Demystifying Models: Answers to Ten Common Questions That Ecologists Have About Earth System Models
Limnology and Oceanography Bulletin(2016)
摘要
Every 2 years the University of Hawaii hosts the Ecological Dissertations in the Aquatic Sciences (EcoDAS) symposium, an ASLO and NSF-funded workshop designed to foster interdisciplinary collaborations among early career aquatic ecologists. During the EcoDAS symposium in 2014, many of the discussions revolved around a growing interest in using global climate models for making projections about how ecosystems and organisms will respond to future changes. However, many EcoDAS participants did not feel they had the required knowledge to employ climate models or participate in a climate modeling study. Following these discussions, we sent out a questionnaire to the past four cohorts of EcoDAS participants to further understand the general feeling of early career scientists toward climate models. Respondents were most interested in using climate models for determining effects of climate change on ecological processes relevant to specific organisms or habitats and the application of models to ecosystem management and assessment of anthropogenic impacts. However, about one third of respondents reported to have never worked with or were uncomfortable working with climate model output, and only about 10% reported having strong experience working with climate models. Similarly, a survey of marine resource managers indicated that 90% wanted to use outputs from climate change models, but > 40% faced hurdles related to lack of technical expertise, time, or personnel (Halpin et al. 2014). We concluded that a solution to the issues identified in these surveys could be to provide a brief, practical primer laying out the basics of climate modeling to increase proficiency in climate model use among aquatic ecologists. We hope this will in turn facilitate conversations between modelers and empiricists and serve as a stepping stone for collaboration among aquatic scientists. Here, we explain the basic functioning of climate models, provide advice on how to use climate models in relation to ecological research, outline data availability from models, discuss model limitations, and highlight common errors for beginners. We also suggest some understudied areas that could be a starting point for collaborations between modellers and empiricists. Projections of future climate change are made using two variants of climate models: General Circulation Models (GCMs) and Earth System Models (ESMs). Both types of models represent the global climate system through mechanistic, mathematical equations describing thermodynamics and fluid dynamics. These models divide Earth into a three-dimensional grid representing latitude, longitude and a vertical component (altitude of the atmosphere, ocean depth). At the start of a model run, each grid cell is assigned a value for each of the model's state variables (e.g., atmospheric temperature, ocean salinity). These initial conditions (Table 1) are based on global mean observations. For each time interval of the model simulation, values of the state variables (Table 1) change based on the mathematical equations, which describe either processes occurring within a grid cell (e.g., heating/cooling, evaporation/precipitation) and flows between cells. The model is first run over an initialization period of hundreds to thousands of years during which the climate system will come into a quasi-equilibrium state. This stage is referred to as “spin-up” (Table 1). Once this quasi-equilibrium is reached, greenhouse gases, volcanic emissions, aerosols, and land use may be varied reflecting historical trajectories or future scenarios. The difference between GCMs and ESMs is that the former