Centralized Multi-Sensor Structured Branch Multiple Hypothesis Algorithm
Computer Simulation(2015)
摘要
This paper studies the parallel multi-sensor multi-target tracking in dense clutter. A structured branch multiple hypothesis tracking algorithm based on multi-level data compression is proposed to solve the problem of large association computation,low corrected paring rate between measurements and tracks with traditional data association algorithm performed under the condition of dense clutter and high false alarm rate. The algorithm is designed to determine the optimal pairing and expand the existed single-stage data compression to two-stage. Then redundant branches generated in traditional MHT algorithm are processed with this method for compression and pruning. After that,Optimal Equivalent branch is remained in track family tree and optimized output state estimate is given. Simulation result shows that this approach performs good tracking accuracy and real-time ability,reduces the computation time and provides an effective way for multi-target tracking in dense clutter.
更多查看译文
关键词
algorithm,multi-sensor
AI 理解论文
溯源树
样例
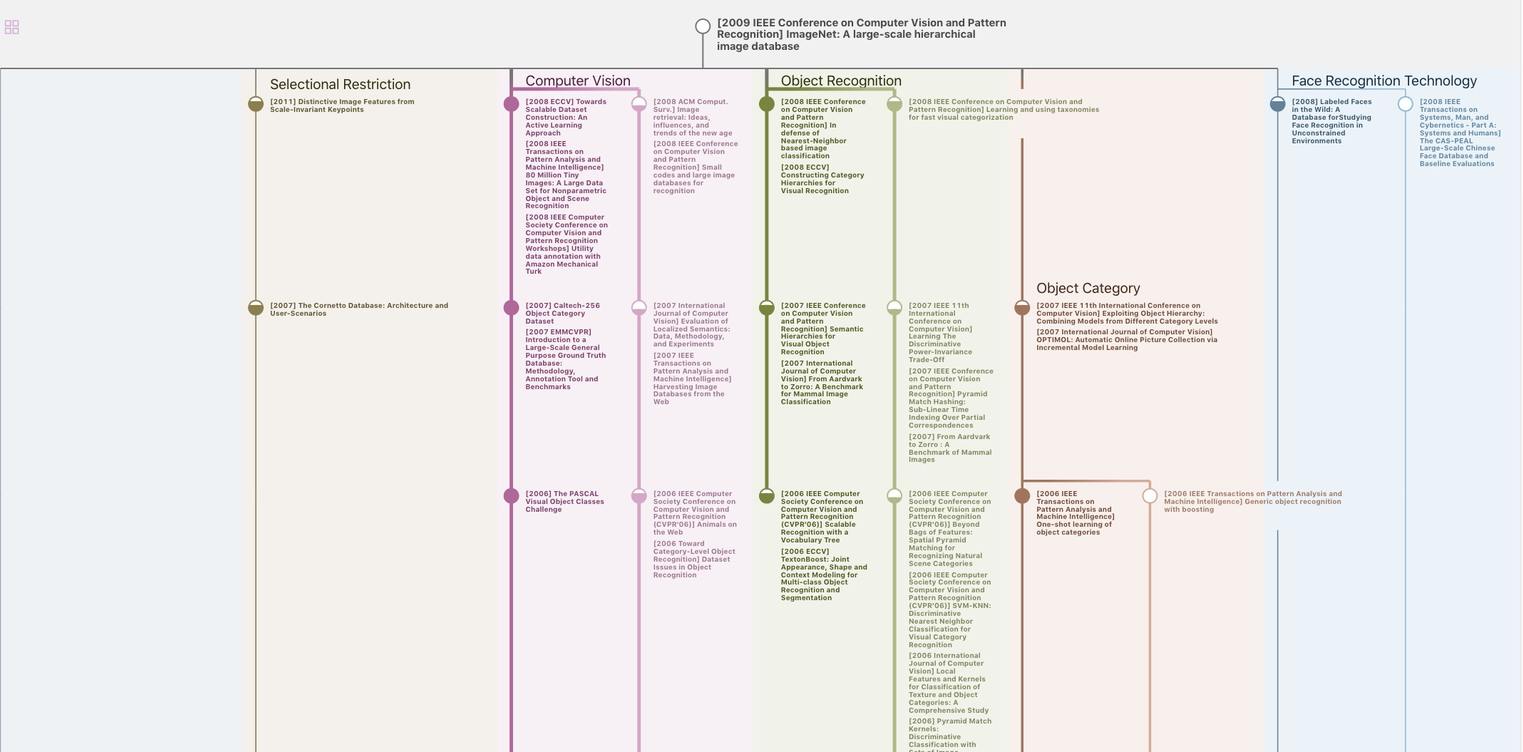
生成溯源树,研究论文发展脉络
Chat Paper
正在生成论文摘要