1.34%, 1.45%, 2.5%, 3.4%, 4.3%, 4.6%, and 11.3%. This involved recording the intensity values of the luminosity (L), red (R), green (G), and blue (B) domains of sample images and analyzing the mean sample values by discriminant analysis using the Proc GLM and Proc Discrim procedures in SAS statistical software. The analysis showed a misclassification rate of 14%. The sample with 2.5% foreign material showed the maximum error during classification of samples. The sample with the maximum amount of total dockage (around 11.3%) was significantly different from all other samples. The overall misclassification rate decrease to 9.4% when the sample with 11.3% total dockage was removed from the analysis. Therefore, the presence of a sample with high total dockage content caused some bias in the analysis. It was concluded that the study showed some potential in discriminating between samples with different amounts of total dockage, but a model based on color information alone will not give accurate results. There is a need to include morphological and textural features to improve the accuracy of the model.
更多查看译文
关键词
Canola,Discriminant analysis,Dockage,Flatbed scanner,Grading,Machine vision
AI 理解论文
溯源树
样例
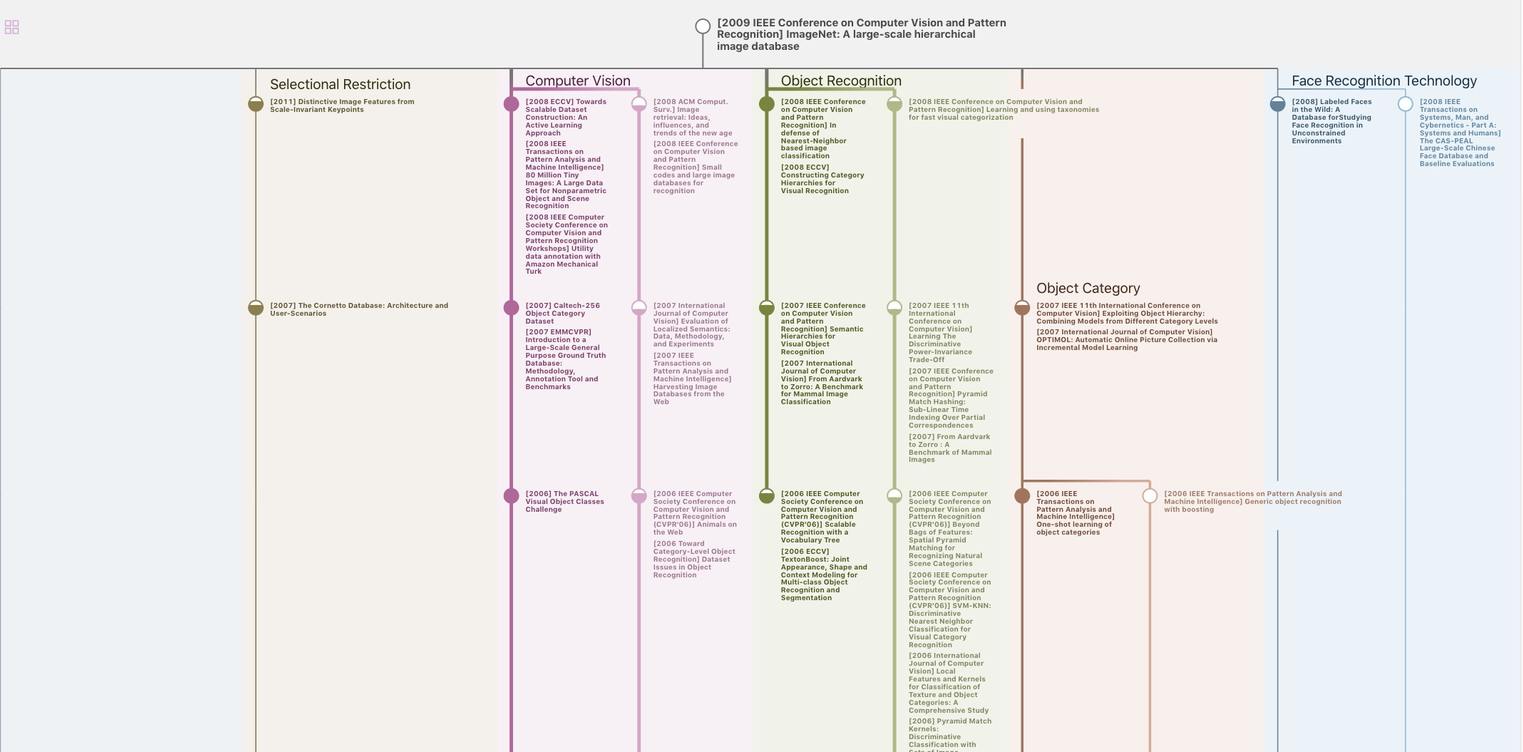
生成溯源树,研究论文发展脉络
Chat Paper
正在生成论文摘要