Radiogenomics Of Breast Cancer Using Dce-Mri And Gene Expression Profiling
CANCER RESEARCH(2016)
Abstract
Proceedings: AACR 107th Annual Meeting 2016; April 16-20, 2016; New Orleans, LAThe utilization of radiomics (high-throughput extraction and analysis of imaging features) to abstract underlying genomic features of an evolving malignancy is an emerging field that has the potential to detail biological information of a tumor in a non-invasive and more accessible manner. Furthermore, applying radiomics confers a comprehensive spatial view of the tumor, a potential advantage over the limitations of sampling a small region of tissue that may not accurately represent the underlying complexity of the entire tumor. Most studies to date that incorporate radiogenomics in the analysis of breast cancers have focused on few basic clinical data or individual genetic mutations such as BRCA or HER2 status.Here, we use an automated quantitative radiomics analysis platform developed at the University of Chicago that enables computerized feature extraction of tumors to analyze magnetic resonant imaging scans of 50 breast cancer patients (mean age of diagnosis [range]: 54 [24-89]; receptor status: HER2+: 14, Triple negative: 7; stage [1 through 4]: 10%, 40%, 42%, 8%) who have had comprehensive gene expression profiling performed using Agilent Human Gene Expression arrays. Our imaging platform extracts 38 features across six major phenotypes (size, shape, morphology, enhancement texture, kinetic curve assessment, and enhancement variance kinetics) (see Table for listing of 24 selected features). Existing radiomic analysis derived from a TCGA/TCIA dataset suggests that there are many correlations between imaging phenotypes and various genetic pathways, such as VEGF signaling and volume of enhancing voxels, base excision repair and enhancement texture entropy, and TGF-beta signaling and enhancement texture variance. We confirm these relationships as well as establish novel associations using a robust imaging dataset. By associating specific radiomic features with gene expression profile of tumors, we have the opportunity to extract detailed biological information non-invasively through clinical imaging. Phenotype category | Image phenotype | Description || ----------------------------- | ------------------------------------- | --------------------------------------------------------------------------------------------------------------- || Size | Volume | Volume of lesion || Size | Effective diameter | Diameter of a sphere with the same volume as the lesion || Size | Surface area | Lesion surface area || Shape | Sphericity | Similarity of the lesion shape to a sphere || Shape | Irregularity | Deviation of the lesion surface from the surface of a sphere || Shape | Surface area / volume | Ratio of surface area to volume || Morphology | Margin sharpness | Mean of the image gradient at the lesion margin || Morphology | Variance of margin sharpness | Variance of the image gradient at the lesion margin || Morphology | Variance of radial gradient histogram | Degree to which the enhancement structure extends in a radial pattern originating from the center of the lesion || Enhancement Texture | Contrast | Local image variations || Enhancement Texture | Entropy | Randomness of the gray-levels || Enhancement Texture | Difference variance | Variations of difference of gray-levels between voxel-pairs || Enhancement Texture | Angular second moment | Image homogeneity || Enhancement Texture | Maximum correlation coefficient | Nonlinear gray-level dependence || Enhancement Texture | Sum average | Overall brightness || Enhancement Texture | Sum of squares | Spread in the gray-level distribution || Kinetic Curve Assessment | Maximum enhancement | Maximum contrast enhancement || Kinetic Curve Assessment | Time to peak | Time at which the maximum enhancement occurs || Kinetic Curve Assessment | Uptake rate | Uptake speed of the contrast enhancement || Kinetic Curve Assessment | Curve shape index | Difference between late and early enhancement || Kinetic Curve Assessment | Total rate variation | How rapidly the contrast will enter and exit from the lesion || Enhancement-Variance Kinetics | Maximum variance of enhancement | Maximum spatial variance of contrast enhancement over time || Enhancement-Variance Kinetics | Time to peak maximum variance | Time at which the maximum variance occurs || Enhancement-Variance Kinetics | Enhancement variance increasing rate | Rate of increase of the enhancement-variance during uptake |Selected imaging phenotypes extracted from MRI scansCitation Format: Albert C. Yeh, Stephanie McGregor, Hui Li, Yuan Ji, Yitan Zhu, Tatyana Grushko, Alexandra Edwards, Fan Lui, Jing Zhang, Qiu Niu, Yonglan Zheng, Toshio Yoshimatsu, Galina Khramtsova, Karen Drukker, Gregory Karczmar, Hiroyuki Abe, Jeffrey Mueller, Maryellen Giger, Olufunmilayo Olopade. Radiogenomics of breast cancer using DCE-MRI and gene expression profiling. [abstract]. In: Proceedings of the 107th Annual Meeting of the American Association for Cancer Research; 2016 Apr 16-20; New Orleans, LA. Philadelphia (PA): AACR; Cancer Res 2016;76(14 Suppl):Abstract nr 2633.
