The influence of virulence factors on dose response of food-borne pathogens
Journal of Food Processing and Technology(2016)
摘要
Considerable effort has gone into generating mathematical models for use in the assessment of risk of specific foodborne pathogens present in various foodstuffs. The usefulness of such models, especially to the food industry and regulators, is subject to generalizations made and assumptions used, by modellers. In particular, accuracy of these models is highly dependent on the human dose response data incorporated. For many pathogens, these data are sparse and (when available) are widely accepted as often inaccurate. The sweeping assumption made in all such models, that all strains of the same pathogen species are equally virulent and able to cause disease in humans, is incorrect. In this review, we speculate that some of the major bacterial foodborne pathogens (e.g., Salmonella, Campylobacter and Shiga toxin-producing Escherichia coli) substantially vary between strains, in their ability to (1) survive in and respond to the environment from the point of detection of the pathogen load in food, (2) colonize the human gut and (3) generate a disease outcome which will be observed as a dose response. There is increasing evidence for these speculations, and we propose that this variation could have a major impact on the dose response curves currently used for microbiological risk assessments. We discuss the importance of mechanisms that facilitate the survival of pathogens as they transit from farm-to-fork and then into the host. We also comment on the impact of genetic polymorphisms and how they impact the widely differing infectious doses observed with many pathogens. In conclusion, we suggest that mathematical models should be modified to incorporate more realistic dose response data, based on known knowledge of strain variation in environmental survival, colonization and clinical impact. Such modification would more accurately inform the food industry and regulators.
更多查看译文
AI 理解论文
溯源树
样例
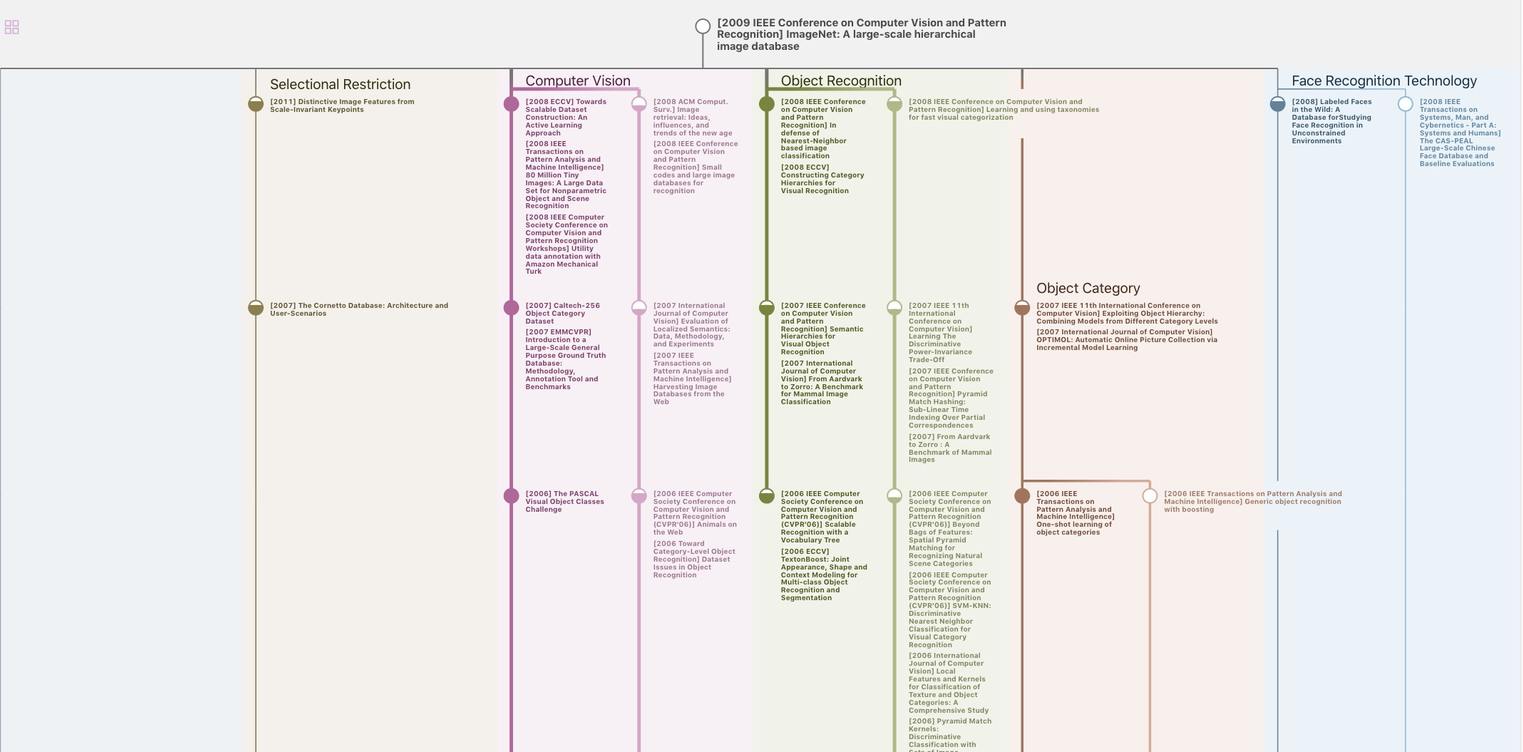
生成溯源树,研究论文发展脉络
Chat Paper
正在生成论文摘要