The solution structure of the human complement regulator CFHR5 reveals a compact dimeric structure by X-ray scattering and analytical ultracentrifugation
IMMUNOBIOLOGY(2016)
摘要
Clinical genome sequencing (cGS) followed by orthogonal confirmatory testing is standard practice. While orthogonal testing significantly improves specificity, it also results in increased turnaround time and cost of testing. The purpose of this study is to evaluate machine learning models trained to identify false positive variants in cGS data to reduce the need for orthogonal testing.We sequenced five reference human genome samples characterized by the Genome in a Bottle Consortium (GIAB) and compared the results with an established set of variants for each genome referred to as a truth set. We then trained machine learning models to identify variants that were labeled as false positives.After training, the models identified 99.5% of the false positive heterozygous single-nucleotide variants (SNVs) and heterozygous insertions/deletions variants (indels) while reducing confirmatory testing of nonactionable, nonprimary SNVs by 85% and indels by 75%. Employing the algorithm in clinical practice reduced overall orthogonal testing using dideoxynucleotide (Sanger) sequencing by 71%.Our results indicate that a low false positive call rate can be maintained while significantly reducing the need for confirmatory testing. The framework that generated our models and results is publicly available at https://github.com/HudsonAlpha/STEVE.
更多查看译文
关键词
human complement regulator cfhr5,analytical ultracentrifugation,x-ray
AI 理解论文
溯源树
样例
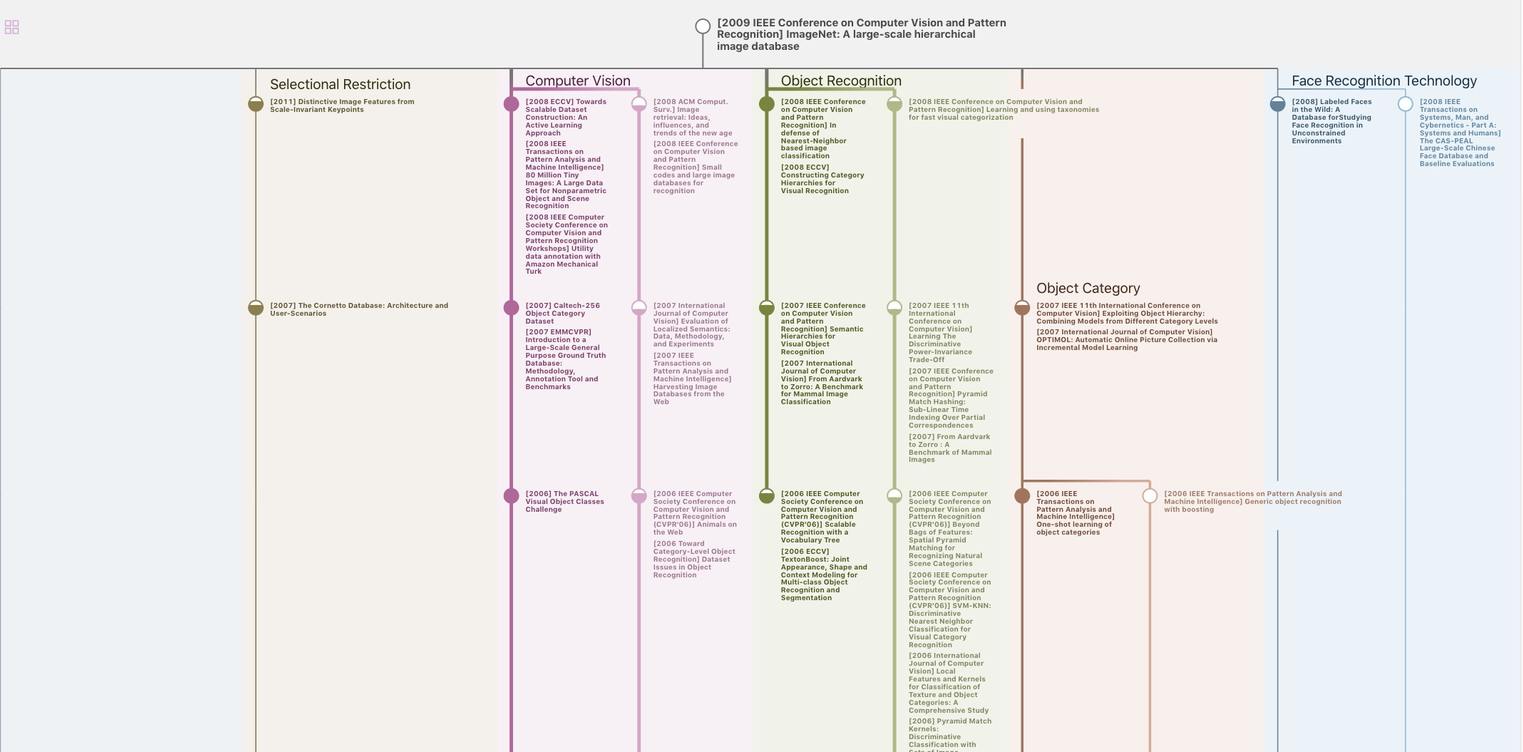
生成溯源树,研究论文发展脉络
Chat Paper
正在生成论文摘要