MoreTranslated text
AI Read Science
Must-Reading Tree
Example
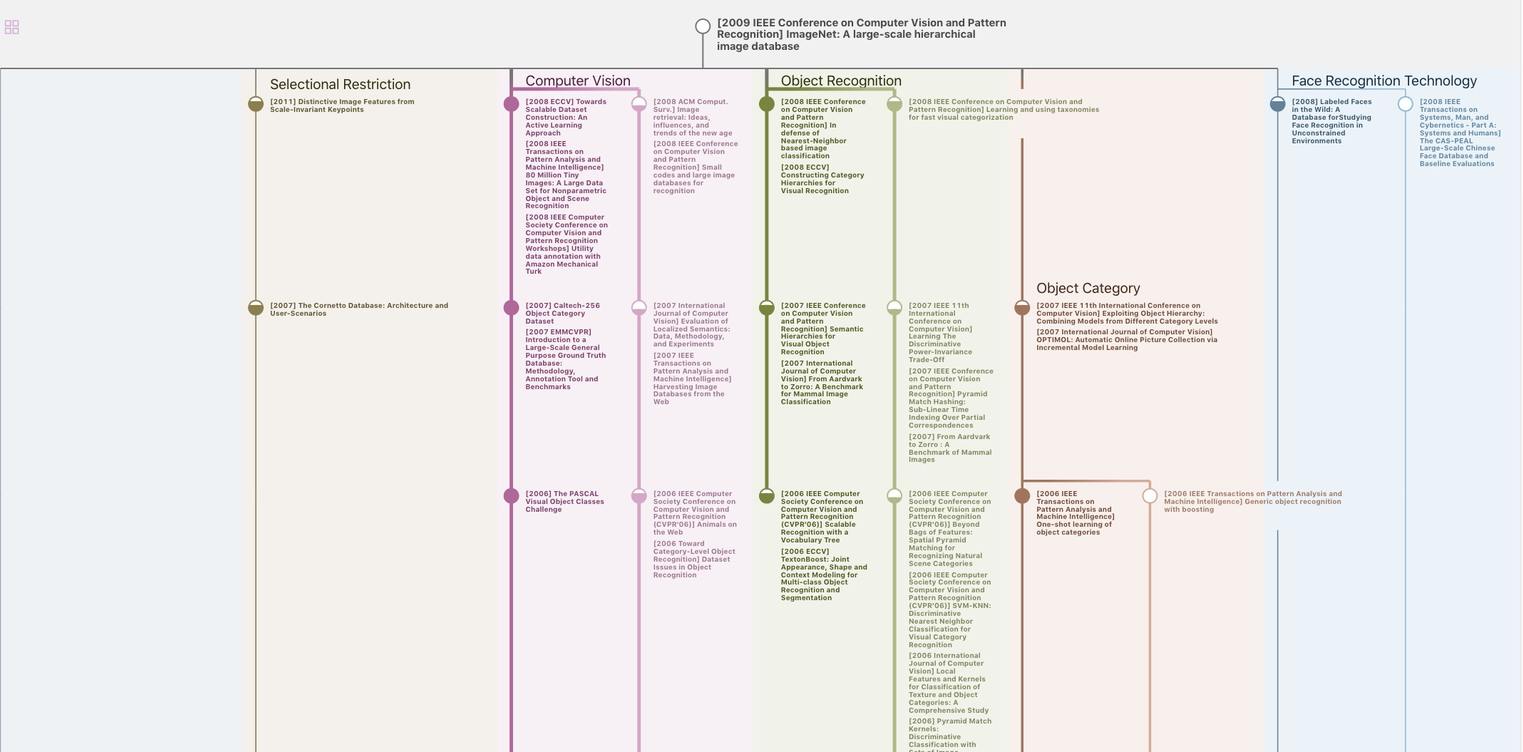
Generate MRT to find the research sequence of this paper
Chat Paper
Summary is being generated by the instructions you defined