Personalized Crime Location Prediction
EUROPEAN JOURNAL OF APPLIED MATHEMATICS(2016)
摘要
Crime reduction and prevention strategies are vital for policymakers and law enforcement to face inevitable increases in urban crime rates as a side effect of the projected growth of urban population by the year 2030. Studies conclude that crime does not occur uniformly across urban landscapes but concentrates in certain areas. This phenomenon has drawn attention to spatial crime analysis, primarily focusing on crime hotspots, areas with disproportionally higher crime density. In this paper, we present CRIMETRACER(1), a personalized random walk-based approach to spatial crime analysis and crime location prediction outside of hotspots. We propose a probabilistic model of spatial behaviour of known offenders within their activity spaces. Crime Pattern Theory concludes that offenders, rather than venture into unknown territory, frequently select targets in or near places they are most familiar with as part of their activity space. Our experiments on a large real-world crime dataset show that CRIMETRACER outperforms all other methods used for location recommendation we evaluate here.
更多查看译文
关键词
Spatial crime analysis,Random walk model,Activity space,Crime occurrence space,Co-offending networks
AI 理解论文
溯源树
样例
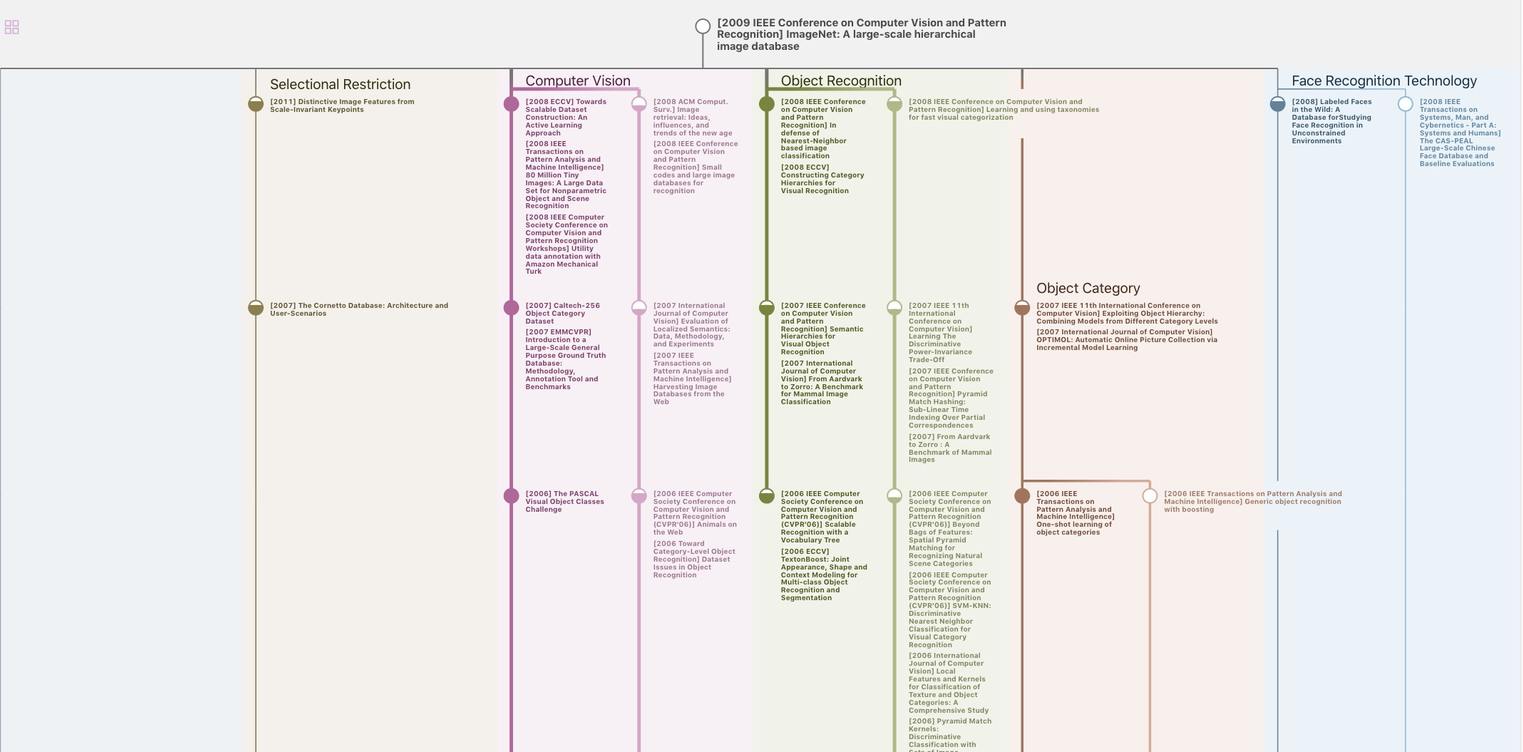
生成溯源树,研究论文发展脉络
Chat Paper
正在生成论文摘要