Data Analytics vs. Data Science: A Study of Similarities and Differences in Undergraduate Programs Based on Course Descriptions.
The Journal of information and systems in education(2015)
摘要
1. INTRODUCTION Inexpensive data storage and the ever-growing flow of data from a variety of sources increase the amount of data available to organizations. Competing in the era of big data will require analytically-focused employees with the specialized knowledge and skills to extract useful information from this data. Some have expressed great concern that the demand for employees with this skill set will far outstrip supply (see, e.g., Davenport and Patil, 2012). A widely cited report by McKinsey and Company concluded, United States alone faces a shortage of 140,000 to 190,000 people with deep analytical skills as well as 1.5 million managers and analysts to analyze big data and make decisions based on their findings (Manyika et al., 2011, p. 3). Universities are responding to this call to educate the next generation of entry level data savvy professionals. There are a growing number of degree programs, specializations, and certificates in data science and data analytics at both the graduate and undergraduate levels (Davenport and Patil, 2012; Dumbill et al., 2013). However, there are still relatively few full degree programs at the undergraduate level. A recent review of undergraduate degree programs in data analytics and data science identified thirteen such programs across the United States (Aasheim et al., 2014). Since that time, it is likely that more such programs have been developed. However, as more universities expand into this area, little is currently known about the specifics of skills covered in those degree programs or the extent to which skills coverage is comparable across different programs. This paper fills that gap by examining the course offerings of a small sample of undergraduate data analytics and data science programs to determine what similarities and differences exist across programs. In addition, discrepancies between skills in the literature and those offered in degree programs are identified. This examination will contribute to the goal of identifying important topics for an undergraduate program in data analytics and data science. The focus will be data analytics programs specifically and how they relate to the traditional information systems program. 2. LITERATURE REVIEW Organizations have collected and analyzed data in an attempt to gain strategic advantage in the market place for many years. However, in recent years, the amount and complexity of available data has exploded, making it more difficult to gain insights from data to improve business decision making. This section presents a review of relevant literature addressing (1) the growth of big data, (2) the evolution of data analytics as a field of study, (3) legal and ethical issues surrounding big data, and (4) implications for academia. 2.1 The Growth of Big Data A number of factors have contributed to the explosion of data. In the latter part of the 20th century, organizations emphasized integrating transactional databases into data warehouses that could then be analyzed to improve business decisions (Eckerson, 2011). As organizations began to realize benefits from this analysis, this trend accelerated. In one example, Walmart was able to identify bestselling products in hurricane-prone areas when storms were approaching; as a result, prior to storm season, Walmart stores stocked-up not only on obvious high-demand staples such as batteries but also the less obvious number two bestselling item--Pop-Tarts (Preimesberger, 2011). Growth in e-commerce and social media has contributed to the increase in data accumulation, particularly as organizations utilize clickstream data and social media comments to track customer sentiment and understand consumer behavior. Organizations also collect unstructured data through sources such as bar codes, QR codes, RFID tags, and sensors. United Parcel Service (UPS) installed sensors on more than 46,000 delivery trucks to monitor location, safety, and efficiency related data including speed, direction, and mechanical performance (Davenport, 2013). …
更多查看译文
AI 理解论文
溯源树
样例
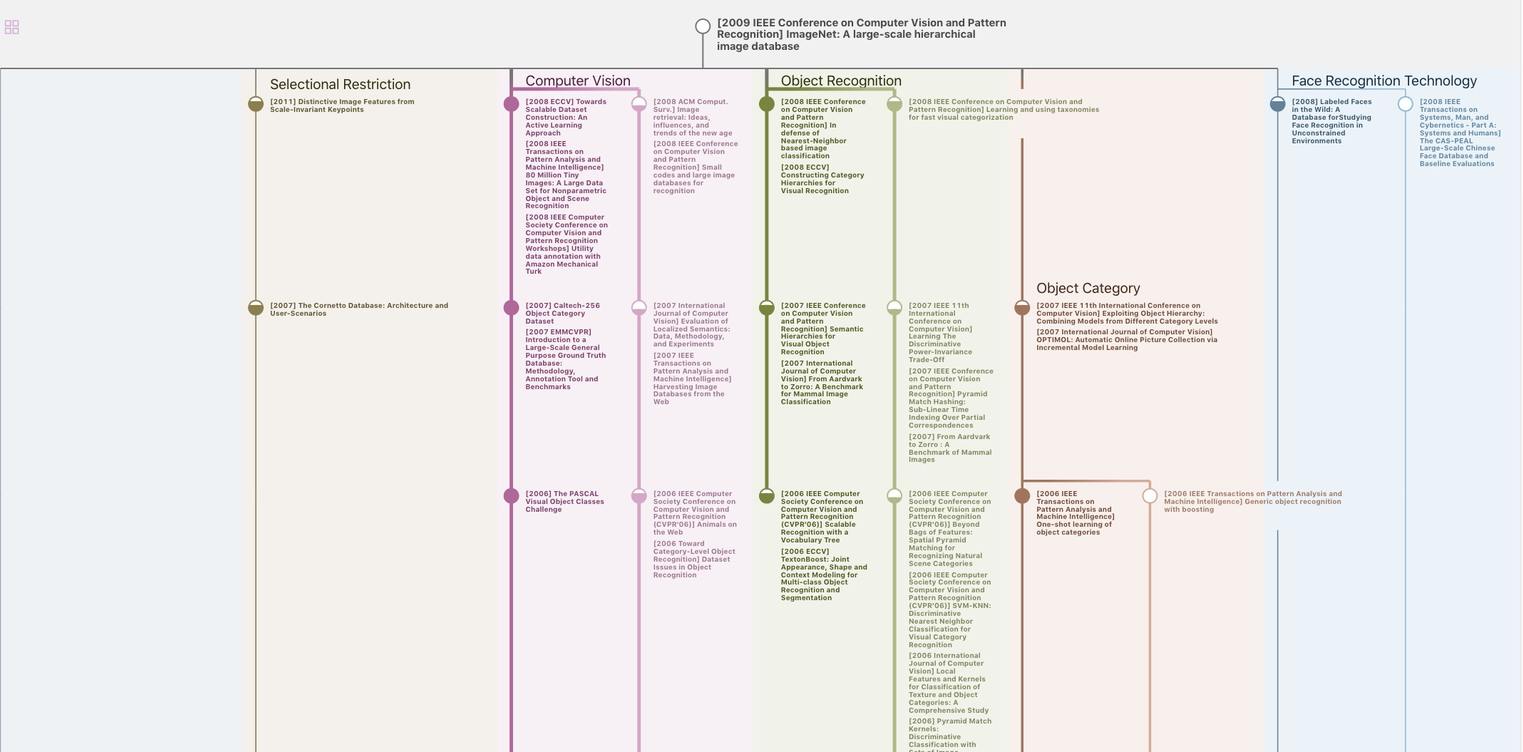
生成溯源树,研究论文发展脉络
Chat Paper
正在生成论文摘要