Engineering Design Exploration Using Locally Optimized Covariance Kriging
AIAA JOURNAL(2016)
摘要
Surrogate models are used in many engineering applications where actual function evaluations are computationally expensive. Kriging is a flexible surrogate model best suited for interpolating nonlinear system responses with a limited number of training points. It is commonly used to alleviate the high computational cost associated with design exploration techniques: for example, uncertainty quantification and multidisciplinary design optimization. However, when the underlying function shows varying degrees of nonlinear behavior within a design domain of interest, kriging, with a stationary covariance structure, can result in low-quality predictions and an overly conservative expected mean squared error. This effect is often amplified by data collected adaptively and unevenly during iterative design explorations. In this paper, the locally optimized covariance kriging method is proposed to capture the nonstationarity of the underlying function behavior. In locally optimized covariance kriging, the nonstationary behavior of an underlying function is identified with a statistical test process and approximated by aggregating a finite number of locally optimized stationary covariance structures. Compared to traditional stationary kriging, the proposed method provides an efficient and flexible computational framework capable of capturing transitional system behaviors and imposing physical understanding in building a surrogate model. Practical significance of the proposed method is discussed along with numerical examples.
更多查看译文
关键词
engineering,design,exploration
AI 理解论文
溯源树
样例
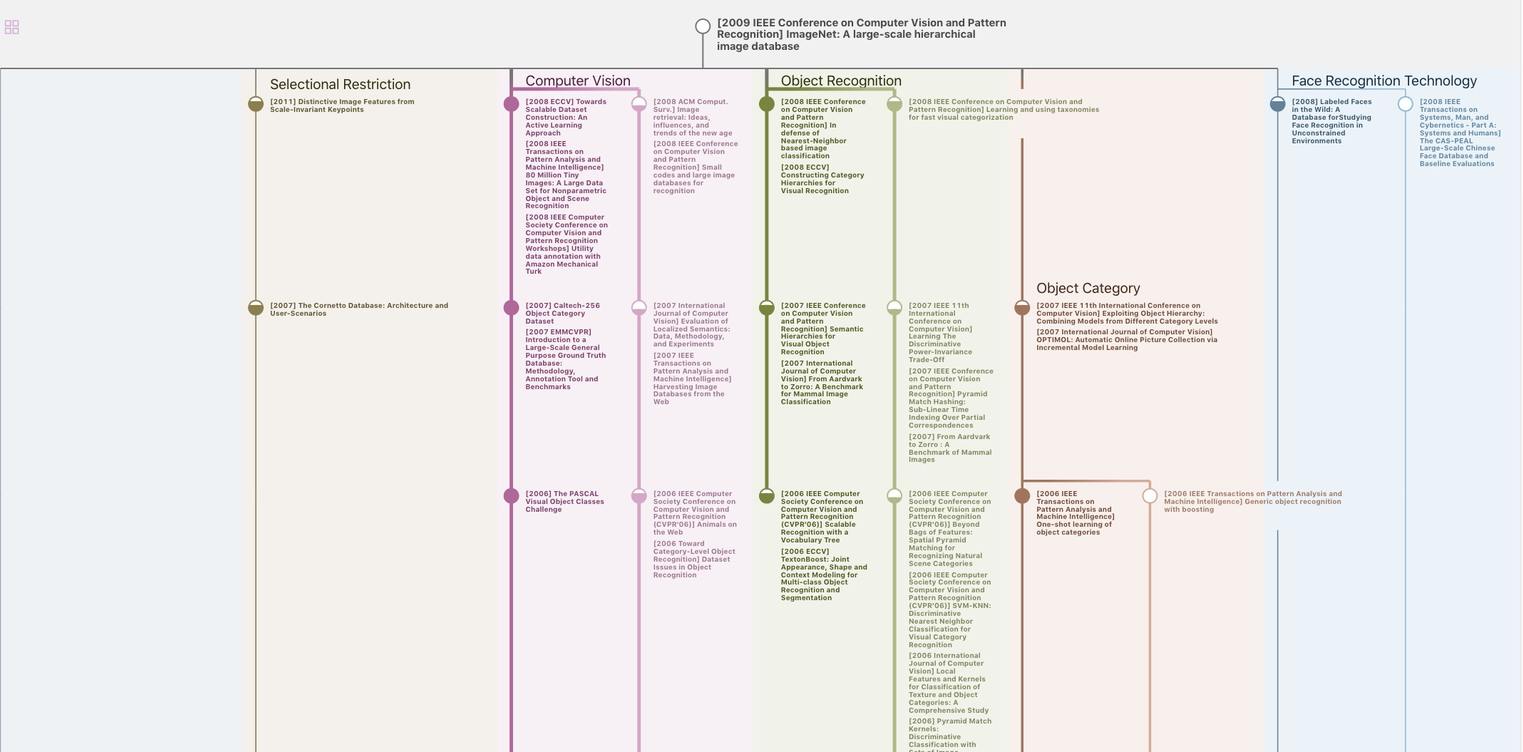
生成溯源树,研究论文发展脉络
Chat Paper
正在生成论文摘要