generally represents physical processes occurring in the atmosphere, ocean, cryosphere, and interactions between these domains. In addition to representing oceanic and atmospheric dynamics, ESMs also include information on biogeochemical cycling in terrestrial and marine ecosystems and allow for these ecosystems to have feedbacks on the circulation. Therefore, all ESMs are GCMs, but not all GCMs are ESMs. By representing the full carbon cycle, including interactions between the atmosphere, ocean, land, and cryosphere, some ESMs do not need to prescribe a given concentration of atmospheric CO2 associated with emissions of greenhouse gases. Instead, CO2 can be dynamically partitioned between different reservoirs. Many ESMs also describe the fluxes of oxygen, nitrogen, phosphorus, silica, and iron throughout the earth system. To describe the cycling of these elements, most ESMs include functional groups of phytoplankton and zooplankton, as well as particulate and dissolved organic matter. Due to the inclusion of lower trophic level organisms in ESMs, these models are becoming increasingly useful for examining ecological questions. For example, ESMs have been used to investigate diverse ecological topics, such as how will climate change affect the seasonal timing of phytoplankton blooms (Henson et al. 2009, 2013; ), the species range of top marine predators (Hazen et al. 2013), and the boundaries of marine biomes (Polovina et al. 2011). Models provide experimental earth systems that can be used to test an imposed change and reasonably estimate the impacts of that change. Since the impacts of the imposed change are the outcome from numerical equations, models can be effective at elucidating the mechanistic drivers behind changes in oceanic dynamics and ecosystem structure and function. This differs from observational analyses, which rely mainly on correlative significance tests limited in space and time to support a hypothesized process that may lack a mechanistic explanation. Statistical relationships may also break down under novel future climate conditions. Modeling also differs from experimental analysis in that it is less limited in terms of the spatial and time scales of biological responses, and in its ability to manipulate multiple factors while maintaining adequate controls. Unlike most ocean observations, models provide “complete” data coverage, so that model output has no spatial or temporal gaps. The main disadvantage to using models is that they are dependent on the accuracy of the underlying model structure. While a model provides mechanistic drivers of the underlying processes, how representative these processes are of the natural system is limited by imperfect knowledge. This is why increased collaboration between modelers and empiricists is important. Other disadvantages stem from the spatial resolution and the number of ecological processes resolved by models. Data from all IPCC-class models are available online for free through the Coupled Model Intercomparison Project – Phase 5 (CMIP5). Figure 1 provides an overview of how to download and use CMIP5 data. To begin working with data from CMIP5, you will need access to software for visualizing and analyzing your data. MATLAB, R, Python, and IDL are commonly used programs for working with data from climate models. However, all of these require basic scientific computing skills. Ocean Data View (ODV) and ArcGIS are two additional programs that can be used, which have the advantage of not requiring users to have any previous programming knowledge. Working with outputs from ESMs is similar to working with data from satellites or any other type of gridded dataset with information on geographic locations and multiple time periods. The learning curve for people who