On penalized maximum likelihood estimation of approximate factor models
arXiv: Computation(2016)
摘要
In this paper, we mainly focus on the estimation of high-dimensional approximate factor model. We rewrite the estimation of error covariance matrix as a new form which shares similar properties as the penalized maximum likelihood covariance estimator given by Bien and Tibshirani(2011). Based on the lagrangian duality, we propose an APG algorithm to give a positive definite estimate of the error covariance matrix. The new algorithm for the estimation of approximate factor model has a desirable non-increasing property. By keeping the error covariance matrix to be positive definite, the efficiency of the new algorithm on estimation and forecasting is investigated via extensive simulations and real data analysis.
更多查看译文
AI 理解论文
溯源树
样例
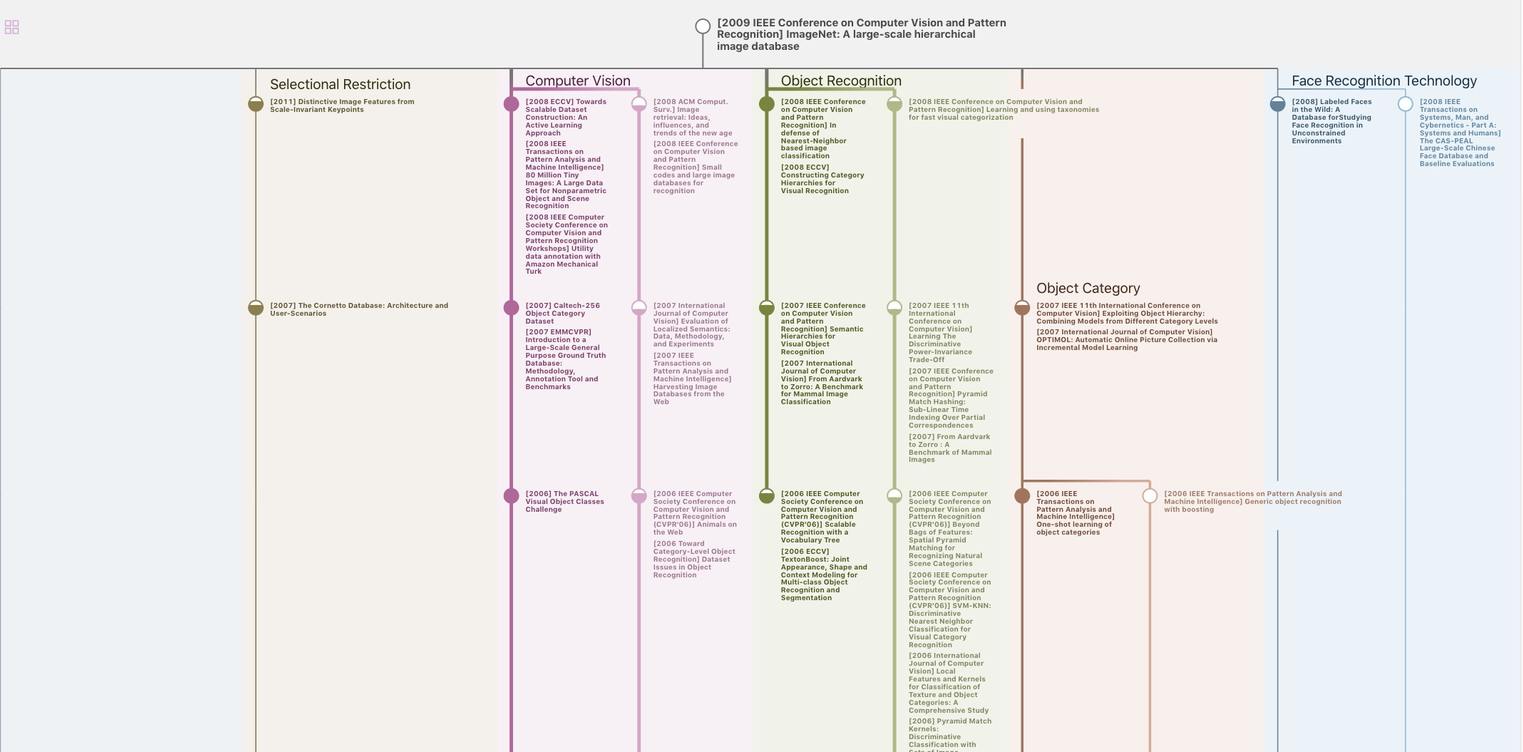
生成溯源树,研究论文发展脉络
Chat Paper
正在生成论文摘要