0920 Enrichment and Patterns of Comorbidities in the Electronic Health Record of Patients with diagnosed Obstructive Sleep Apnea
Sleep(2023)
Abstract
Abstract Introduction Obstructive Sleep Apnea (OSA) is the most common respiratory disorder of sleep and an independent risk factor for multiple other medical conditions related to poor quality of life and increased mortality and morbidity. The current study utilized the electronic health record (EHR) to examine relationships between OSA and common comorbidities within a large-scale dataset. Methods Cases with OSA and non-cases (controls) were defined using a validated EHR case identification algorithm, and matched based on age, sex, and observation period. We collapsed International Classification of Disease codes into PheCodes representing common etiologies and identified the top 20 most common comorbidities in the EHR among cases. We then compared overall and annual prevalence between matched cases and controls using conditional logistic regression. Latent class analysis (LCA) was used to identify subtypes of cases defined by specific combinations of comorbidities. Results Overall, 60,586 EHR-defined cases and 60,586 matched controls (from a total of 1,226,755 EHR-defined controls) were identified. Matched pairs had a median (IQR) age of 54 (45-63) years and 57.4% were males. BMI was higher in cases (median [IQR] = 35.9 [29.6, 41.0] kg/m2) than matched controls (28.0 [24.0, 32.0] kg/m2). Patients with OSA were at significantly greater risk of having each of the top PheCodes when compared to matched controls, with odds ratios ranging from 3.1 to 30.8 in the full matched set and 1.3 to 10.2 after controlling for BMI in the subset with available data. LCA identified 5 distinct subgroups based on comorbidities, labeled as High Comorbidity Burden, Cardiovascular Comorbidities, Depressive and Inflammatory Conditions, Low Comorbidity Burden, and Obesity and Cardiometabolic Dysregulation. Conclusion Our study demonstrates the power of leveraging the EHR to understand the relative health burden of OSA. In addition to showing enrichment for a number of comorbidities, we identified 5 subtypes defined by specific combinations of comorbidities in the EHR. These results could be informative for understanding likely disease outcomes and improved prevention and clinical care. Support (if any) National Research Service Awards (NRSA) T32 HL07713 National Institutes of Health (NIH) P01 HL094307
MoreTranslated text
Key words
obstructive sleep apnea,comorbidities,electronic health record
AI Read Science
Must-Reading Tree
Example
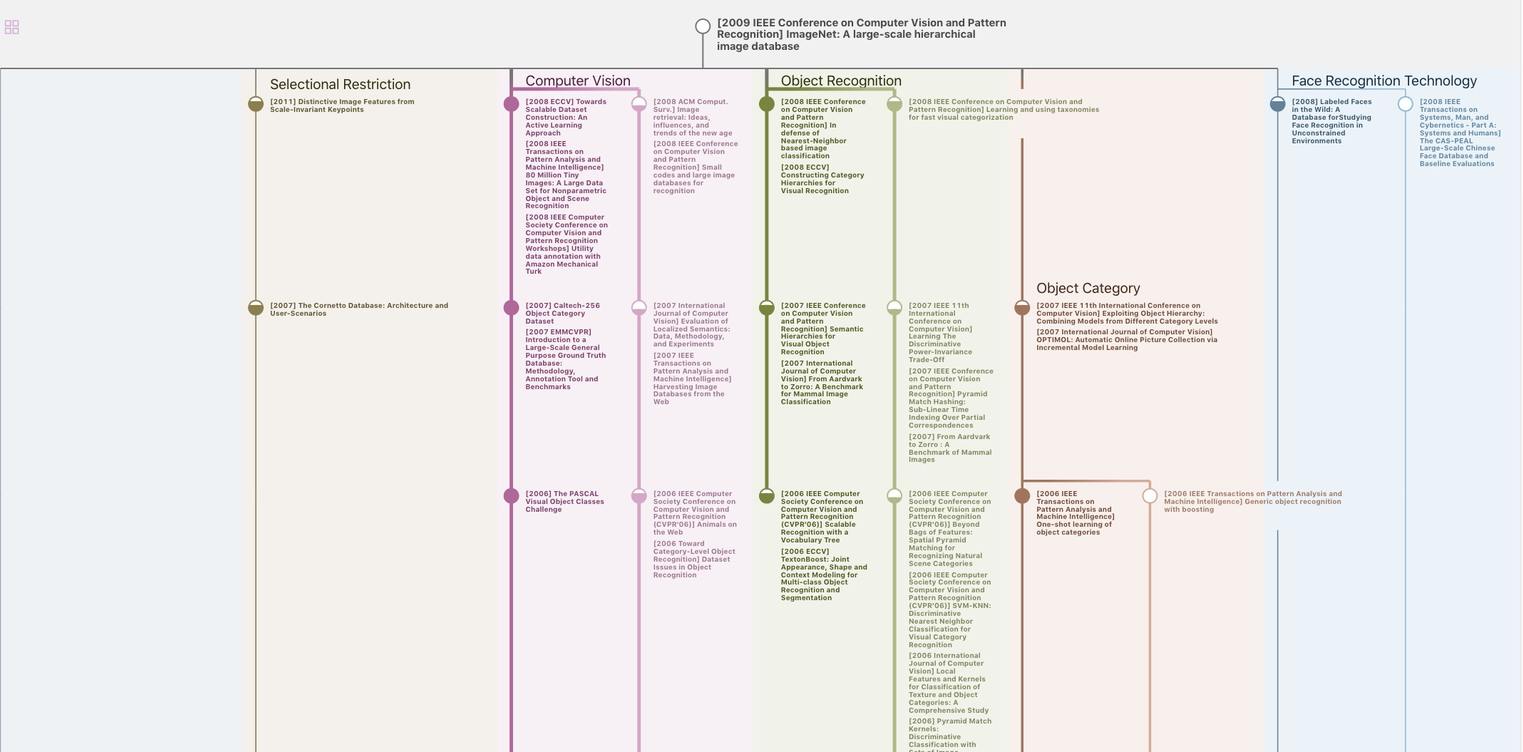
Generate MRT to find the research sequence of this paper
Chat Paper
Summary is being generated by the instructions you defined