Nonlinear sensor fault diagnosis using mixture of probabilistic PCA models
Mechanical Systems and Signal Processing(2017)
Abstract
This paper presents a methodology for sensor fault diagnosis in nonlinear systems using a Mixture of Probabilistic Principal Component Analysis (MPPCA) models. This methodology separates the measurement space into several locally linear regions, each of which is associated with a Probabilistic PCA (PPCA) model. Using the transformation associated with each PPCA model, a parity relation scheme is used to construct a residual vector. Bayesian analysis of the residuals forms the basis for detection and isolation of sensor faults across the entire range of operation of the system. The resulting method is demonstrated in its application to sensor fault diagnosis of a fully instrumented HVAC system. The results show accurate detection of sensor faults under the assumption that a single sensor is faulty.
MoreTranslated text
Key words
Fault diagnosis,Sensor fault detection,Principal Component Analysis,Mixture of Probabilistic PCA,Nonlinear fault detection
AI Read Science
Must-Reading Tree
Example
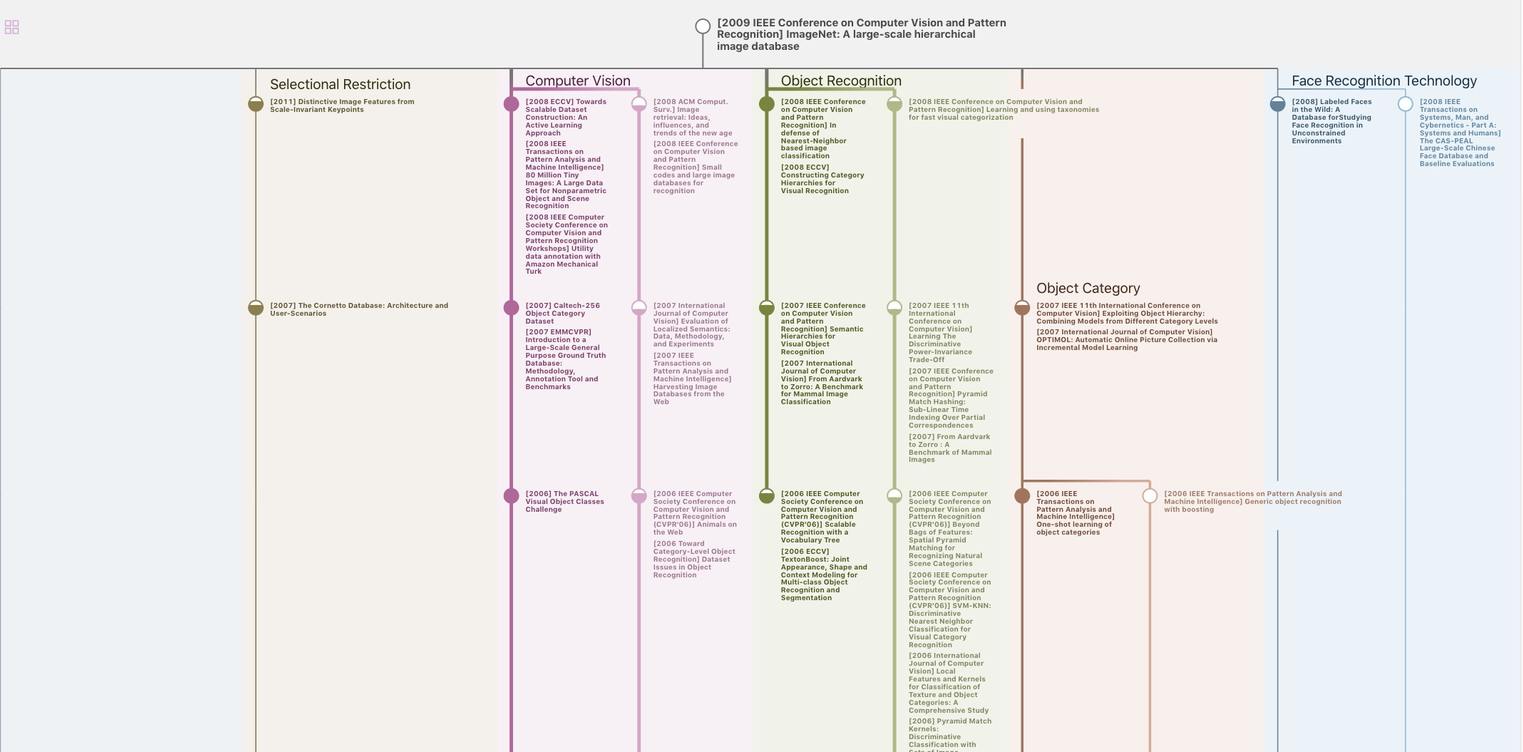
Generate MRT to find the research sequence of this paper
Chat Paper
Summary is being generated by the instructions you defined