previously have used such datasets and software programs should not be excessively steep when transitioning to working with data outputted from ESMs. Schematic illustrating the process of downloading, reading, and pre-processing data files from IPCC-class models. One potentially confusing aspect of working with ESMs is the proliferation of acronyms describing models. While it is beyond the scope of this paper to provide a comprehensive list to acronyms, we recommend the following resources for further detail: (1) Standardized abbreviations for climate variables used by CMIP5 are described in http://cmip-pcmdi.llnl.gov/cmip5/docs/standard_output.pdf (last accessed: March 6, 2016); (2) The 2013 IPCC report defines acronyms pertaining to IPCC climate models in http://www.ipcc.ch/pdf/assessment-report/ar5/wg1/WG1AR5_AnnexIV_FINAL.pdf (last accessed: March 6, 2016). There is no single best model for all applications. The strengths of each model will vary depending on the variable of interest, region, temporal or spatial scale, and your scientific question. Since models are built in different ways and have contrasting strengths and weaknesses, it is a good practice to compare outputs from several models. The latest IPCC report used a compilation of over 50 GCMs referred to as CMIP5. Among those, only 16 are ESMs that have an ocean biogeochemistry component that include nutrients (Si, Fe, N, P) and one or more phytoplankton and zooplankton groups. Since different models perform better in different regions, using many models (also known as an ensemble) often improves accuracy on a global basis. Using multiple models also helps to assess uncertainty in projections due to differences in model structure. The ensemble mean often most closely resembles observations, but it is also important to consider the range of variability between models. Also, the dynamics of an ensemble may not be internally consistent, since it represents a combination of different models. Currently, there is no agreed upon way of weighting different models in an ensemble. At the regional scale, weighting can be problematic because a model can correctly reproduce small, regional features for the wrong reasons. If this occurs, reproducing such features may be unrelated to the ability to make robust future projections (Stock et al. 2011). The 2013 IPCC report uses a “one model, one vote” approach for calculating ensemble means (Flato et al. 2013). When calculating ensemble means and other statistics, remember that each model is not fully independent, since many models were constructed using similar code and mathematical equations. Despite advantages of using ensembles, it may be more practical to consider a single model in cases where one wants to compare a large number of projections from different scenarios. This can be useful for conservation or management studies to show the effects of different mitigation strategies. ESMs are designed to simulate the earth system at the global scale and reproduce broad regions of physical and biogeochemical variability. ESMs have a coarse spatial resolution (~ 1° × 1°), although exact resolution varies between models. The atmospheric and oceanic components of a single model may have different resolutions or the resolution of the model grid may vary with latitude (i.e., finer resolution in the tropics to resolve equatorial currents). Typically ESMs cannot resolve mesoscale processes (e.g., eddies, fronts). Until recently, eddy-resolving models were prohibitively computationally expensive for anything beyond regional, multi-year simulations. Some next generation ESMs, currently under development, will have a 0.1° to 0.25° resolution that can begin to resolve eddies (Griffies et al. 2015). Overall, smaller scale coastal processes