Level-Screening Designs for factors with many levels
ANNALS OF APPLIED STATISTICS(2016)
摘要
We consider designs for f factors each at m levels, where f is small but m is large. Main effect designs with mf experimental points are presented. For two factors, two types of designs are investigated, termed sawtooth and dumbbell designs, based on a graphical representation. For three factors, cyclic sawtooth designs are considered. The paper seeks optimal and near optimal designs which involve factors with many levels but few observations. It also investigates issues of robustness when as much as one third of the data is structurally missing. An important area of application is in screening for drug discovery and we compare our designs with others using a published data set with two factors each with fifty levels, where the dumbbell design outperforms others and is an example of an inherently unbalanced design dominating more balanced designs.
更多查看译文
关键词
Screening designs,lead optimization in drug discovery,main effects,microarray loop designs,connectivity,identifiability,prediction and contrast variance
AI 理解论文
溯源树
样例
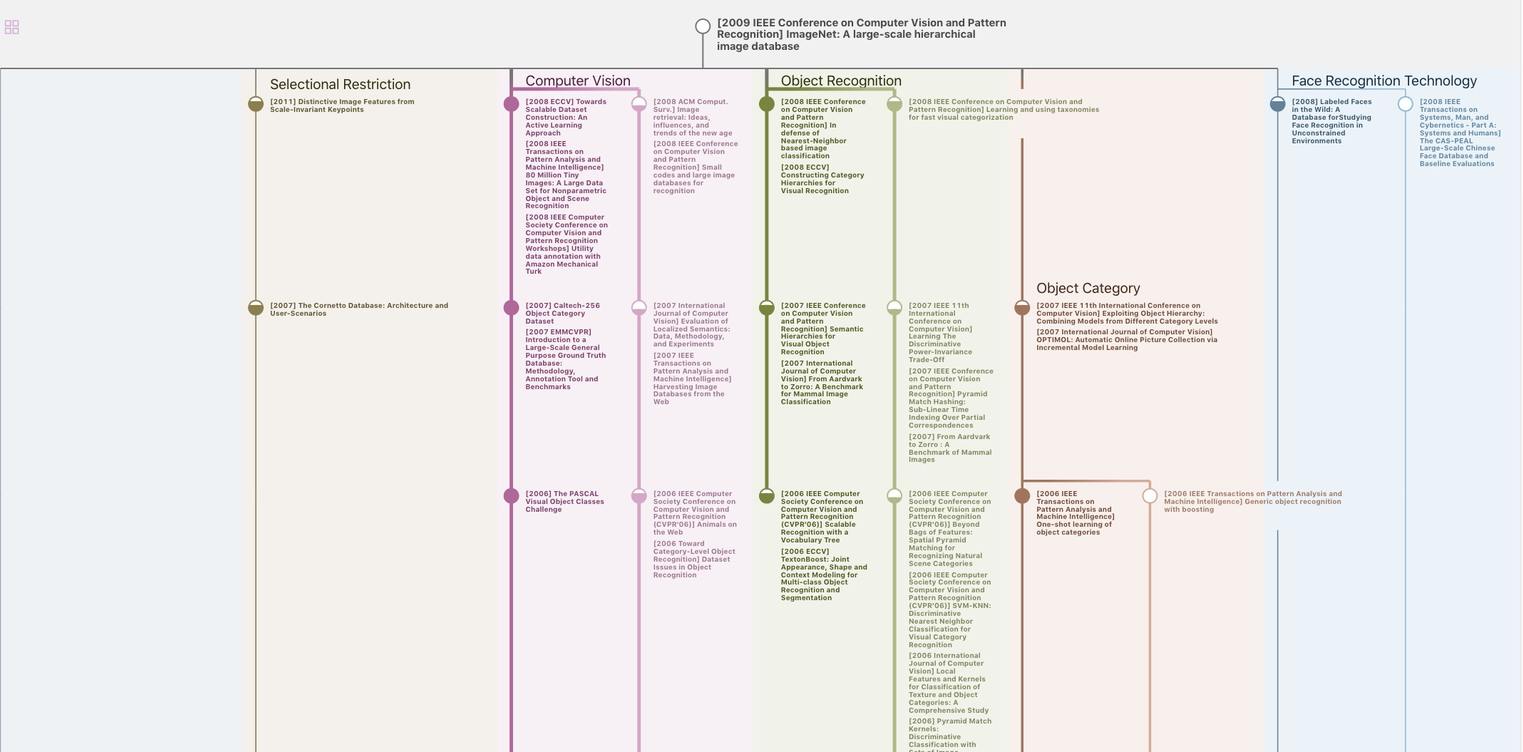
生成溯源树,研究论文发展脉络
Chat Paper
正在生成论文摘要