Reply to: "CD8 + T cell diversification by asymmetric cell division"
NATURE IMMUNOLOGY(2015)
摘要
The goal of our recent work1 was to trace the transcriptional ‘roadmap’ of individual CD8+ T lymphocytes as they differentiated in vivo from the naive state into one of three ‘fates’: terminally differentiated, short-lived effector (TSLE) cells (KLRG1hiIL-7Rlo), central memory (TCM) cells (CD44hiCD62Lhi) and effector memory (TEM) cells (CD44hiCD62Llo). We performed single-cell gene expression analyses on individual CD8+ T lymphocytes isolated at multiple time points (naive, cell division 1, day 3, day 5, day 7 TSLE, day 45 TEM, day 45 TCM) following microbial infection in vivo. This work suggested that an early divergence in lymphocyte fates may occur during an immune response to microbial infection.We disagree with the statement by Flossdorf et al. that we did not demonstrate how well the proposed early divergent model described the experimental data2. Single-cell gene expression measurements from cells representing all time points sampled were first incorporated into six linear and twelve bifurcative Hidden Markov Models (HMMs). We then tested how well each of these 18 possible models described the experimental data by calculating the cumulative distribution functions (CDF) of the log-likelihoods for each model. An alternative to calculating the CDF of the log-likelihoods for each model would have been to report the maximum log-likelihood of each model. However, the maximum log-likelihood value can be affected by the high degree of measurement noise that can occur in single-cell data. We therefore elected to use a ‘bootstrap’ technique commonly employed in modern statistics3 which resamples the dataset with replacement multiple times in order to assess the robustness of each model. Using this bootstrap technique, we calculated the CDFs of the log-likelihoods for each of the 18 models we tested, identifying an early divergent model as the one that best described the experimental data (Fig. 5b and Supplementary Fig. 4c in ref. 1). In this model, an activated CD8+ T lymphocyte gives rise to pre-TSLE or pre-memory cells; pre-TSLE cells subsequently undergo further differentiation to acquire the TSLE fate, whereas pre-memory cells further diverge to give rise to TCM or TEM cells.We further disagree with the contention by Flossdorf et al. that we did not demonstrate that the best model described the data significantly better than alternative models2. We compared the CDFs of the log-likelihoods for each of the 17 alternative models to the best model in a pairwise fashion. The significance of each of these pairwise comparisons was tested using the 2-sample Kolmogorov-Smirnov test, a commonly used, non-parametric, goodness-of-fit test. These analyses demonstrated that the best model described the data significantly better than alternative models (Supplementary Fig. 4c in ref. 1). Moreover, as a negative control, we showed that for all models tested, scrambled data performed no better than the bootstrap samples from actual data. While most of the alternative models had a higher log-likelihood on the experimental data than on the scrambled data, some did not. Notably, these alternative models, specifically sequential models that ordered memory and pre-memory states before the terminal effector and pre-effector states, were least consistent with the experimental data.Flossdorf et al. also argue that HMM is not a suitable analysis approach because it does not take into account potential differences in proliferative rates between pre-TSLE and pre-memory cells2. While the HMM does not explicitly model the different proliferative activities of the developing subsets, the training data we used to construct the HMM most certainly does. Different proliferative rates exhibited by pre-memory and pre-TSLE cell subsets would result in different frequencies of these cells within the day 3 and day 5 populations used for constructing the HMM. In other words, because pre-TSLE cells proliferate more, these cells would be found at higher frequencies within the day 3 and day 5 populations and therefore be more highly represented in the HMM. Thus, the HMM approach implicitly takes into account the different proliferative rates of the developing subsets.Flossdorf et al. suggest that an alternative computational analysis approach, Monocle4, is superior to our HMM because it does not assign cells to particular subsets and therefore makes inferences directly from their gene expression profiles. It should be clarified, however, that in our study, only cells at the beginning (naive T cells) or end (TSLE, TCM, or TEM cells) of the differentiation process were actually given ‘assignments.’ The unknown processes that the HMM was designed to reveal (i.e., the pathways by which a cell differentiates from the naive state to one of the three fates) were traced using gene expression data from cells with no labels other than the time after infection at which they were isolated (cell division 1, day 3, day 5, day 7). Thus, like Monocle, HMM can infer the path between the naive state to each one of the final cell fates on the basis of gene expression data alone without being biased by any cell labels or assignments.Flossdorf et al. re-analyzed our day 5 data using Monocle and failed to detect evidence of a bifurcation2. It is likely that Flossdorf et al. did not arrive at the same conclusion we did for several reasons. First, they used data only from a single time point, day 5 post-infection, rather than including data from all of the other intermediate time points that we used for our analysis. Second, selection of this particular time point may have been too late to discern the divergence in fate specification. In our study, both the principal component (Fig. 3 in ref. 1) and HMM (Fig. 5 in ref. 1) analyses suggested an early bifurcation in cell fate specification, which we confirmed experimentally in cells that had undergone their first division (Fig. 6 in reference 1). In a second analysis using Monocle, Flossdorf et al. analyzed data from naive, day 7 TSLE, day 45 TCM and day 45 TEM cells from our study, and failed to discern evidence of a bifurcation between memory (TCM and TEM) and terminal effector (TSLE) subsets2. However, the use of gene expression data solely from cells representing the ‘fully differentiated’ final fates, and not from cells representing the intermediate states, would seem to be inadequate to discern possible pathways of differentiation between the naive state and final fates.Lastly, Flossdorf et al. re-analyzed their published data5 and did not reach the same conclusions that we did in our study. Flossdorf et al. defined the starting state as naive and the final fates as day 8 CD27hi cells and day 8 CD27lo cells. They assayed protein expression of two markers, CD62L and CD27, by CD8+ T cells at a single time point, day 8 post-infection, and applied mathematical modeling to ascertain the differentiation pathways from a naive cell to day 8 CD27hi cells or day 8 CD27lo cells. Importantly, there were several differences in the aim and experimental design of the two studies that are likely to account for the discrepant conclusions obtained (summarized in Table 1). First, we defined the final fates as day 7 KLRG1hiIL-7Rαlo TSLE cells, day 45 CD44hiCD62Lhi TCM cells and day 45 CD44hiCD62Llo TEM cells. We then isolated cells from multiple intermediate time points without labeling them on the basis of surface markers and thus avoided making a priori assumptions about the identity of ‘pre-memory’ or ‘pre-effector’ T cells. Notably, in contrast to our study, no cells derived from intermediate states of differentiation were included in the analysis by Flossdorf et al (ref. 2). Moreover, we note that because our experimental data suggested that a divergence in cell fate occurs during the first cell division (Fig. 6 in ref. 1), evidence of a bifurcation may not have been detectable using only data obtained from cells isolated at a single late time point, day 8 post-infection, 7 days after the proposed bifurcation is thought to occur. Thus, important differences in the goals, assumptions and parameters analyzed are likely to account for the differences in the conclusions reached by each study.Table 1Comparison of aim and experimental design of studies by Arsenio et al. and Buchholz et al.In summary, the correspondence by Flossdorf et al. contends that our recently published work1 omitted key computational and functional validations of the model put forth by our experimental data; analysis of our dataset using an alternative computational approach yielded different conclusions; and re-analysis of their previously published dataset failed to recapitulate our findings. In this response, we demonstrate that these contentions lack merit, as the requested computational and functional validations were already included in the published paper; only a selected subset of our dataset was used for their analysis, making a direct comparison impossible; and substantial differences in the goals, designs and parameters analyzed by the two studies are likely to account for the discrepant conclusions drawn. Nonetheless, the points raised serve to highlight the utility and importance of single-cell approaches and make a compelling argument for their use in future studies of T lymphocyte fate specification.