that are of particular interest to aquatic ecologists may not be resolvable using current ESMs. Furthermore, river inputs in ESMs typically impact the freshwater balance only and do not yet modify ocean biogeochemistry. Thus, for the moment, a regional model will be better at capturing nearshore processes than an ESM. However, current research efforts are attempting to provide a global model of river/watershed export of nutrients (http://marine.rutgers.edu/globalnews/datasets.htm; last accessed: March 6, 2016). Researchers interested in processes operating at small spatial scales can explore dynamical and statistical downscaling, which are reviewed by Harris et al. (2014). Dynamical downscaling uses a global ESM to force the boundary conditions of a regional climate model with finer resolution. In statistical downscaling, empirical relationships between variables are used to increase spatial resolution in cases where fine-scale data are available for some variables. Numerical models only include processes closely aligned to the primary goal of model development, which is making climate projections at a global scale. Models also only include processes that can be described with a mechanistic formulation or parameterized. Many empirical studies conclude with an argument that a process needs to be included in future models to improve accuracy (e.g., Davison et al. 2013; Holding et al. 2013, 2015; ). While there are several important ecological and biogeochemical processes that remain unresolved by ESMs, there is a risk of over-parsing or over-tuning a model by incorporating a process that, although critical for a particular region or variable, may offset other model processes that were already effectively simulated. Adding new variables can increase computing time, while not improving overall model performance. Consequently, there is a clear trade-off when including new processes. Furthermore, there is a lag time between the discovery of an important process and its incorporation into a model because models are developed in distinct phases. In conclusion, collaborations between modelers and empiricists are critical for furthering knowledge of how ecosystems will respond to global climate change. For scientists new to ESMs, we recommend developing collaborations with climate modelers in order to have a source of support for resolving queries and to get help understanding what features of a model are “real” vs. features that may arise due to idiosyncrasies of model design. Table 2 lists several references that can be consulted for further help with ESMs. Overall, we hope that this paper eases the burden of getting started with using ESMs, as well as spurs a greater dialog between modelers and experimental and field ecologists. We acknowledge the support of NSF (grant no. OCE-1356192) and ASLO for funding our participation in the 2014 EcoDAS XI symposium. We also thank the organizers and host of EcoDAS XI, Center for Microbial Oceanography: Research and Education (C-MORE) at the University of Hawaii at Manoa, for making this collaborative work possible. A. Gnanadesikan and J.L. Sarmiento reviewed earlier drafts of this manuscript and provided helpful feedback. Rebecca G. Asch, Program in Atmospheric and Oceanic Sciences, Princeton University, Princeton, New Jersey Darren J. Pilcher, Pacific Marine Environmental Laboratory, National Oceanic and Atmospheric Administration, Seattle, Washington Sara Rivero-Calle, Department of Earth and Planetary Sciences, Johns Hopkins University, Baltimore, Maryland Johnna M. Holding, Institut Mediterrani d'Estudis Avançats, Esporles-Mallorca-Illes Balears
更多查看译文
关键词
Ecosystem Functioning,Ecosystem Management
AI 理解论文
溯源树
样例
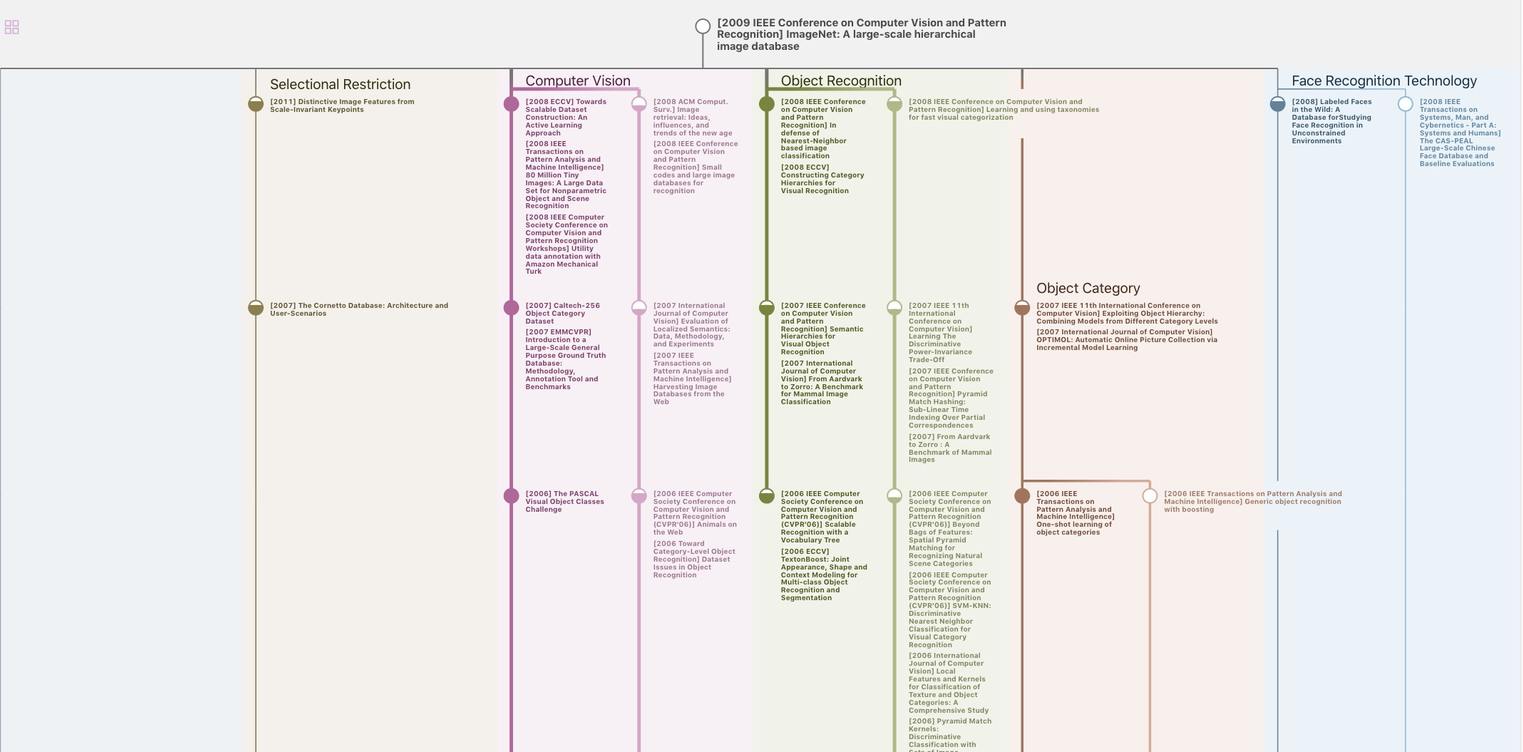
生成溯源树,研究论文发展脉络
Chat Paper
正在生成论文摘要