更多查看译文
关键词
Immunology,Lymphocyte differentiation,Biomedicine,general,Infectious Diseases
AI 理解论文
溯源树
样例
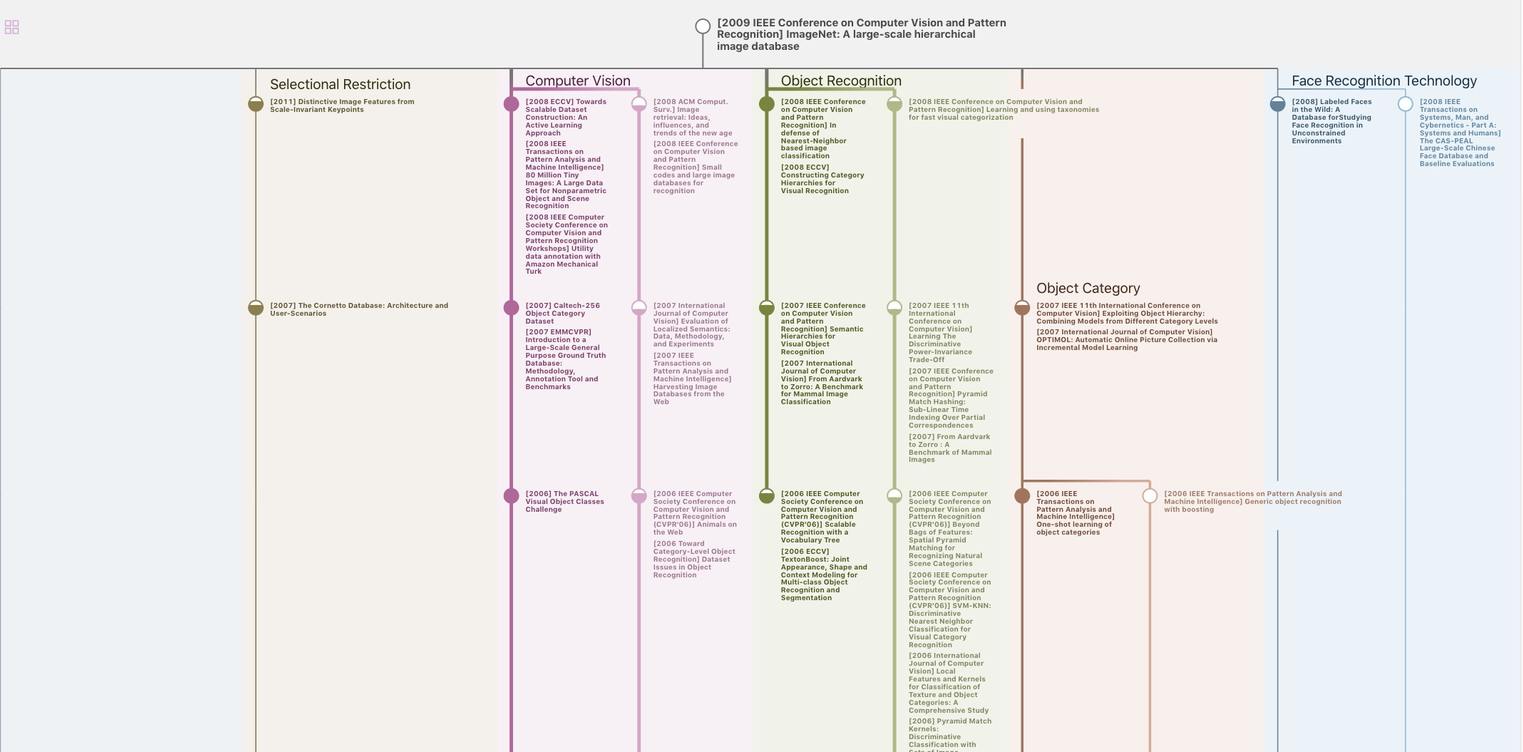
生成溯源树,研究论文发展脉络
Chat Paper
正在生成论文摘要